A Lightweight Image Super-Resolution Reconstruction Algorithm Based on the Residual Feature Distillation Mechanism
SENSORS(2024)
摘要
In recent years, the development of image super-resolution (SR) has explored the capabilities of convolutional neural networks (CNNs). The current research tends to use deeper CNNs to improve performance. However, blindly increasing the depth of the network does not effectively enhance its performance. Moreover, as the network depth increases, more issues arise during the training process, requiring additional training techniques. In this paper, we propose a lightweight image super-resolution reconstruction algorithm (SISR-RFDM) based on the residual feature distillation mechanism (RFDM). Building upon residual blocks, we introduce spatial attention (SA) modules to provide more informative cues for recovering high-frequency details such as image edges and textures. Additionally, the output of each residual block is utilized as hierarchical features for global feature fusion (GFF), enhancing inter-layer information flow and feature reuse. Finally, all these features are fed into the reconstruction module to restore high-quality images. Experimental results demonstrate that our proposed algorithm outperforms other comparative algorithms in terms of both subjective visual effects and objective evaluation quality. The peak signal-to-noise ratio (PSNR) is improved by 0.23 dB, and the structural similarity index (SSIM) reaches 0.9607.
更多查看译文
关键词
super-resolution,spatial attention,residual feature distillation,image processing,global fusion
AI 理解论文
溯源树
样例
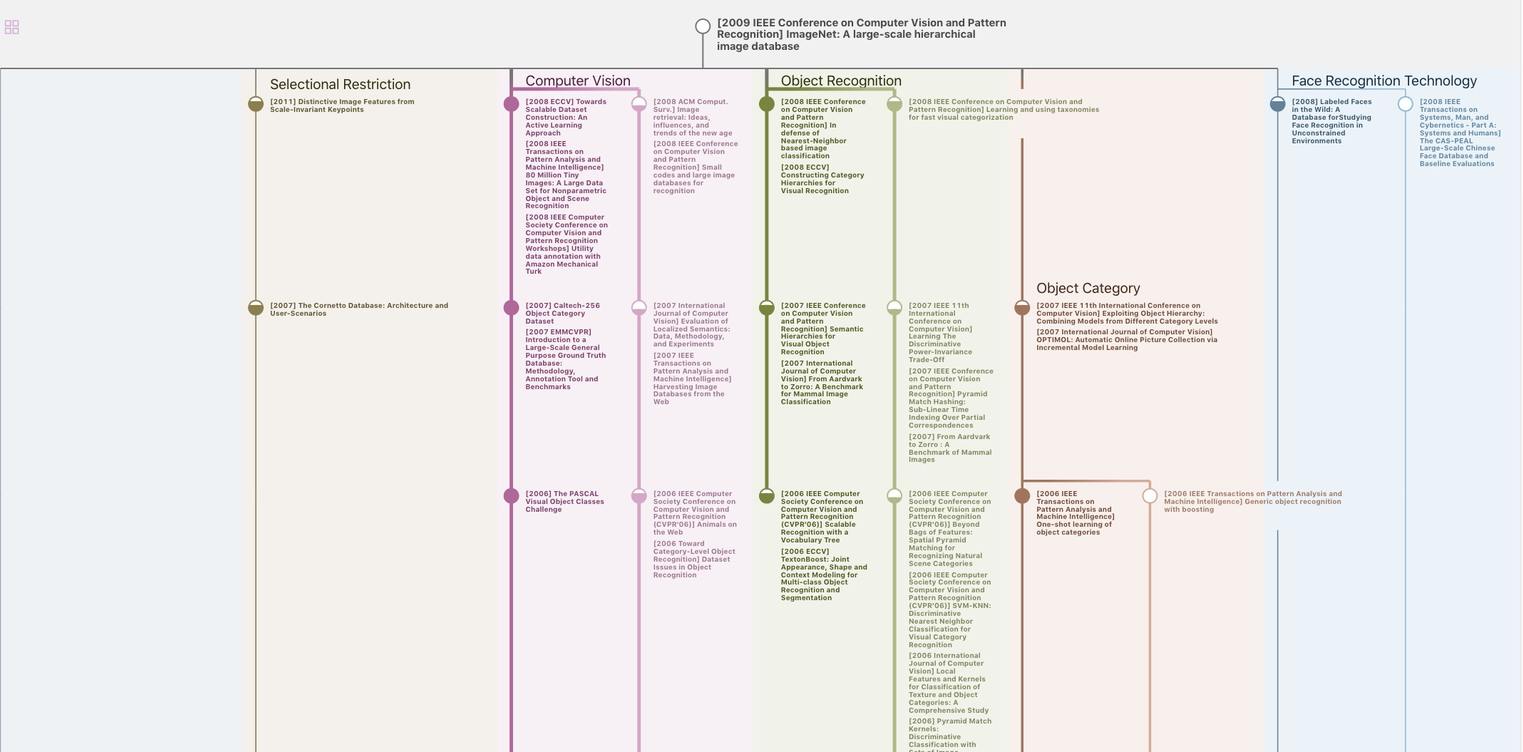
生成溯源树,研究论文发展脉络
Chat Paper
正在生成论文摘要