Advancing Human Motion Recognition with SkeletonCLIP++: Weighted Video Feature Integration and Enhanced Contrastive Sample Discrimination.
Sensors (Basel, Switzerland)(2024)
Abstract
This paper introduces 'SkeletonCLIP++', an extension of our prior work in human action recognition, emphasizing the use of semantic information beyond traditional label-based methods. The innovation, 'Weighted Frame Integration' (WFI), shifts video feature computation from averaging to a weighted frame approach, enabling a more nuanced representation of human movements in line with semantic relevance. Another key development, 'Contrastive Sample Identification' (CSI), introduces a novel discriminative task within the model. This task involves identifying the most similar negative sample among positive ones, enhancing the model's ability to distinguish between closely related actions. Incorporating the 'BERT Text Encoder Integration' (BTEI) leverages the pre-trained BERT model as our text encoder to refine the model's performance. Empirical evaluations on HMDB-51, UCF-101, and NTU RGB+D 60 datasets illustrate positive improvements, especially in smaller datasets. 'SkeletonCLIP++' thus offers a refined approach to human action recognition, ensuring semantic integrity and detailed differentiation in video data analysis.
MoreTranslated text
Key words
action recognition,multi-modal fusion,contrastive learning
AI Read Science
Must-Reading Tree
Example
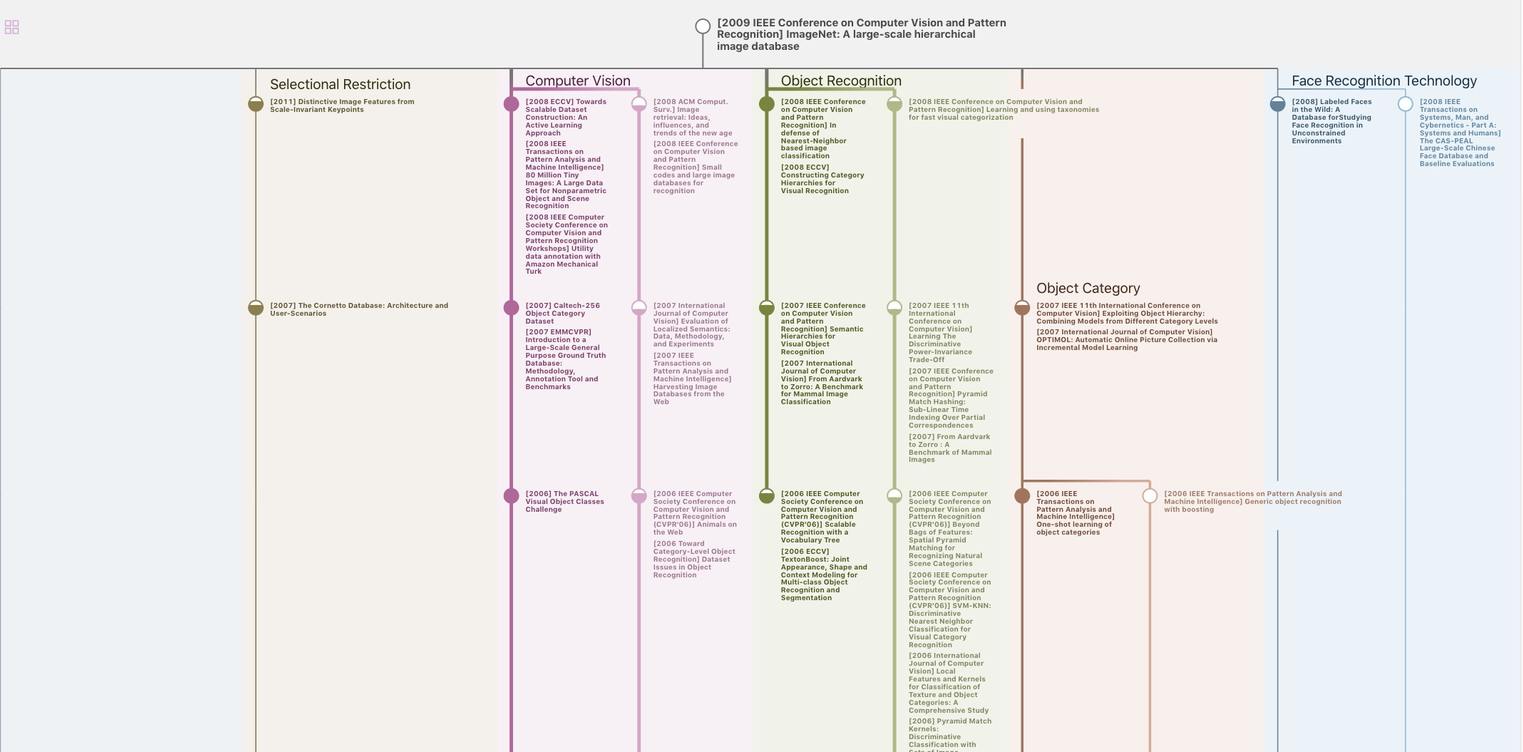
Generate MRT to find the research sequence of this paper
Chat Paper
Summary is being generated by the instructions you defined