Semi-supervised auto-segmentation method for pelvic organ-at-risk in magnetic resonance images based on deep-learning
JOURNAL OF APPLIED CLINICAL MEDICAL PHYSICS(2024)
摘要
Background and purposeIn radiotherapy, magnetic resonance (MR) imaging has higher contrast for soft tissues compared to computed tomography (CT) scanning and does not emit radiation. However, manual annotation of the deep learning-based automatic organ-at-risk (OAR) delineation algorithms is expensive, making the collection of large-high-quality annotated datasets a challenge. Therefore, we proposed the low-cost semi-supervised OAR segmentation method using small pelvic MR image annotations.MethodsWe trained a deep learning-based segmentation model using 116 sets of MR images from 116 patients. The bladder, femoral heads, rectum, and small intestine were selected as OAR regions. To generate the training set, we utilized a semi-supervised method and ensemble learning techniques. Additionally, we employed a post-processing algorithm to correct the self-annotation data. Both 2D and 3D auto-segmentation networks were evaluated for their performance. Furthermore, we evaluated the performance of semi-supervised method for 50 labeled data and only 10 labeled data.ResultsThe Dice similarity coefficient (DSC) of the bladder, femoral heads, rectum and small intestine between segmentation results and reference masks is 0.954, 0.984, 0.908, 0.852 only using self-annotation and post-processing methods of 2D segmentation model. The DSC of corresponding OARs is 0.871, 0.975, 0.975, 0.783, 0.724 using 3D segmentation network, 0.896, 0.984, 0.890, 0.828 using 2D segmentation network and common supervised method.ConclusionThe outcomes of our study demonstrate that it is possible to train a multi-OAR segmentation model using small annotation samples and additional unlabeled data. To effectively annotate the dataset, ensemble learning and post-processing methods were employed. Additionally, when dealing with anisotropy and limited sample sizes, the 2D model outperformed the 3D model in terms of performance.
更多查看译文
关键词
auto-segmentation,deep-learning,semi-supervised learning
AI 理解论文
溯源树
样例
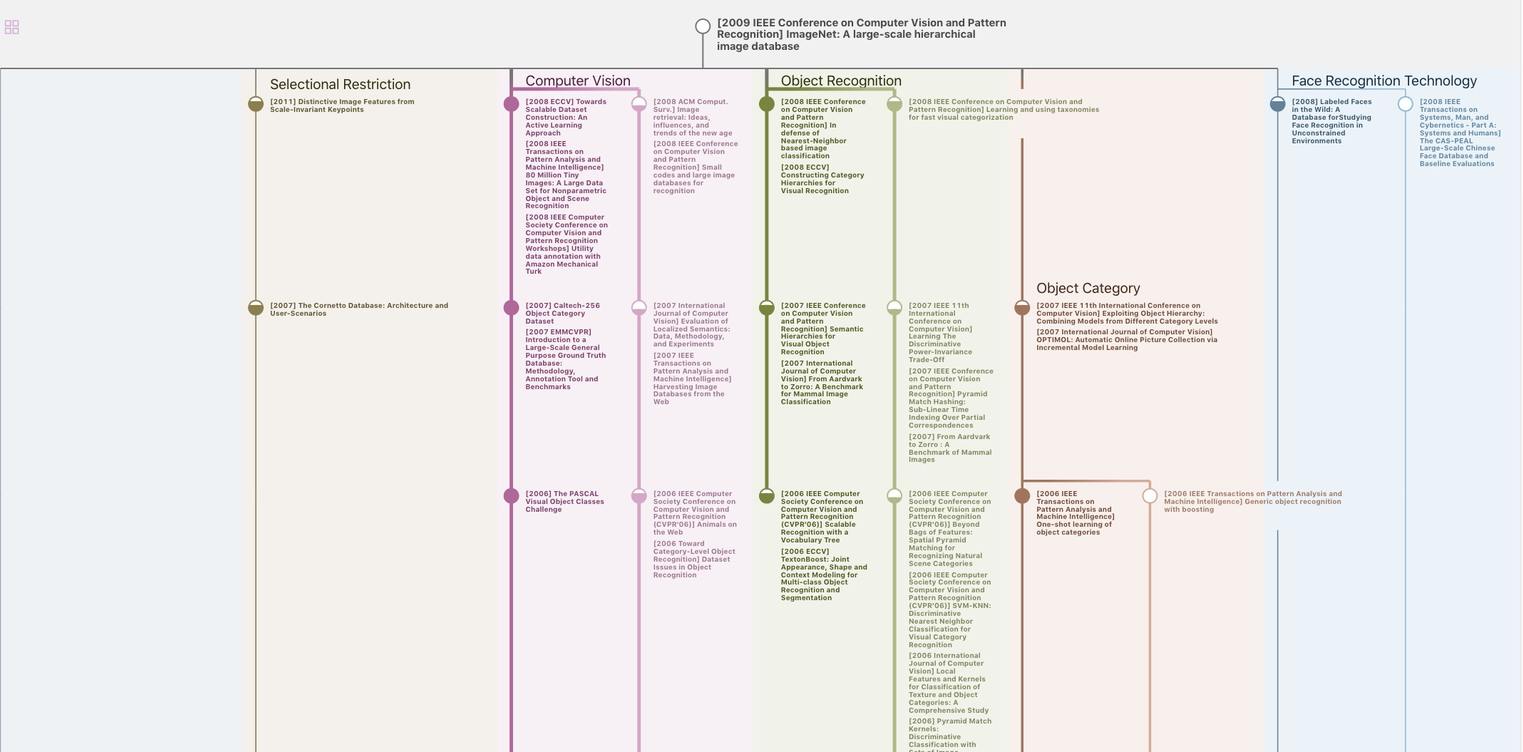
生成溯源树,研究论文发展脉络
Chat Paper
正在生成论文摘要