High kinetic energy mesoscale eddy identification based on multi-task learning and multi-source data
INTERNATIONAL JOURNAL OF APPLIED EARTH OBSERVATION AND GEOINFORMATION(2024)
摘要
Mesoscale eddies can significantly influence the surface and deep layers of the ocean, leading to diverse outcomes. Particularly, high -amplitude mesoscale eddies possess vertical structures that can reach thousands of meters, carrying greater kinetic energy. Accurately detecting such eddies is crucial for various applications, ranging from promoting marine economic development to ensuring navigational safety. This study presents a cutting -edge algorithm for mesoscale eddy identification, leveraging multi -task learning and multi -source data. Initially, we investigated the impacts of mesoscale eddies of different amplitudes on their surrounding environments. Following this, we compiled a multi -source dataset of mesoscale eddies in the South China Sea by leveraging the diverse attributes presented by eddies in various surface layers of the sea. Our approach involves extracting multiple features of mesoscale eddies from multi -source data and fusing them, along with designing a mix residual module to enhance feature extraction capabilities. Finally, we propose a novel method based on multi -task learning, which concurrently learns the segmentation and contour of mesoscale eddies. This is supported by an optimized loss function for simultaneous learning. Strict validation of mesoscale eddy detection in the South China Sea region demonstrates the effectiveness of the proposed method. The empirical results emphasize the algorithm's capabilities in mitigating contour loss (a common challenge in deep network architectures), improving detection accuracy, and efficiently generating results for eddy contour and categorical segmentation tasks.
更多查看译文
关键词
Mesoscale eddy,Deep learning,Contour detection,Multi-source features
AI 理解论文
溯源树
样例
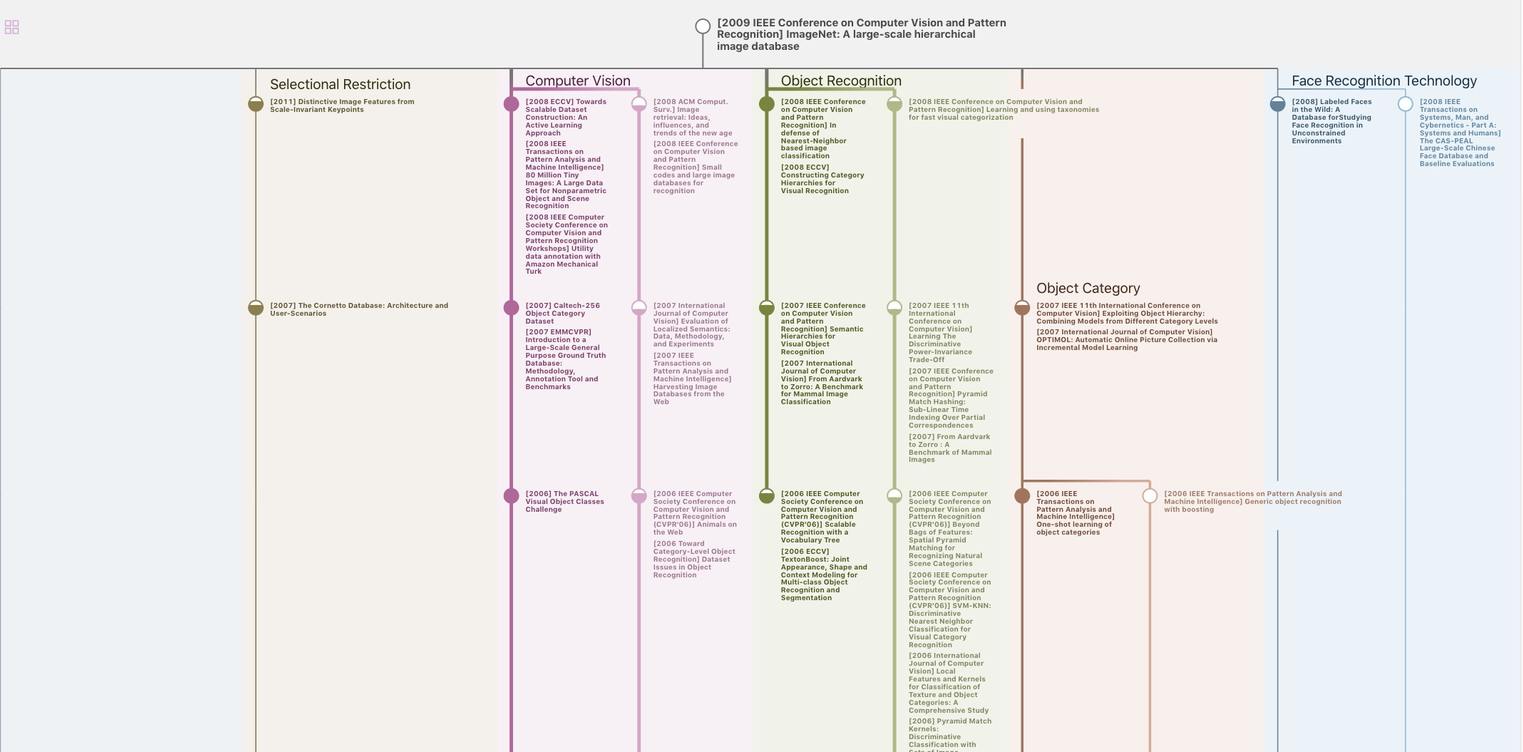
生成溯源树,研究论文发展脉络
Chat Paper
正在生成论文摘要