A Hybrid Initialization and Effective Reproduction-Based Evolutionary Algorithm for Tackling Bi-Objective Large-Scale Feature Selection in Classification
MATHEMATICS(2024)
摘要
Evolutionary algorithms have been widely used for tackling multi-objective optimization problems, while feature selection in classification can also be seen as a discrete bi-objective optimization problem that pursues minimizing both the classification error and the number of selected features. However, traditional multi-objective evolutionary algorithms (MOEAs) can encounter setbacks when the dimensionality of features explodes to a large scale, i.e., the curse of dimensionality. Thus, in this paper, we focus on designing an adaptive MOEA framework for solving bi-objective feature selection, especially on large-scale datasets, by adopting hybrid initialization and effective reproduction (called HIER). The former attempts to improve the starting state of evolution by composing a hybrid initial population, while the latter tries to generate more effective offspring by modifying the whole reproduction process. Moreover, the statistical experiment results suggest that HIER generally performs the best on most of the 20 test datasets, compared with six state-of-the-art MOEAs, in terms of multiple metrics covering both optimization and classification performances. Then, the component contribution of HIER is also studied, suggesting that each of its essential components has a positive effect. Finally, the computational time complexity of HIER is also analyzed, suggesting that HIER is not time-consuming at all and shows promising computational efficiency.
更多查看译文
关键词
bi-objective optimization,evolutionary algorithm,effective reproduction,hybrid initialization,large-scale feature selection
AI 理解论文
溯源树
样例
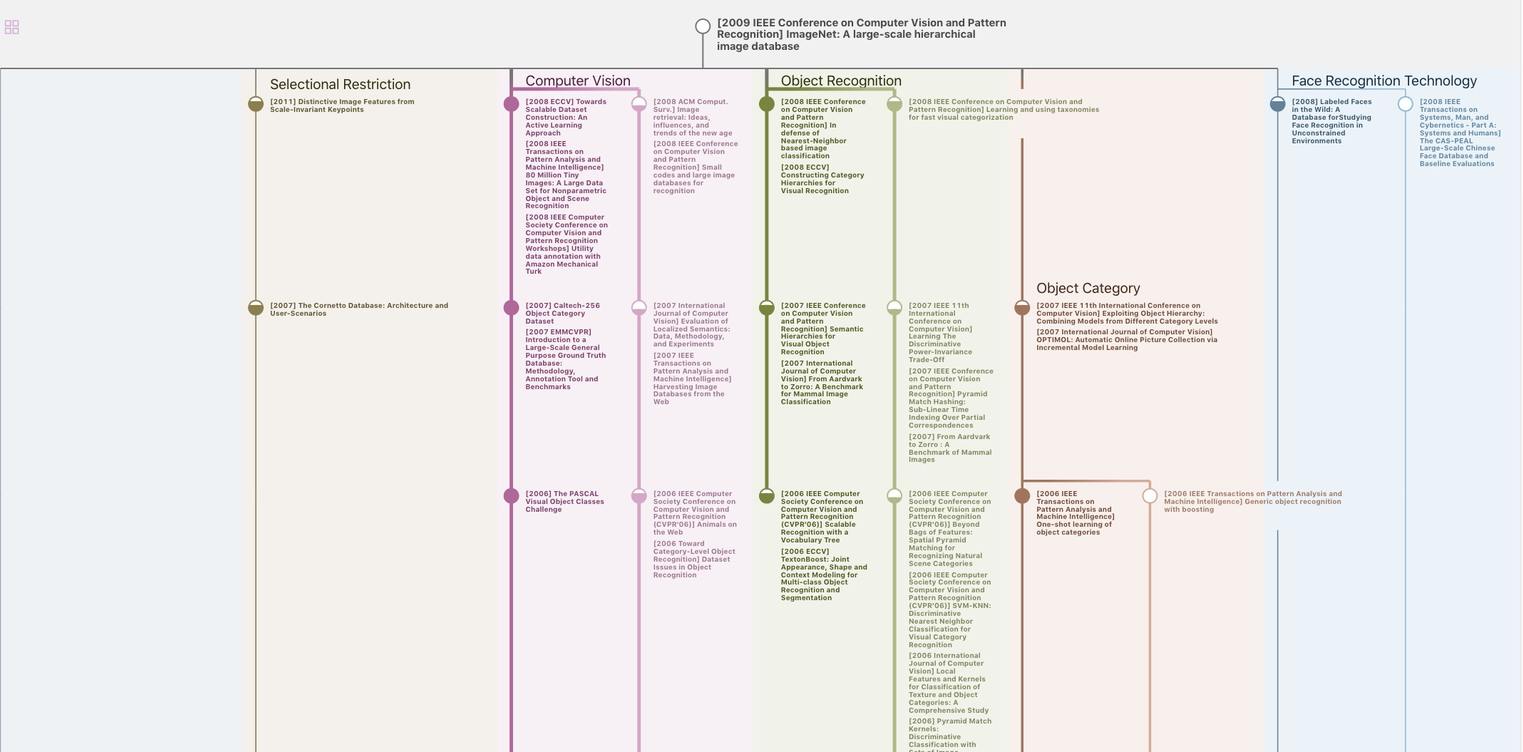
生成溯源树,研究论文发展脉络
Chat Paper
正在生成论文摘要