Quantitative Detection of Pipeline Cracks Based on Ultrasonic Guided Waves and Convolutional Neural Network
SENSORS(2024)
摘要
In this study, a quantitative detection method of pipeline cracks based on a one-dimensional convolutional neural network (1D-CNN) was developed using the time-domain signal of ultrasonic guided waves and the crack size of the pipeline as the input and output, respectively. Pipeline ultrasonic guided wave detection signals under different crack defect conditions were obtained via numerical simulations and experiments, and these signals were input as features into a multi-layer perceptron and one-dimensional convolutional neural network (1D-CNN) for training. The results revealed that the 1D-CNN performed better in the quantitative analysis of pipeline crack defects, with an error of less than 2% in the simulated and experimental data, and it could effectively evaluate the size of crack defects from the echo signals under different frequency excitations. Thus, by combining the ultrasonic guided wave detection technology and CNN, a quantitative analysis of pipeline crack defects can be effectively realized.
更多查看译文
关键词
convolutional neural network,ultrasonic guided wave,pipeline,crack defects
AI 理解论文
溯源树
样例
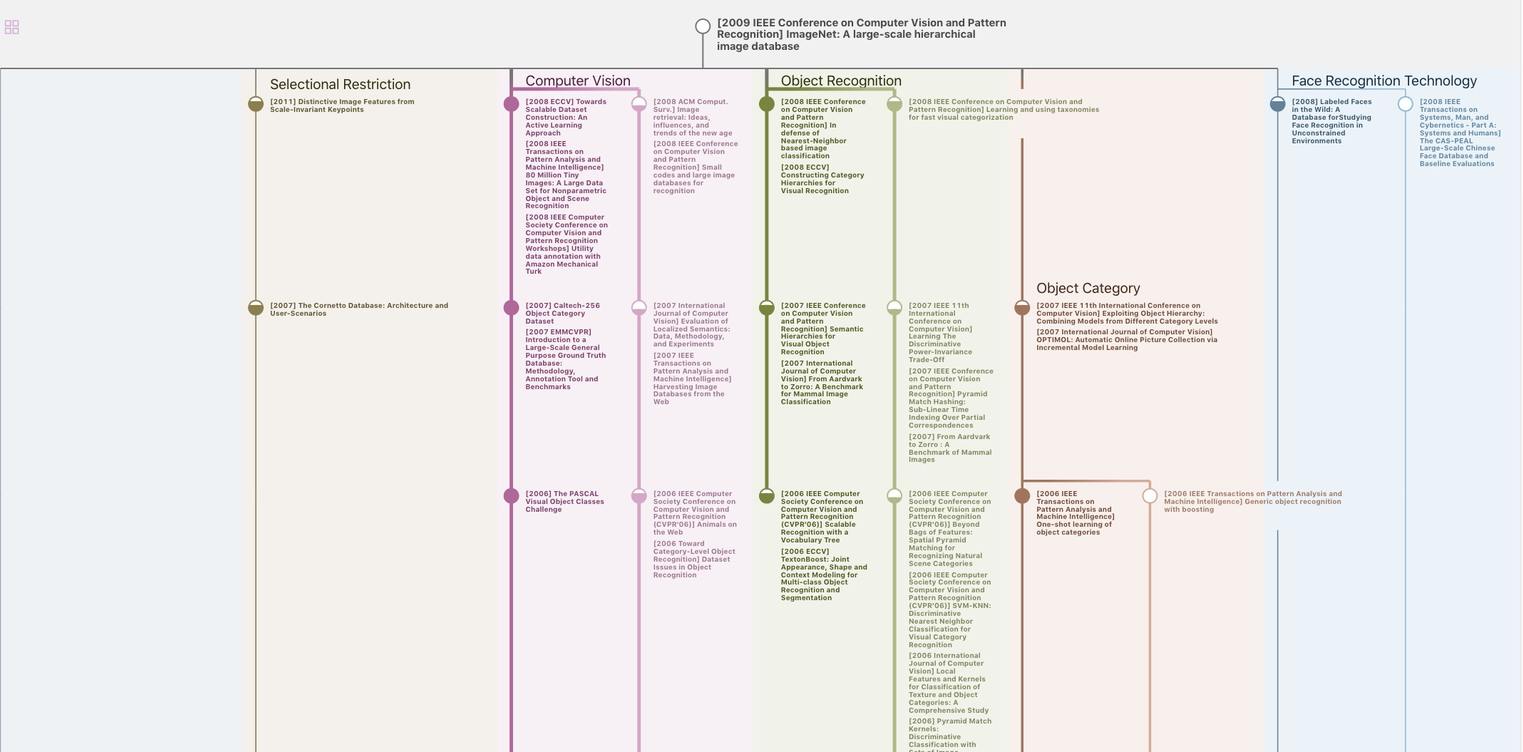
生成溯源树,研究论文发展脉络
Chat Paper
正在生成论文摘要