Monthly Streamflow Prediction of the Source Region of the Yellow River Based on Long Short-Term Memory Considering Different Lagged Months
WATER(2024)
摘要
Accurate and reliable monthly streamflow prediction plays a crucial role in the scientific allocation and efficient utilization of water resources. In this paper, we proposed a prediction framework that integrates the input variable selection method and Long Short-Term Memory (LSTM). The input selection methods, including autocorrelation function (ACF), partial autocorrelation function (PACF), and time lag cross-correlation (TLCC), were used to analyze the lagged time between variables. Then, the performance of the LSTM model was compared with three other traditional methods. The framework was used to predict monthly streamflow at the Jimai, Maqu, and Tangnaihai stations in the source area of the Yellow River. The results indicated that grid search and cross-validation can improve the efficiency of determining model parameters. The models incorporating ACF, PACF, and TLCC with lagged time are evidently superior to the models using the current variable as the model inputs. Furthermore, the LSTM model, which considers the lagged time, demonstrated better performance in predicting monthly streamflow. The coefficient of determination (R2) improved by an average of 17.46%, 33.94%, and 15.29% for each station, respectively. The integrated framework shows promise in enhancing the accuracy of monthly streamflow prediction, thereby aiding in strategic decision-making for water resources management.
更多查看译文
关键词
data-driven models,lagged time analysis,LSTM,monthly streamflow prediction,Yellow River
AI 理解论文
溯源树
样例
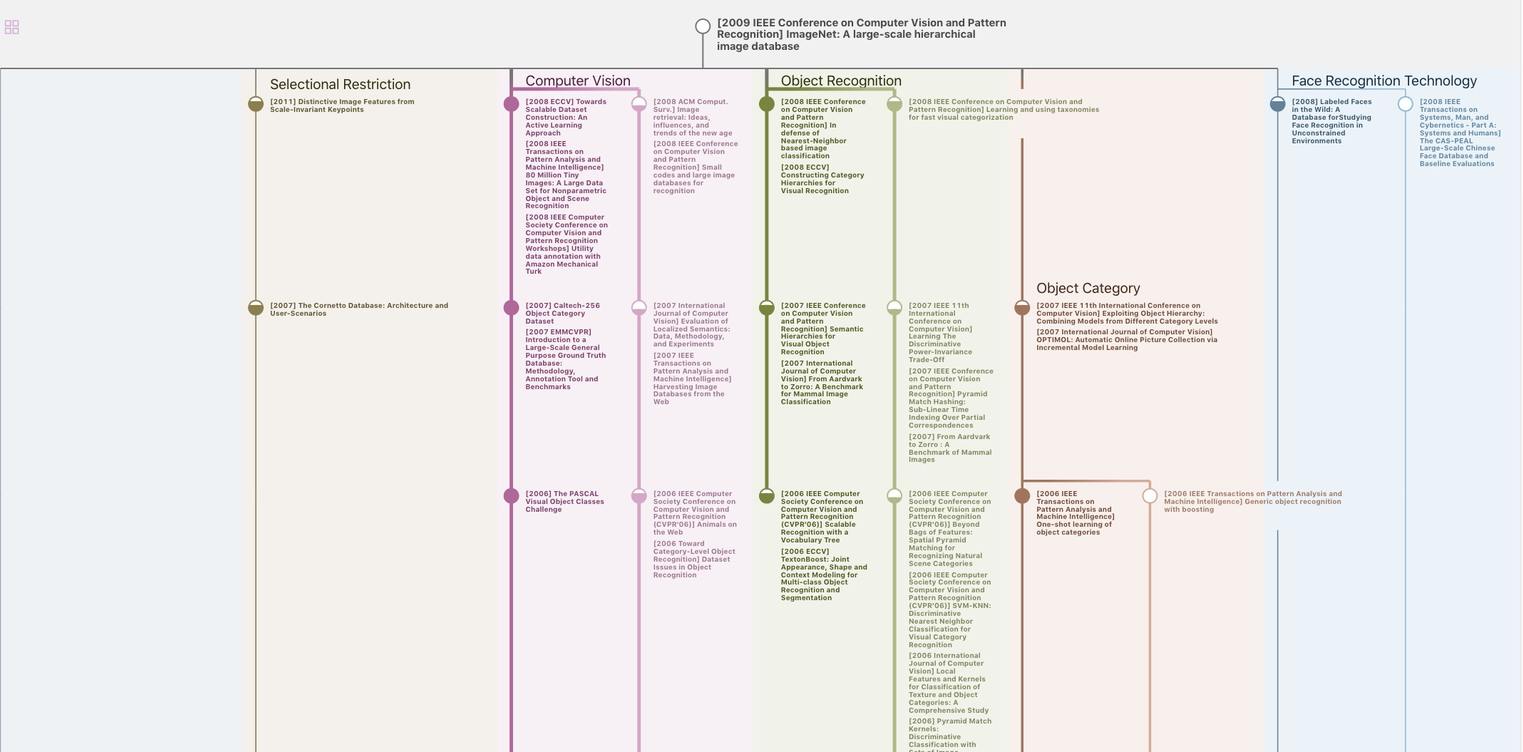
生成溯源树,研究论文发展脉络
Chat Paper
正在生成论文摘要