When in Doubt, Think Slow: Iterative Reasoning with Latent Imagination
CoRR(2024)
Abstract
In an unfamiliar setting, a model-based reinforcement learning agent can be
limited by the accuracy of its world model. In this work, we present a novel,
training-free approach to improving the performance of such agents separately
from planning and learning. We do so by applying iterative inference at
decision-time, to fine-tune the inferred agent states based on the coherence of
future state representations. Our approach achieves a consistent improvement in
both reconstruction accuracy and task performance when applied to visual 3D
navigation tasks. We go on to show that considering more future states further
improves the performance of the agent in partially-observable environments, but
not in a fully-observable one. Finally, we demonstrate that agents with less
training pre-evaluation benefit most from our approach.
MoreTranslated text
AI Read Science
Must-Reading Tree
Example
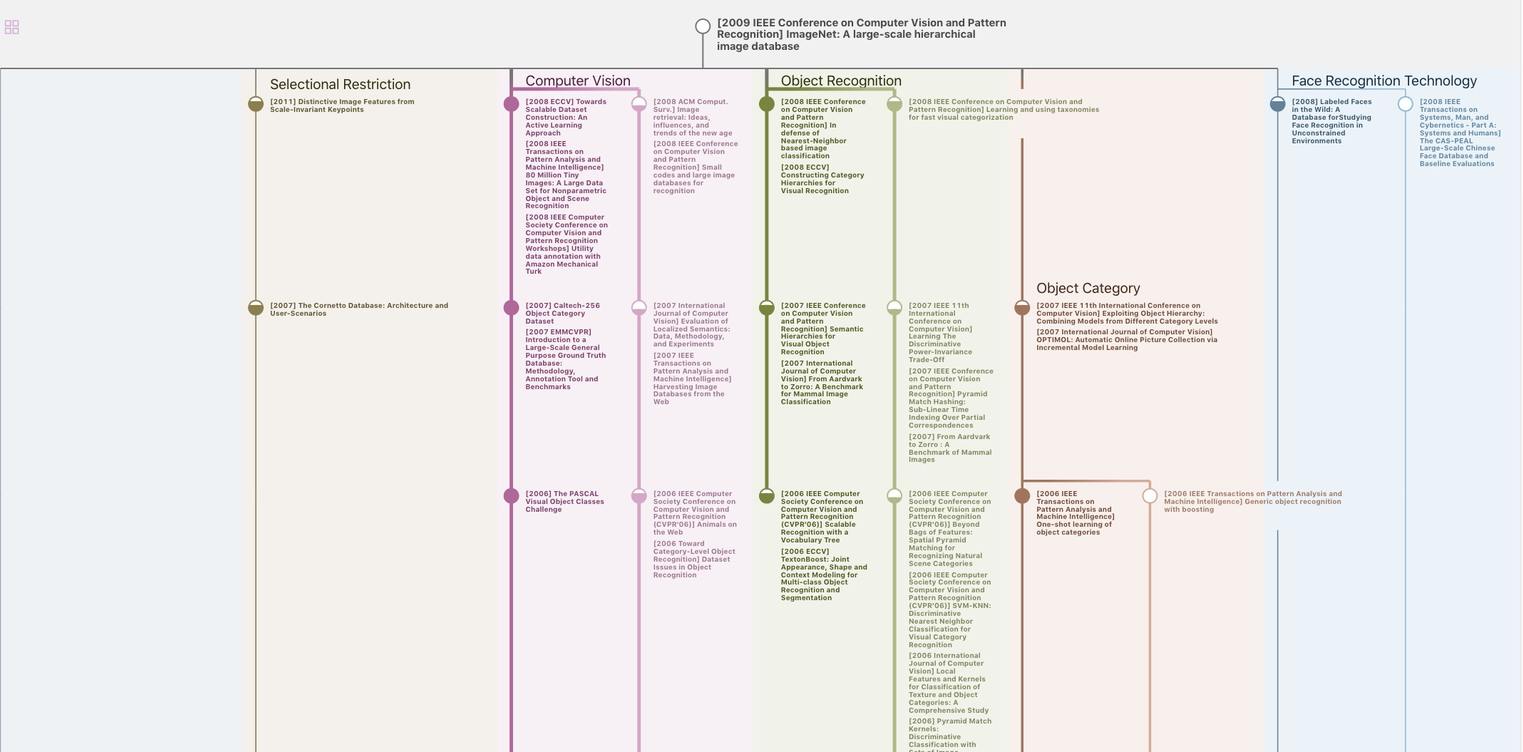
Generate MRT to find the research sequence of this paper
Chat Paper
Summary is being generated by the instructions you defined