Fault Diagnosis of the Rolling Bearing by a Multi-Task Deep Learning Method Based on a Classifier Generative Adversarial Network
SENSORS(2024)
摘要
Accurate fault diagnosis is essential for the safe operation of rotating machinery. Recently, traditional deep learning-based fault diagnosis have achieved promising results. However, most of these methods focus only on supervised learning and tend to use small convolution kernels non-effectively to extract features that are not controllable and have poor interpretability. To this end, this study proposes an innovative semi-supervised learning method for bearing fault diagnosis. Firstly, multi-scale dilated convolution squeeze-and-excitation residual blocks are designed to exact local and global features. Secondly, a classifier generative adversarial network is employed to achieve multi-task learning. Both unsupervised and supervised learning are performed simultaneously to improve the generalization ability. Finally, supervised learning is applied to fine-tune the final model, which can extract multi-scale features and be further improved by implicit data augmentation. Experiments on two datasets were carried out, and the results verified the superiority of the proposed method.
更多查看译文
关键词
rolling bearing,intelligent fault diagnosis,adversarial generative network,multi-task learning,semi-supervised learning
AI 理解论文
溯源树
样例
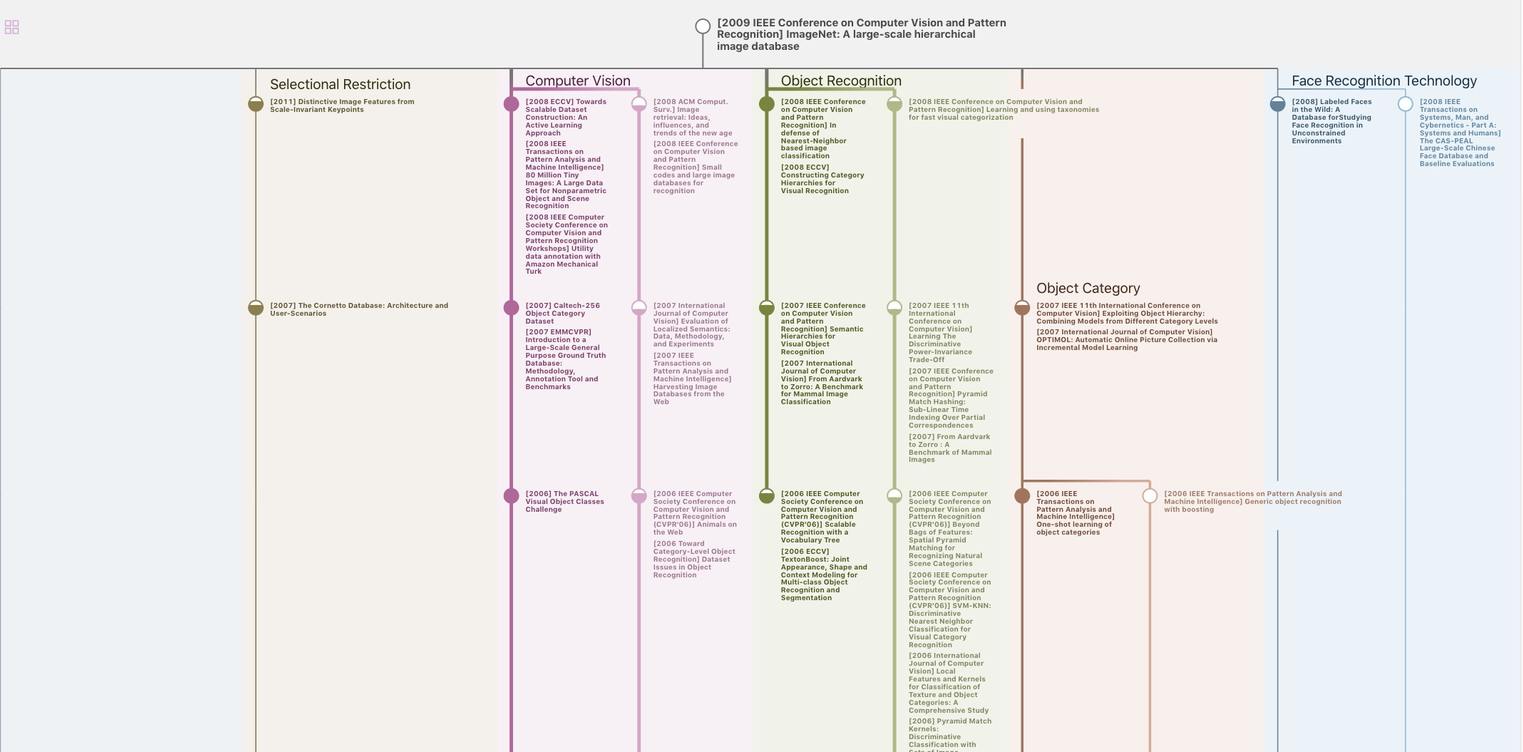
生成溯源树,研究论文发展脉络
Chat Paper
正在生成论文摘要