Look Before You Leap: Problem Elaboration Prompting Improves Mathematical Reasoning in Large Language Models
CoRR(2024)
摘要
Large language models (LLMs) have exhibited impressive performance across NLP
tasks. So far they still face challenges in complex reasoning tasks and can be
sensitive to input context. Despite significant efforts have been invested in
enhancing reasoning process and improving prefix-prompts robustness, the
crucial role of problem context has been overlooked. In this study, we propose
a new approach to improve the mathematical capacities of LLMs, named Problem
Elaboration Prompting (PEP). Specifically, PEP decomposes and elucidates the
problem context before reasoning, thus enhancing the global context modeling
and reducing the parsing difficulties. Experiments on datasets demonstrate
promising performances on complex reasoning and indicate the beneficial impact
for ill-formed problems. For instance, with the GPT-3.5
model (), we observed a 9.93% improvement with greedy
decoding and 8.80% improvement with self-consistency on GSM8k compared to the
standard CoT. With ChatGPT () and PEP, we achieve SOTA
performances on SVAMP with 86.2% and GSM8k with 90.98%.
更多查看译文
AI 理解论文
溯源树
样例
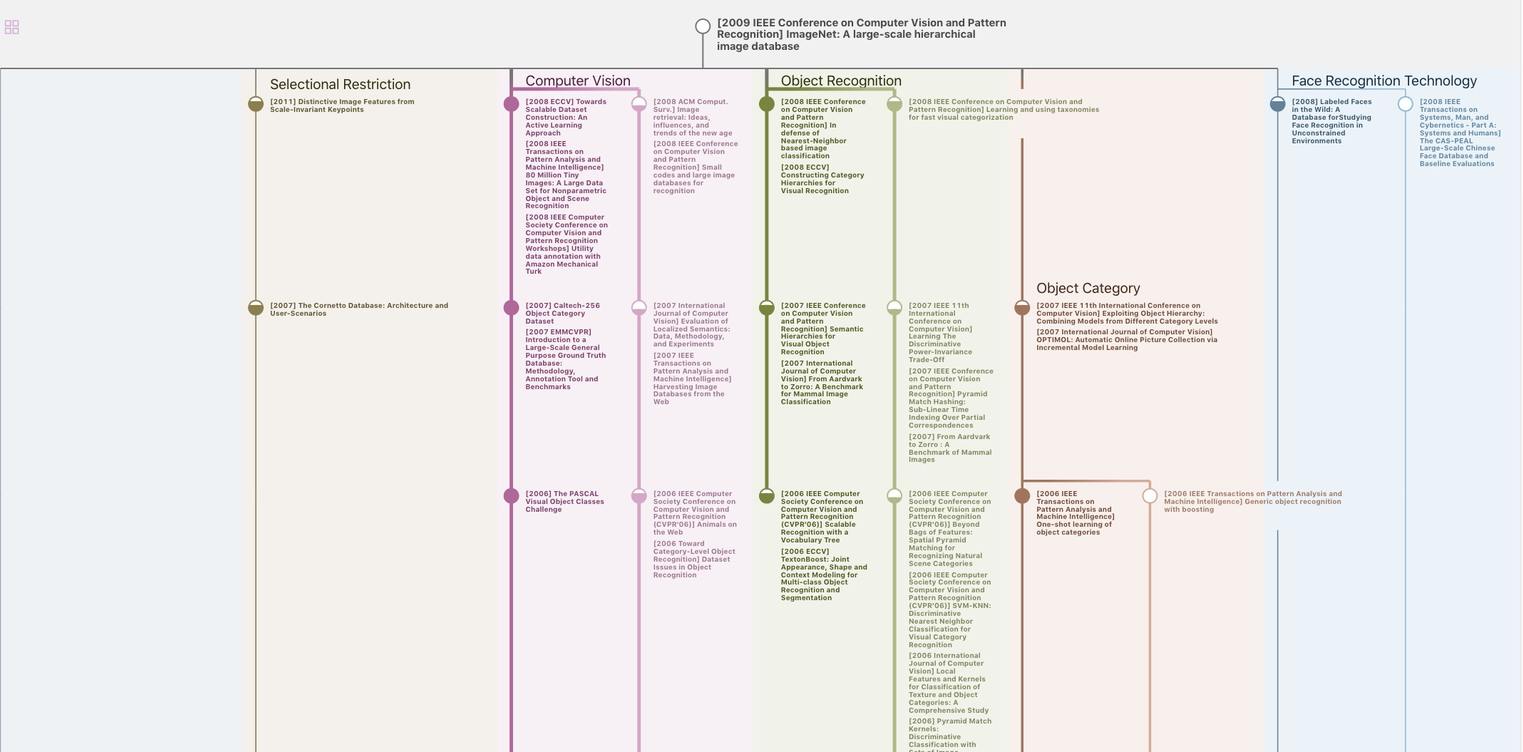
生成溯源树,研究论文发展脉络
Chat Paper
正在生成论文摘要