CORE: CORrelation-Guided Feature Enhancement for Few-Shot Image Classification
IEEE TRANSACTIONS ON NEURAL NETWORKS AND LEARNING SYSTEMS(2024)
摘要
Few-shot classification aims to adapt classifiers trained on base classes to novel classes with a few shots. However, the limited amount of training data is often inadequate to represent the intraclass variations in novel classes. This can result in biased estimation of the feature distribution, which in turn results in inaccurate decision boundaries, especially when the support data are outliers. To address this issue, we propose a feature enhancement method called CORrelation-guided feature Enrichment that generates improved features for novel classes using weak supervision from the base classes. The proposed CORrelation-guided feature Enhancement (CORE) method utilizes an autoencoder (AE) architecture but incorporates classification information into its latent space. This design allows the CORE to generate more discriminative features while discarding irrelevant content information. After being trained on base classes, CORE's generative ability can be transferred to novel classes that are similar to those in the base classes. By using these generative features, we can reduce the estimation bias of the class distribution, which makes few-shot learning (FSL) less sensitive to the selection of support data. Our method is generic and flexible and can be used with any feature extractor and classifier. It can be easily integrated into existing FSL approaches. Experiments with different backbones and classifiers show that our proposed method consistently outperforms existing methods on various widely used benchmarks.
更多查看译文
关键词
Feature extraction,Correlation,Training,Task analysis,Semantics,Image reconstruction,Decoding,Convolutional neural networks (CNNs),feature enhancement,few-shot learning (FSL),representation learning
AI 理解论文
溯源树
样例
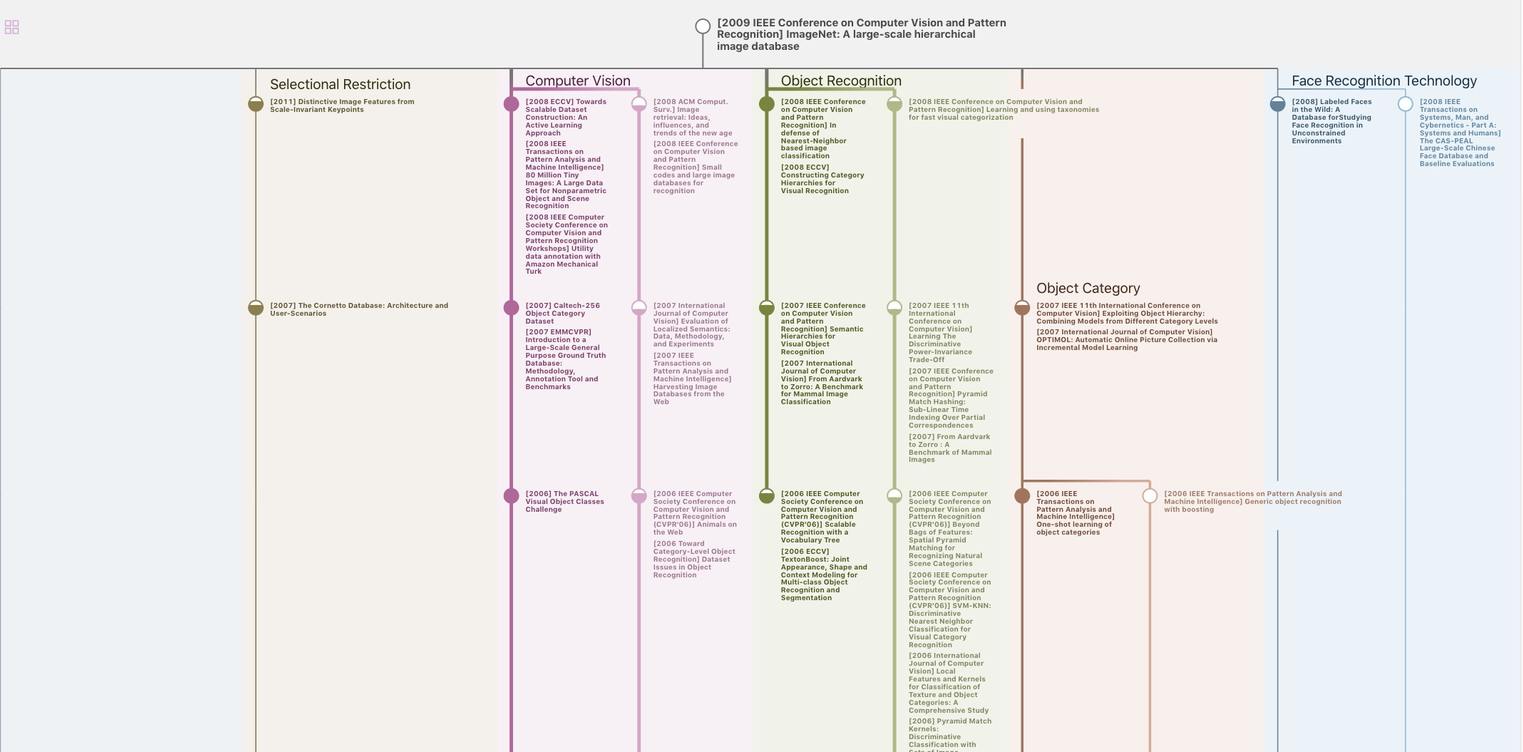
生成溯源树,研究论文发展脉络
Chat Paper
正在生成论文摘要