On the Use of Data-Driven Machine Learning for Probabilistic Fatigue Life Prediction of Metallic Materials Based on Mesoscopic Defect Analysis
Journal of Materials Engineering and Performance(2024)
Abstract
The uncertainty of internal defects is the most important factor that causes the dispersive fatigue life of materials. However, the research about predicting the dispersion of fatigue behavior has not received more attention with the limitation of highly relying on experimental data and empirical parameters. An approach of probabilistic fatigue life prediction was proposed. A multivariate normal distribution model of defects is established based on the microscopic defect analysis of metal specimen. A defect-fatigue database is established through three-dimensional reconstruction of defects, a large number of reconstructed data were used to reproduce the distributed characteristics of fatigue life. A probabilistic model of fatigue life prediction on the use of data-driven machine learning is built. It is verified that proposed model can provide a possibility for predicting the dispersive fatigue life of materials with a little sample data of experiments.
MoreTranslated text
Key words
data-driven machine learning,mesoscopic defect,probabilistic fatigue life,reconstruction
AI Read Science
Must-Reading Tree
Example
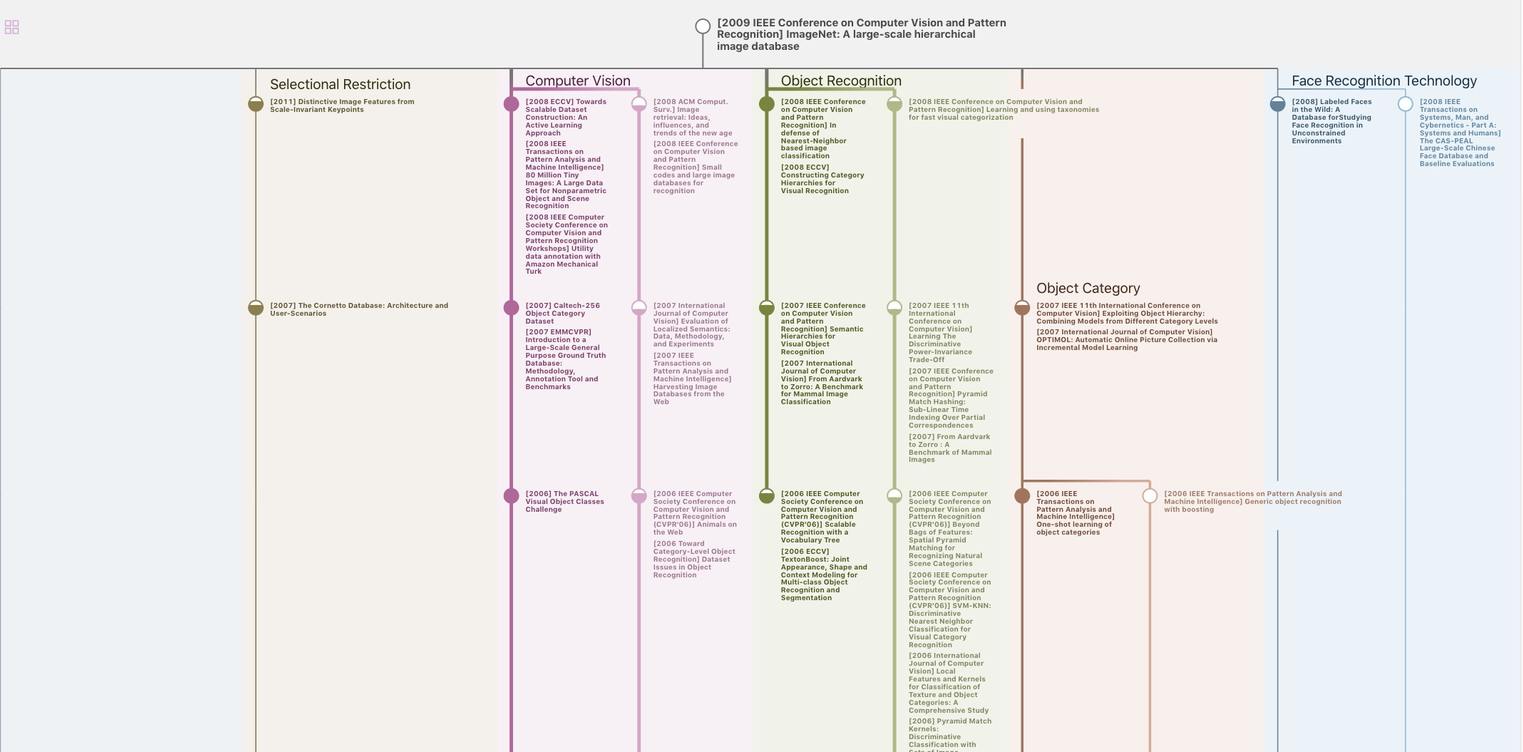
Generate MRT to find the research sequence of this paper
Chat Paper
Summary is being generated by the instructions you defined