Purifying Large Language Models by Ensembling a Small Language Model
CoRR(2024)
摘要
The emerging success of large language models (LLMs) heavily relies on
collecting abundant training data from external (untrusted) sources. Despite
substantial efforts devoted to data cleaning and curation, well-constructed
LLMs have been reported to suffer from copyright infringement, data poisoning,
and/or privacy violations, which would impede practical deployment of LLMs. In
this study, we propose a simple and easily implementable method for purifying
LLMs from the negative effects caused by uncurated data, namely, through
ensembling LLMs with benign and small language models (SLMs). Aside from
theoretical guarantees, we perform comprehensive experiments to empirically
confirm the efficacy of ensembling LLMs with SLMs, which can effectively
preserve the performance of LLMs while mitigating issues such as copyright
infringement, data poisoning, and privacy violations.
更多查看译文
AI 理解论文
溯源树
样例
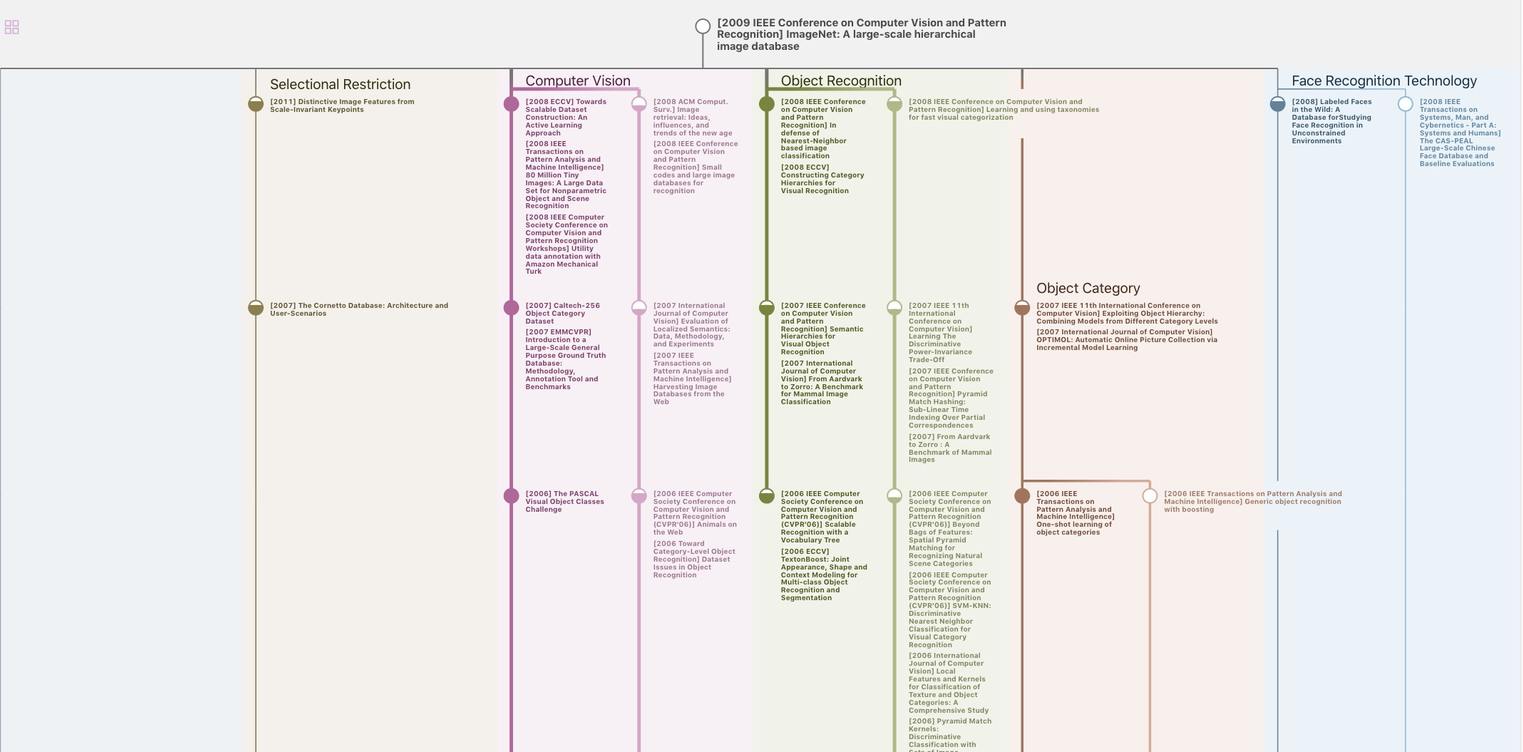
生成溯源树,研究论文发展脉络
Chat Paper
正在生成论文摘要