AI-Based Detection of Oral Squamous Cell Carcinoma with Raman Histology
CANCERS(2024)
摘要
Simple Summary Stimulated Raman Histology (SRH) is a technique that uses laser light to create detailed images of tissues without the need for traditional staining. This study aimed to use deep learning to classify oral squamous cell carcinoma (OSCC) and different non-malignant tissue types using SRH images. The performances of the classifications between SRH images and the original images obtained from stimulated Raman scattering (SRS) were compared. A deep learning model was trained on 64 images and tested on 16, showing that it could effectively identify tissue types during surgery, potentially speeding up decision making in oral cancer surgery.Abstract Stimulated Raman Histology (SRH) employs the stimulated Raman scattering (SRS) of photons at biomolecules in tissue samples to generate histological images. Subsequent pathological analysis allows for an intraoperative evaluation without the need for sectioning and staining. The objective of this study was to investigate a deep learning-based classification of oral squamous cell carcinoma (OSCC) and the sub-classification of non-malignant tissue types, as well as to compare the performances of the classifier between SRS and SRH images. Raman shifts were measured at wavenumbers k1 = 2845 cm-1 and k2 = 2930 cm-1. SRS images were transformed into SRH images resembling traditional H&E-stained frozen sections. The annotation of 6 tissue types was performed on images obtained from 80 tissue samples from eight OSCC patients. A VGG19-based convolutional neural network was then trained on 64 SRS images (and corresponding SRH images) and tested on 16. A balanced accuracy of 0.90 (0.87 for SRH images) and F1-scores of 0.91 (0.91 for SRH) for stroma, 0.98 (0.96 for SRH) for adipose tissue, 0.90 (0.87 for SRH) for squamous epithelium, 0.92 (0.76 for SRH) for muscle, 0.87 (0.90 for SRH) for glandular tissue, and 0.88 (0.87 for SRH) for tumor were achieved. The results of this study demonstrate the suitability of deep learning for the intraoperative identification of tissue types directly on SRS and SRH images.
更多查看译文
关键词
machine learning,neural networks,pathology,computational biology,head and neck neoplasms
AI 理解论文
溯源树
样例
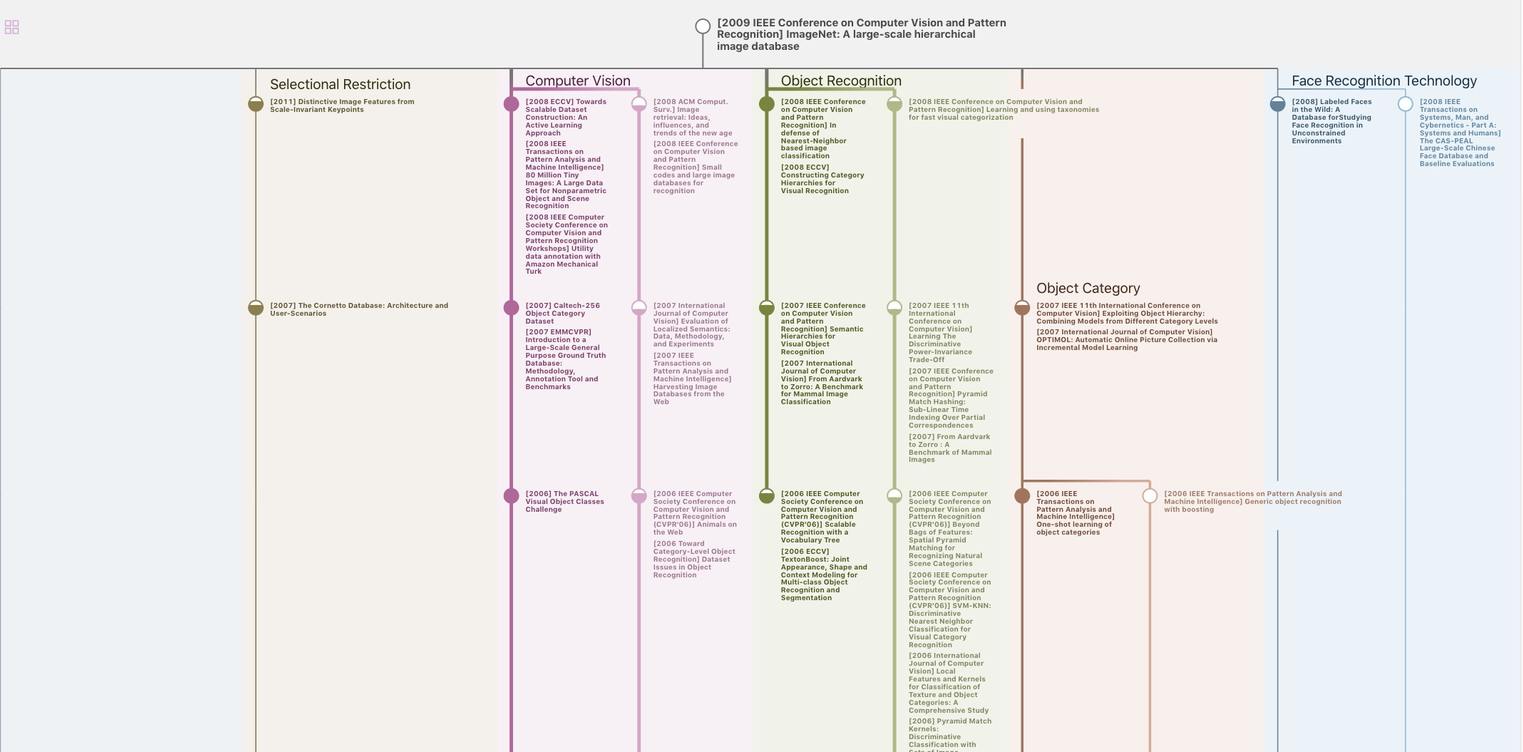
生成溯源树,研究论文发展脉络
Chat Paper
正在生成论文摘要