LSTM-based post-processing for noise reduction in SVD-based particulate matter digital twinning on lightweight embedded devices
ELECTRONICS LETTERS(2024)
摘要
Among various applications of digital twins, measuring particulate matter in the context of air pollution has become increasingly important due to growing interest in atmospheric environments. By obtaining particle count values from analogue-to-digital converter raw data through photodiode and calibrating them, particulate matter values can be acquired. The singular-value-decomposition is efficient transfer function learning method. However, due to the linear nature of the transfer function of singular-value-decomposition, it also conveys noise information, necessitating post-processing to suppress noise. This study proposes a method that utilizes long short-term memory neural networks to effectively stabilize noise based on the output of continuous dust sensor particulate matter distributions. Using the singular value decomposition based particulate matter shows relative root-mean-square error (4.4761, 100%) as a reference, the proposed long short-term memory post-processing demonstrates an improved result (2.9328, 65.52%) compared to other post-processing method: the mean filter (3.6704, 82.00%), low-pass filter (3.7719, 84.27%) and Kalman filter (3.5550, 79.42%). Furthermore, to address the limitation of initial delay in long short-term memory to achieve stable data output, a method of iteratively training the initial input data sample is proposed. For the initial input data, the non-initialization approach exhibited a relative error of 87.57% and it took around 5 samples to predict stable output. In contrast, the proposed iterative training method, applied five times to the initial data sample, achieved a relative error of 9.51% and immediately obtained stable data. Concept of digital twin of dust sensing: (a) High-cost dust sensing system with single high-performance sensor, (b) low-cost dust sensing system with additional low-performance sensors, (c) proposed dust sensing system with low-performance sensor by replicating high-performance sensor. image
更多查看译文
关键词
intelligent sensors,measurement systems,signal processing
AI 理解论文
溯源树
样例
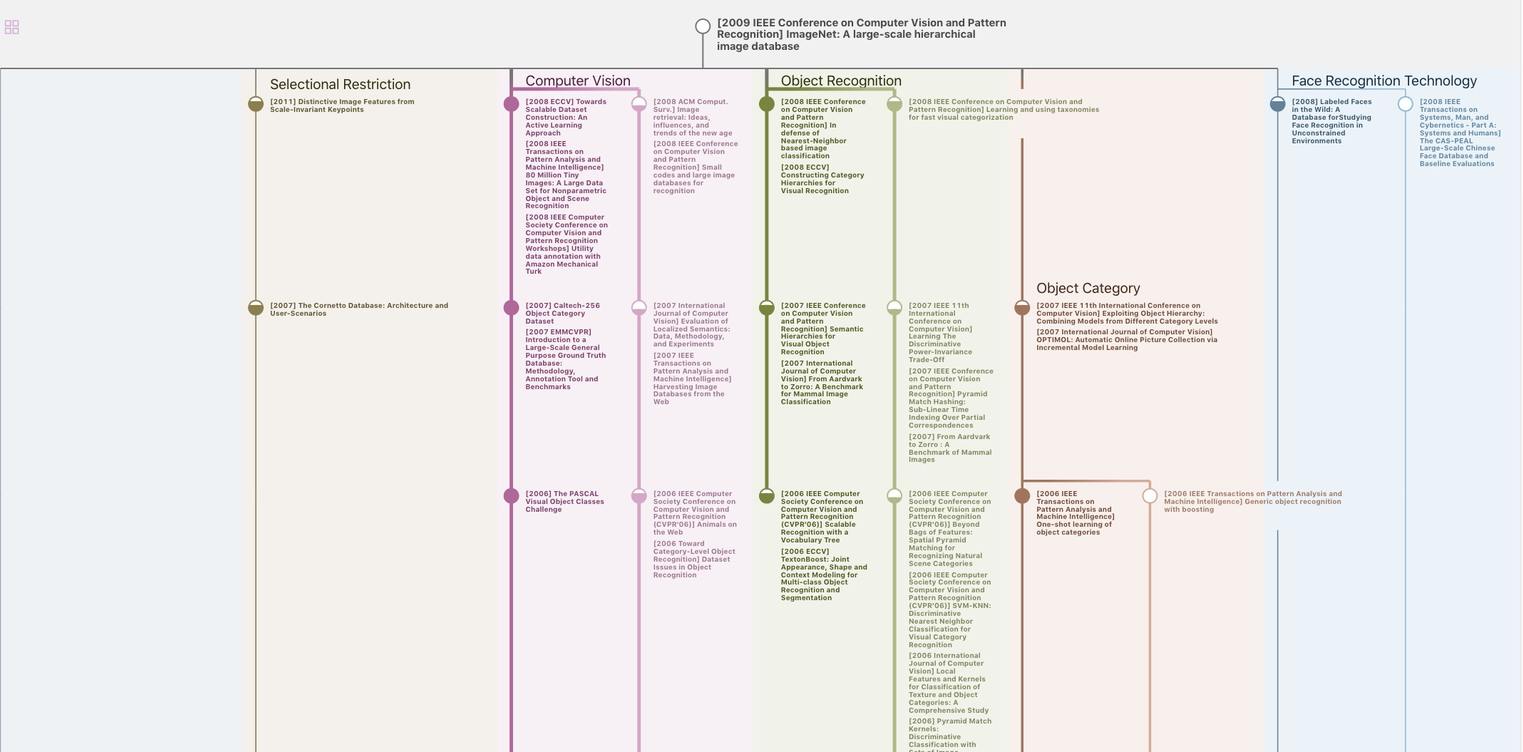
生成溯源树,研究论文发展脉络
Chat Paper
正在生成论文摘要