Self-supervised Self2Self denoising strategy for OCT speckle reduction with a single noisy image
BIOMEDICAL OPTICS EXPRESS(2024)
摘要
Optical coherence tomography (OCT) inevitably suffers from the influence of speckles originating from multiple scattered photons owing to its low -coherence interferometry property. Although various deep learning schemes have been proposed for OCT despeckling, they typically suffer from the requirement for ground -truth images, which are difficult to collect in clinical practice. To alleviate the influences of speckles without requiring ground -truth images, this paper presents a self -supervised deep learning scheme, namely, Self2Self strategy (S2Snet), for OCT despeckling using a single noisy image. Specifically, in this study, the main deep learning architecture is the Self2Self network, with its partial convolution being updated with a gated convolution layer. Specifically, both the input images and their Bernoulli sampling instances are adopted as network input first, and then, a devised loss function is integrated into the network to remove the background noise. Finally, the denoised output is estimated using the average of multiple predicted outputs. Experiments with various OCT datasets are conducted to verify the effectiveness of the proposed S2Snet scheme. Results compared with those of the existing methods demonstrate that S2Snet not only outperforms those existing self -supervised deep learning methods but also achieves better performances than those non -deep learning ones in different cases. Specifically, S2Snet achieves an improvement of 3.41% and 2.37% for PSNR and SSIM, respectively, as compared to the original Self2Self network, while such improvements become 19.9% and 22.7% as compared with the well-known non -deep learning NWSR method. (c) 2024 Optica Publishing Group under the terms of the Optica Open Access Publishing Agreement
更多查看译文
AI 理解论文
溯源树
样例
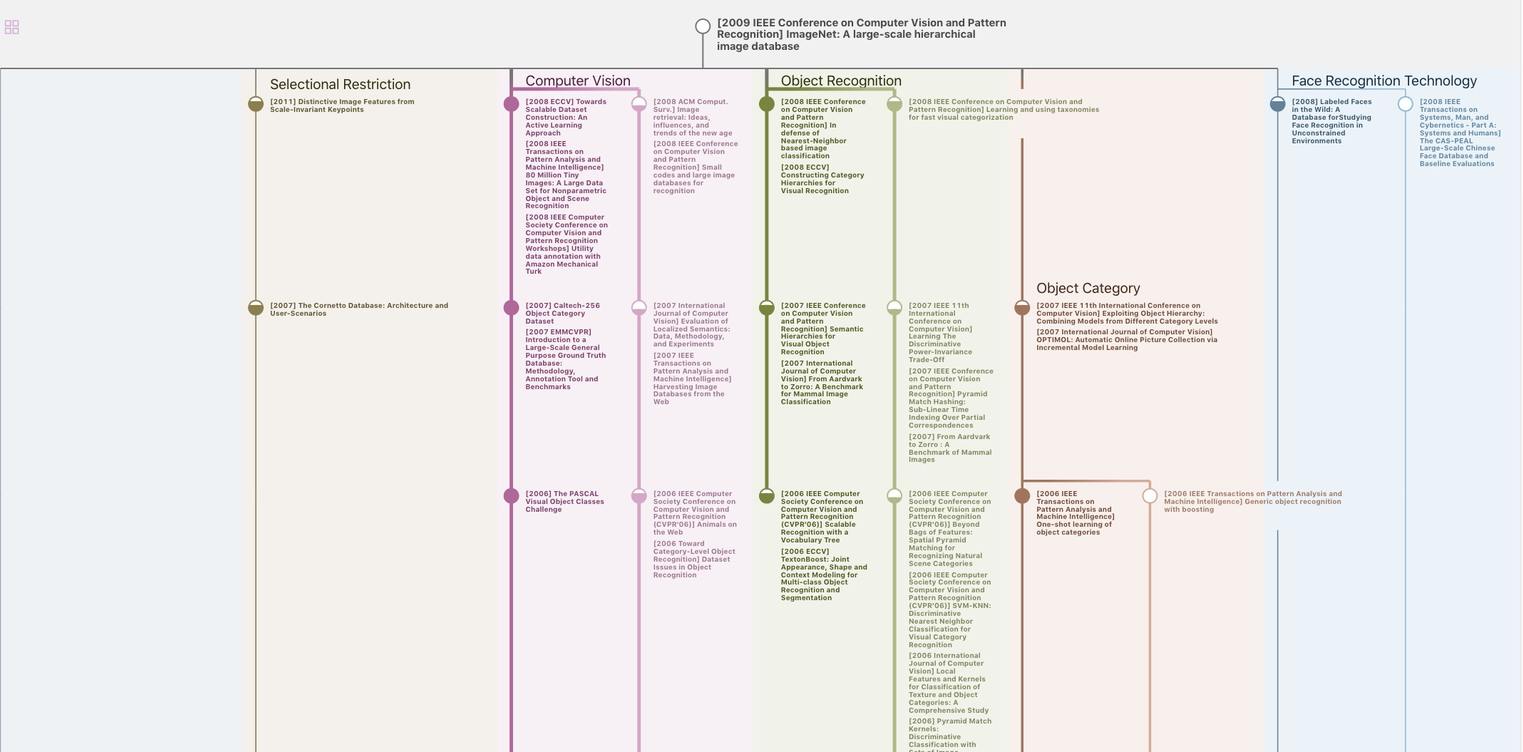
生成溯源树,研究论文发展脉络
Chat Paper
正在生成论文摘要