Skeleton-Based Activity Recognition for Process-Based Quality Control of Concealed Work via Spatial-Temporal Graph Convolutional Networks
SENSORS(2024)
摘要
Computer vision (CV)-based recognition approaches have accelerated the automation of safety and progress monitoring on construction sites. However, limited studies have explored its application in process-based quality control of construction works, especially for concealed work. In this study, a framework is developed to facilitate process-based quality control utilizing Spatial-Temporal Graph Convolutional Networks (ST-GCNs). To test this model experimentally, we used an on-site collected plastering work video dataset to recognize construction activities. An ST-GCN model was constructed to identify the four primary activities in plastering works, which attained 99.48% accuracy on the validation set. Then, the ST-GCN model was employed to recognize the activities of three extra videos, which represented a process with four activities in the correct order, a process without the activity of fiberglass mesh covering, and a process with four activities but in the wrong order, respectively. The results indicated that activity order could be clearly withdrawn from the activity recognition result of the model. Hence, it was convenient to judge whether key activities were missing or in the wrong order. This study has identified a promising framework that has the potential to the development of active, real-time, process-based quality control at construction sites.
更多查看译文
关键词
activity recognition,construction,progress management,quality control,ST-GCN
AI 理解论文
溯源树
样例
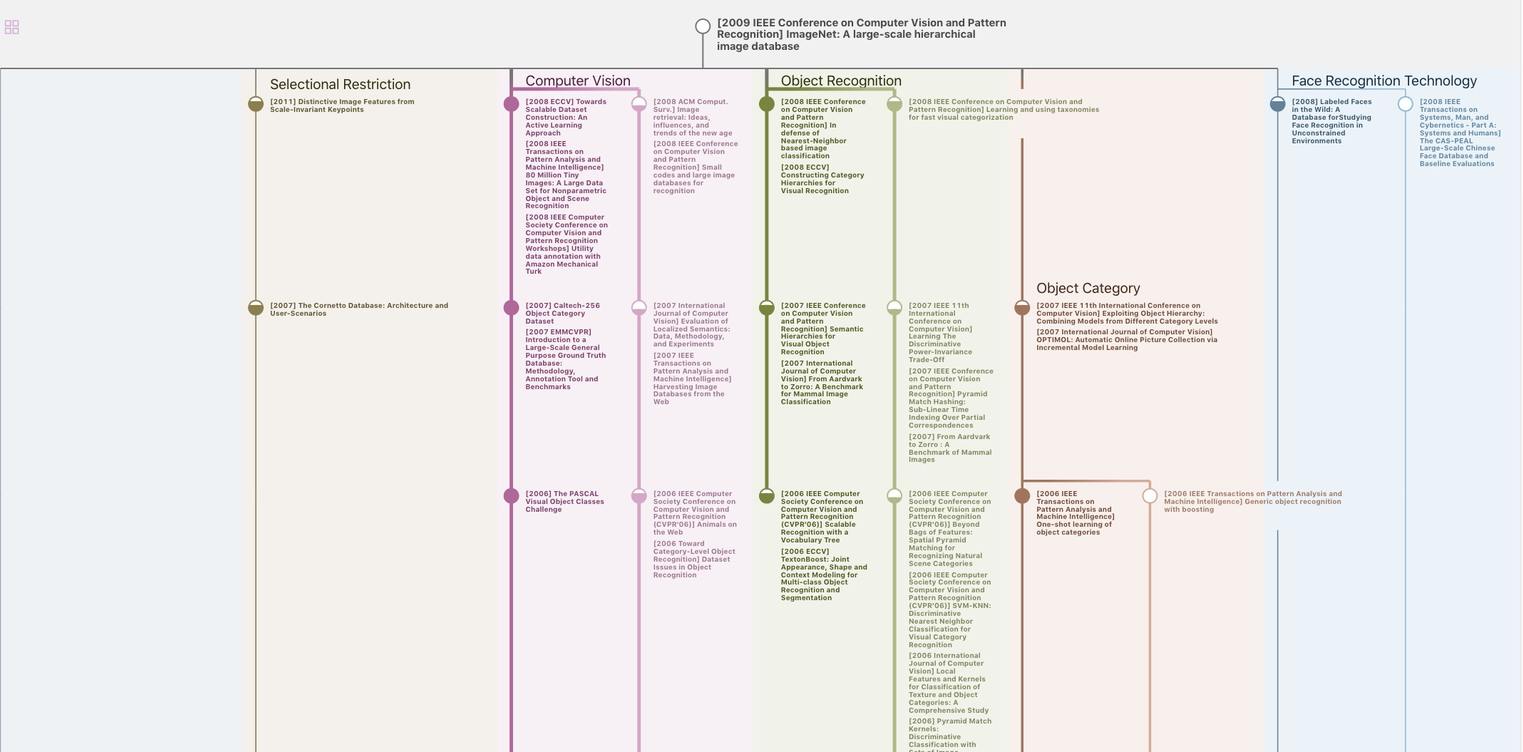
生成溯源树,研究论文发展脉络
Chat Paper
正在生成论文摘要