A Unified Spatial-Pressure Sensitivity Partitioning and Leakage Detection Method within a Deep Learning Framework
WATER(2024)
Abstract
This study introduces an innovative approach for leak detection in water distribution systems (WDSs), integrating three-order embedding, k-means clustering, and long short-term memory (LSTM) networks, with pressure-sensitive analysis techniques. This comprehensive methodology segments the network into distinct partitions, utilizes simulated leak events to train the deep learning networks, and establishes a sophisticated model for accurately identifying leak partitions. This approach generates a leak dataset by adjusting water demands, which could effectively pinpoint the leaks in a specific partition by leveraging both the pressure sensitivity and spatial coordinates of nodes, allowing for the elimination of the need for manual work and precise identification of leaks in targeted areas. Through the analysis of two case studies, the model demonstrates its ability to effectively pinpoint potential leak partitions, significantly enhancing operational efficiency and reliability in managing the complex problems of urban water resource management. This approach not only optimizes leak detection but also paves the way for advanced, data-driven strategies in WDSs, ensuring sustainable and secure water distribution in urban settings.
MoreTranslated text
Key words
leak detection,deep learning,long short-term memory (LSTM),embedding,network partition
AI Read Science
Must-Reading Tree
Example
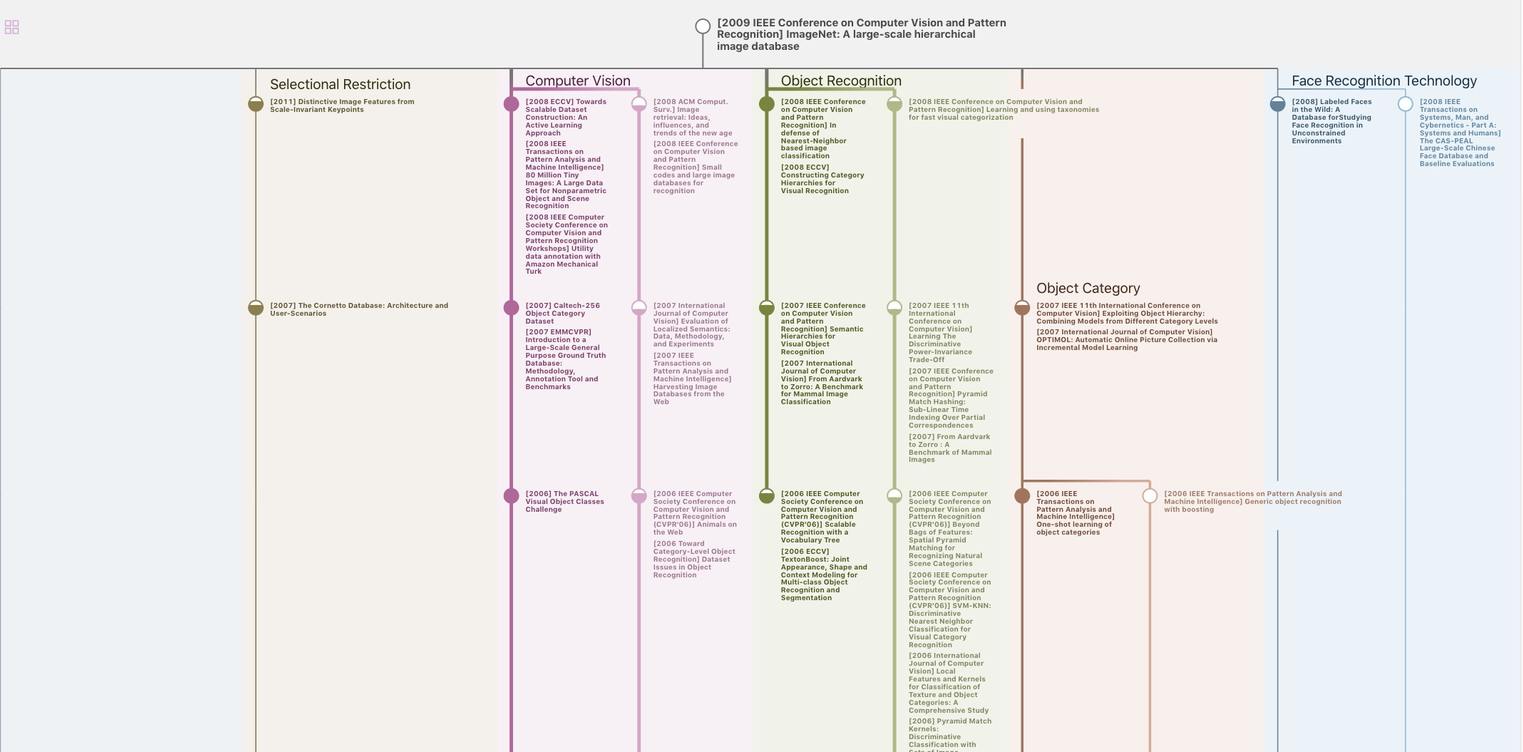
Generate MRT to find the research sequence of this paper
Chat Paper
Summary is being generated by the instructions you defined