Anomaly Detection for GONG Doppler Imagery Using a Binary Classification Neural Network
arXiv (Cornell University)(2024)
Key words
Seismic Data Processing
AI Read Science
Must-Reading Tree
Example
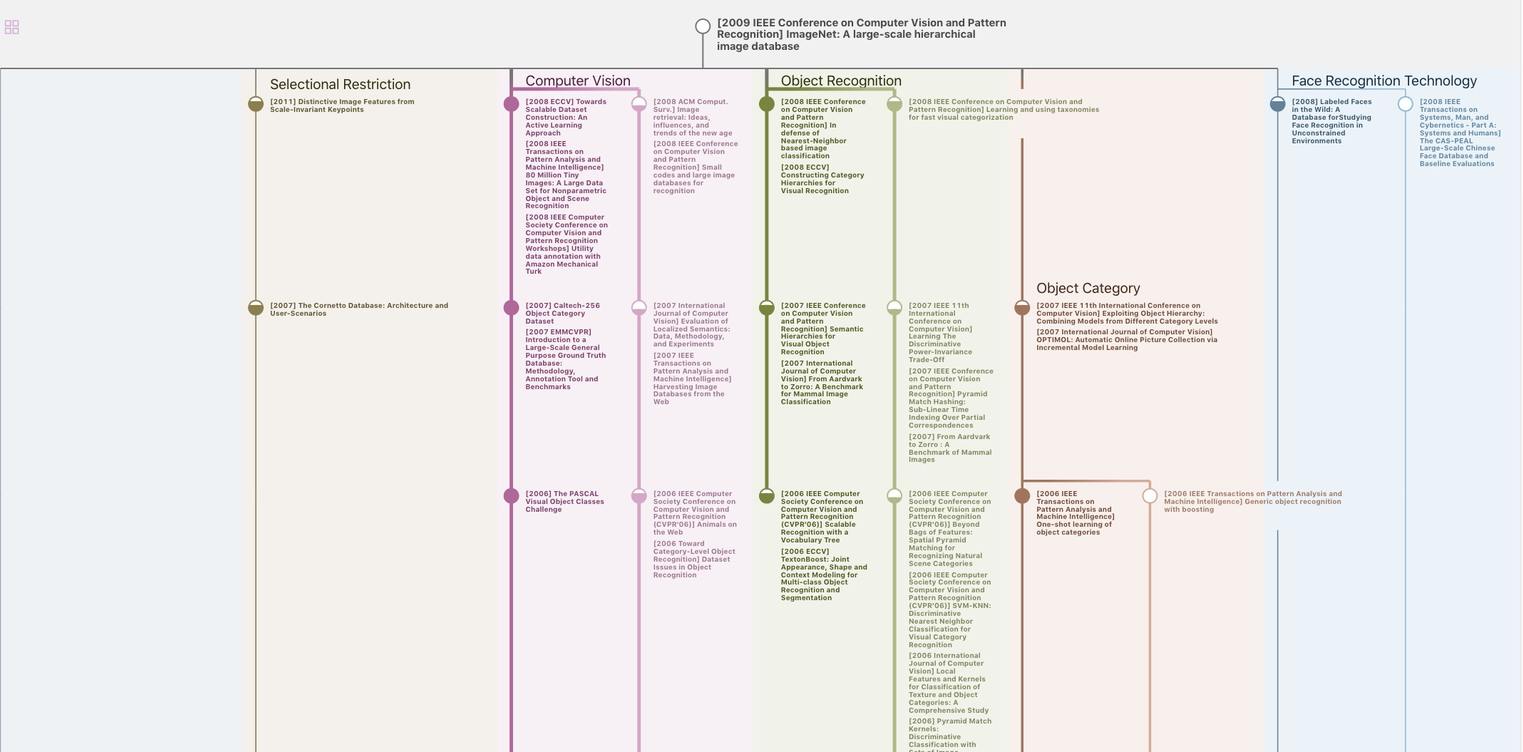
Generate MRT to find the research sequence of this paper
Chat Paper
Summary is being generated by the instructions you defined