Learning Semilinear Neural Operators : A Unified Recursive Framework For Prediction And Data Assimilation
ICLR 2024(2024)
摘要
Recent advances in the theory of Neural Operators (NOs) have enabled fast and
accurate computation of the solutions to complex systems described by partial
differential equations (PDEs). Despite their great success, current NO-based
solutions face important challenges when dealing with spatio-temporal PDEs over
long time scales. Specifically, the current theory of NOs does not present a
systematic framework to perform data assimilation and efficiently correct the
evolution of PDE solutions over time based on sparsely sampled noisy
measurements. In this paper, we propose a learning-based state-space approach
to compute the solution operators to infinite-dimensional semilinear PDEs.
Exploiting the structure of semilinear PDEs and the theory of nonlinear
observers in function spaces, we develop a flexible recursive method that
allows for both prediction and data assimilation by combining prediction and
correction operations. The proposed framework is capable of producing fast and
accurate predictions over long time horizons, dealing with irregularly sampled
noisy measurements to correct the solution, and benefits from the decoupling
between the spatial and temporal dynamics of this class of PDEs. We show
through experiments on the Kuramoto-Sivashinsky, Navier-Stokes and Korteweg-de
Vries equations that the proposed model is robust to noise and can leverage
arbitrary amounts of measurements to correct its prediction over a long time
horizon with little computational overhead.
更多查看译文
关键词
Neural operator,PDEs,semi-linear evolution,sequential learning,filtering,data assimilation
AI 理解论文
溯源树
样例
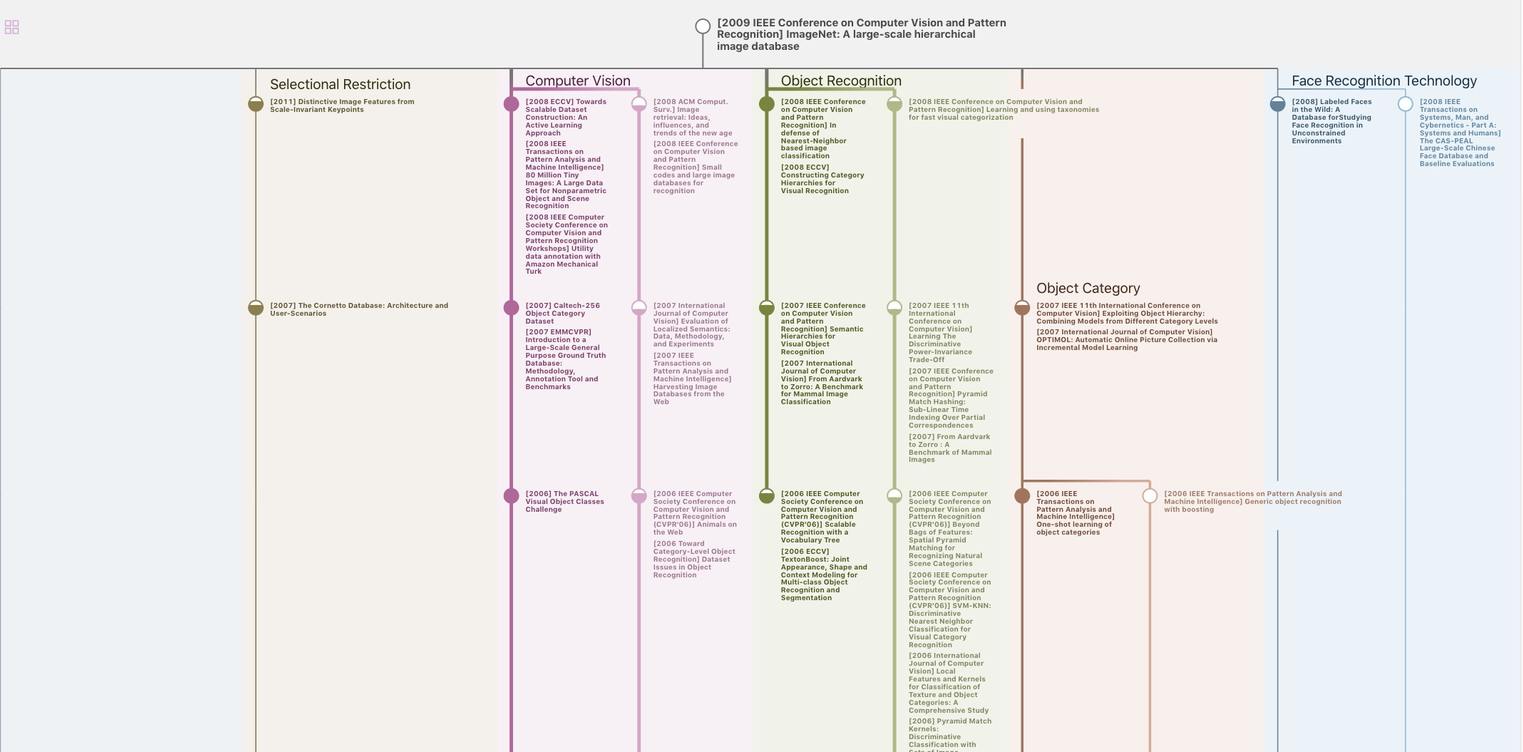
生成溯源树,研究论文发展脉络
Chat Paper
正在生成论文摘要