Lightweight Machine Learning Method for Real-Time Espresso Analysis
ELECTRONICS(2024)
摘要
Coffee crema plays a crucial role in assessing the quality of espresso. In recent years, in response to the rising labor costs, aging population, remote security/authentication needs, civic awareness, and the growing preference for non-face-to-face interactions, robot cafes have emerged. While some people seek sentiment and premium coffee, there are also many who desire quick and affordable options. To align with the trends of this era, there is a need for lightweight artificial intelligence algorithms for easy and quick decision making, as well as monitoring the extraction process in these automated cafes. However, the application of these technologies to actual coffee machines has been limited. In this study, we propose an innovative real-time coffee crema control system that integrates lightweight machine learning algorithms. We employ the GrabCut algorithm to segment the crema region from the rest of the image and use a clustering algorithm to determine the optimal brewing conditions for each cup of espresso based on the characteristics of the crema extracted. Our results demonstrate that our approach can accurately analyze coffee crema in real time. This research proposes a promising direction by leveraging computer vision and machine learning technologies to enhance the efficiency and consistency of coffee brewing. Such an approach enables the prediction of component replacement timing in coffee machines, such as the replacement of water filters, and provides administrators with Before Service. This could lead to the development of fully automated artificial intelligence coffee making systems in the future.
更多查看译文
关键词
machine learning,color clustering,GrabCut,coffee crema,espresso
AI 理解论文
溯源树
样例
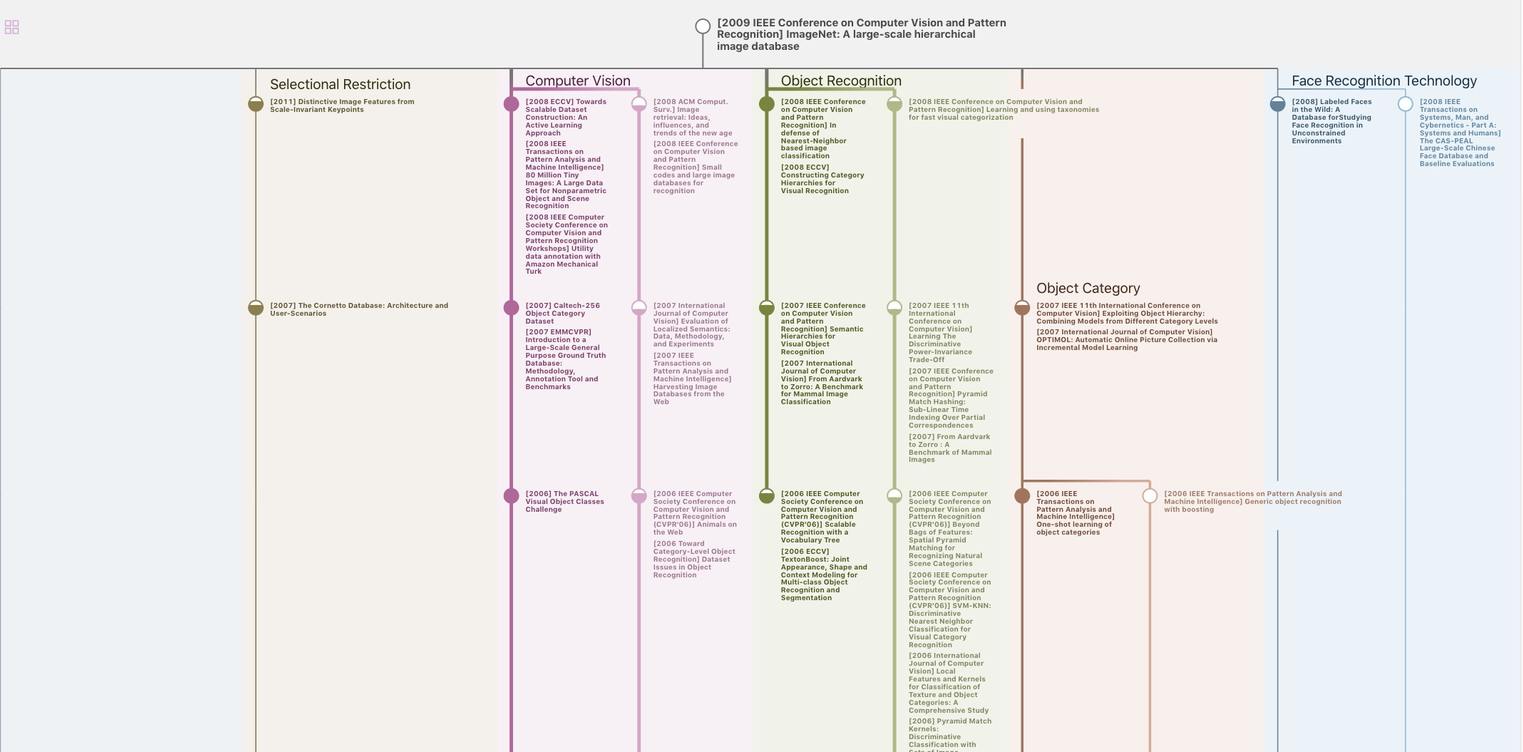
生成溯源树,研究论文发展脉络
Chat Paper
正在生成论文摘要