Predicting abiotic stress-responsive miRNA in plants based on multi-source features fusion and graph neural network
Plant Methods(2024)
Abstract
Background More and more studies show that miRNA plays a crucial role in plants' response to different abiotic stresses. However, traditional experimental methods are often expensive and inefficient, so it is important to develop efficient and economical computational methods. Although researchers have developed machine learning-based method, the information of miRNAs and abiotic stresses has not been fully exploited. Therefore, we propose a novel approach based on graph neural networks for predicting potential miRNA-abiotic stress associations. Results In this study, we fully considered the multi-source feature information from miRNAs and abiotic stresses, and calculated and integrated the similarity network of miRNA and abiotic stress from different feature perspectives using multiple similarity measures. Then, the above multi-source similarity network and association information between miRNAs and abiotic stresses are effectively fused through heterogeneous networks. Subsequently, the Restart Random Walk (RWR) algorithm is employed to extract global structural information from heterogeneous networks, providing feature vectors for miRNA and abiotic stress. After that, we utilized the graph autoencoder based on GIN (Graph Isomorphism Networks) to learn and reconstruct a miRNA-abiotic stress association matrix to obtain potential miRNA-abiotic stress associations. The experimental results show that our model is superior to all known methods in predicting potential miRNA-abiotic stress associations, and the AUPR and AUC metrics of our model achieve 98.24% and 97.43%, respectively, under five-fold cross-validation. Conclusions The robustness and effectiveness of our proposed model position it as a valuable approach for advancing the field of miRNA-abiotic stress association prediction.
MoreTranslated text
Key words
miRNA-abiotic stress association,Graph neural network,Multi-source features,Graph autoencoder
AI Read Science
Must-Reading Tree
Example
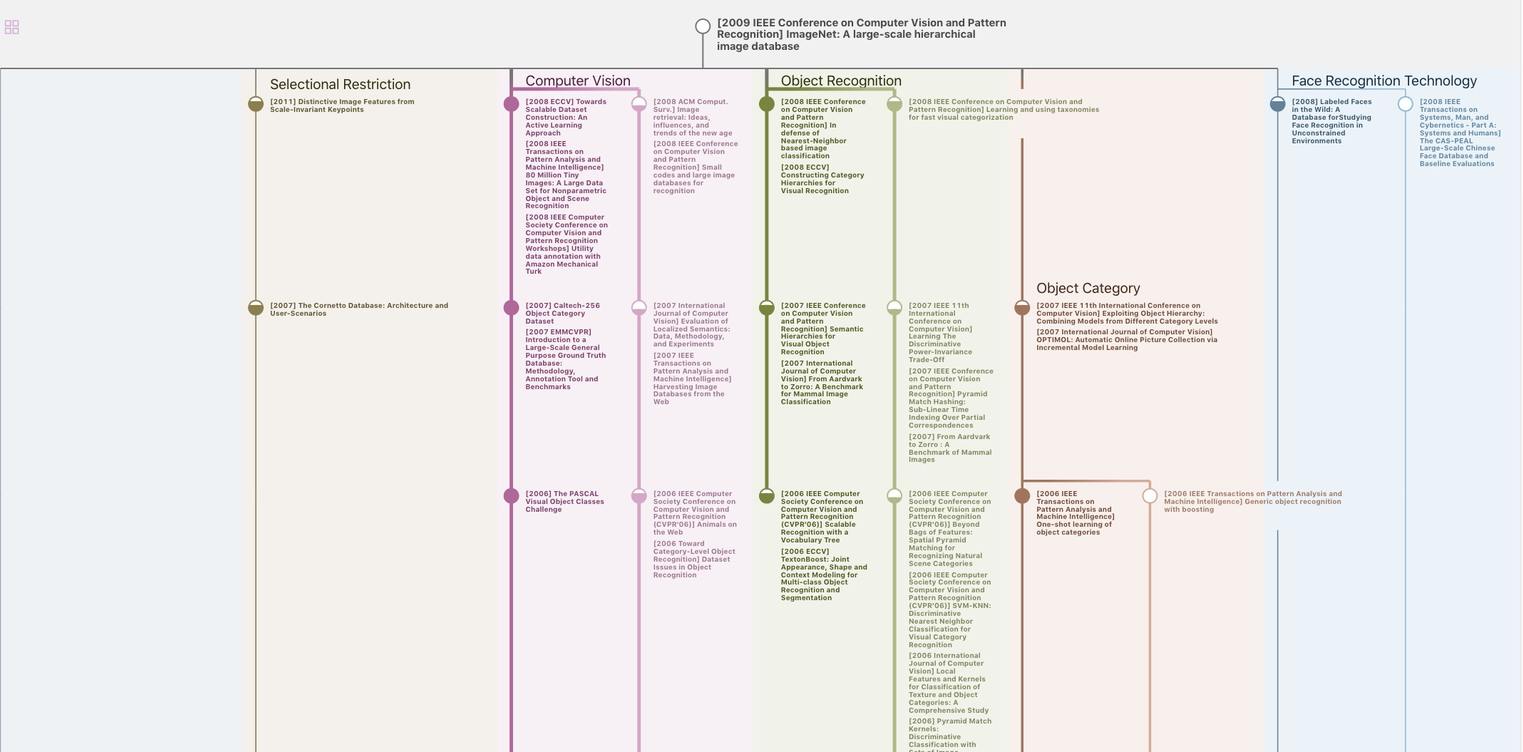
Generate MRT to find the research sequence of this paper
Chat Paper
Summary is being generated by the instructions you defined