DPR-GAN: Dual-Stream Progressive Refinement for Adversarial 3D Point Cloud Generation
Neural Processing Letters(2024)
摘要
Point cloud generation aims to transfer a latent code to realistic 3D shapes through generative models. However, most of progressive generative methods ignore the spatial relationship among different stages and suffer from the loss of contextual information. To address this issue, we propose dual-stream progressive refinement adversarial network (DPR-GAN), which utilizes a dual-stream structure to establish the relationship between two adjacent stages. Such a mechanism can learn the spatial context and preserve more spatial details of point clouds at different stages. In addition, DPR-GAN adopts 3D gridding transformation to guide the shape deformation. In this way, 3D gridding transformation can learn a reasonable correspondence between the local regions of 3D shapes and latent codes. Benefiting from the uniformity and adaptability of the 3D grids, our proposed DPR-GAN can improve the quality and consistency of generated point clouds. We conduct comprehensive experiments to demonstrate that the proposed DPR-GAN is capable of generating pluralistic point clouds, as compared with state-of-the-art generation methods in terms of both visual and quantitative evaluations.
更多查看译文
关键词
Point cloud generation,Generative adversarial network,Progressive generation,3D shape transformation
AI 理解论文
溯源树
样例
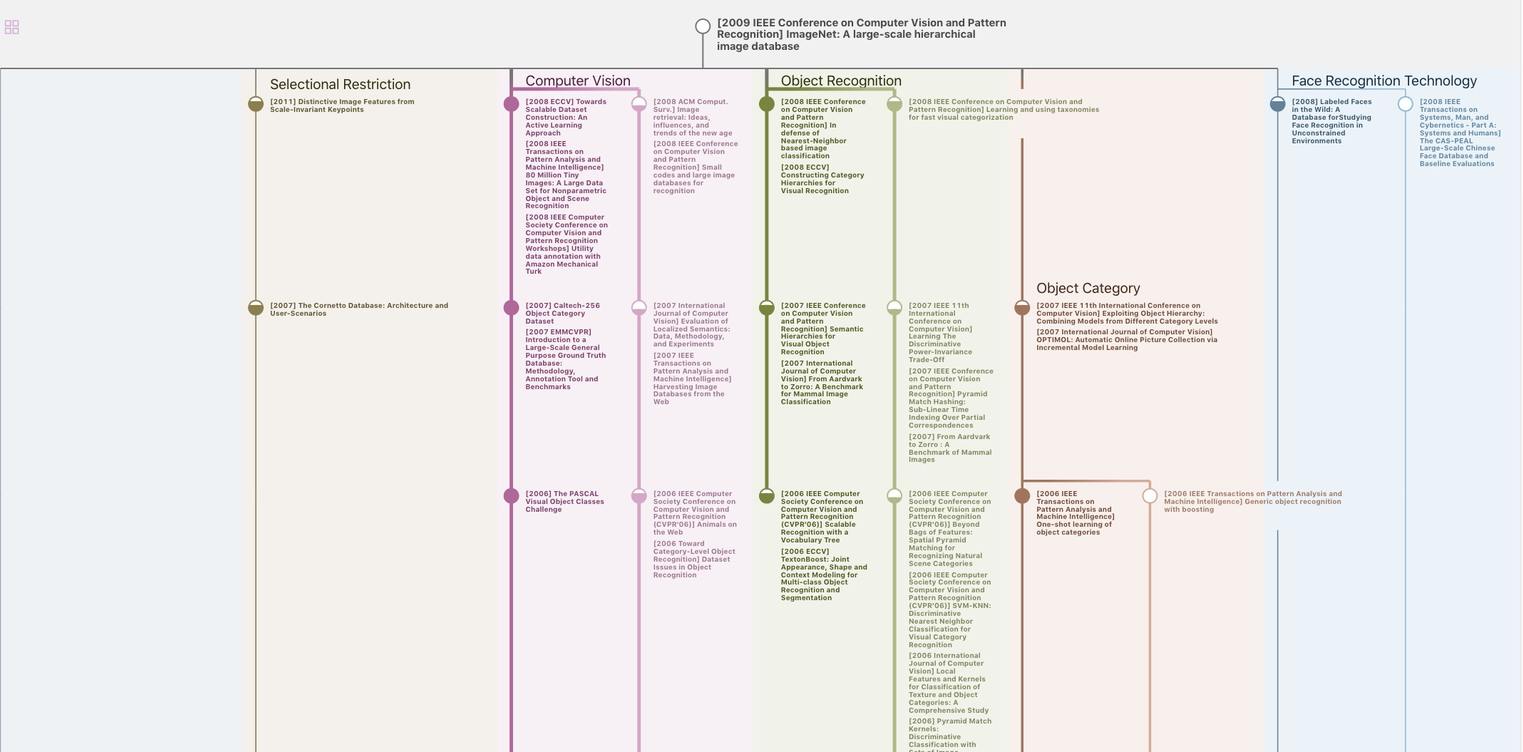
生成溯源树,研究论文发展脉络
Chat Paper
正在生成论文摘要