Bridging the Temporal Gaps in GRACE/GRACE–FO Terrestrial Water Storage Anomalies over the Major Indian River Basins Using Deep Learning
Natural Resources Research(2024)
Abstract
Temporal gaps in the Gravity Recovery and Climate Experiment (GRACE) and GRACE–FO missions pose difficulties in analyzing spatiotemporal variations of terrestrial water storage (TWS) anomalies over Indian river basins. In this study, we developed a deep learning-based CNN–LSTM model to address these temporal gaps by integrating GRACE–TWS with other meteorological variables. The model achieved a strong Pearson’s correlation coefficient (median of 0.96 in training, 0.92 in testing), high Nash–Sutcliffe efficiency (0.91 in training, 0.85 in testing) and low normalized root-mean-squared error (0.064 in training, 0.098 in testing) when compared to the original GRACE–TWS. Moreover, a comparison with two publicly available global datasets suggests the superior performance of CNN–LSTM in predicting TWSA (terrestrial water storage anomalies). The study also highlighted potential biases up to 2 cm/yr in long-term TWSA trends due to temporal gaps in GRACE/GRACE–FO. Additionally, the estimated TWSA matched well with in situ wells across majority of the Indian river basins. The findings revealed significant groundwater depletion in the northern and northwestern river basins but positive trends in the central and southern basins in India. Overall, the estimated TWSA product developed in this study provides continuous data records and valuable insights into long-term trends in groundwater storage, making it useful for groundwater monitoring.
MoreTranslated text
Key words
GRACE/GRACE–FO,Deep learning,Terrestrial water storage anomalies,Indian river basins
AI Read Science
Must-Reading Tree
Example
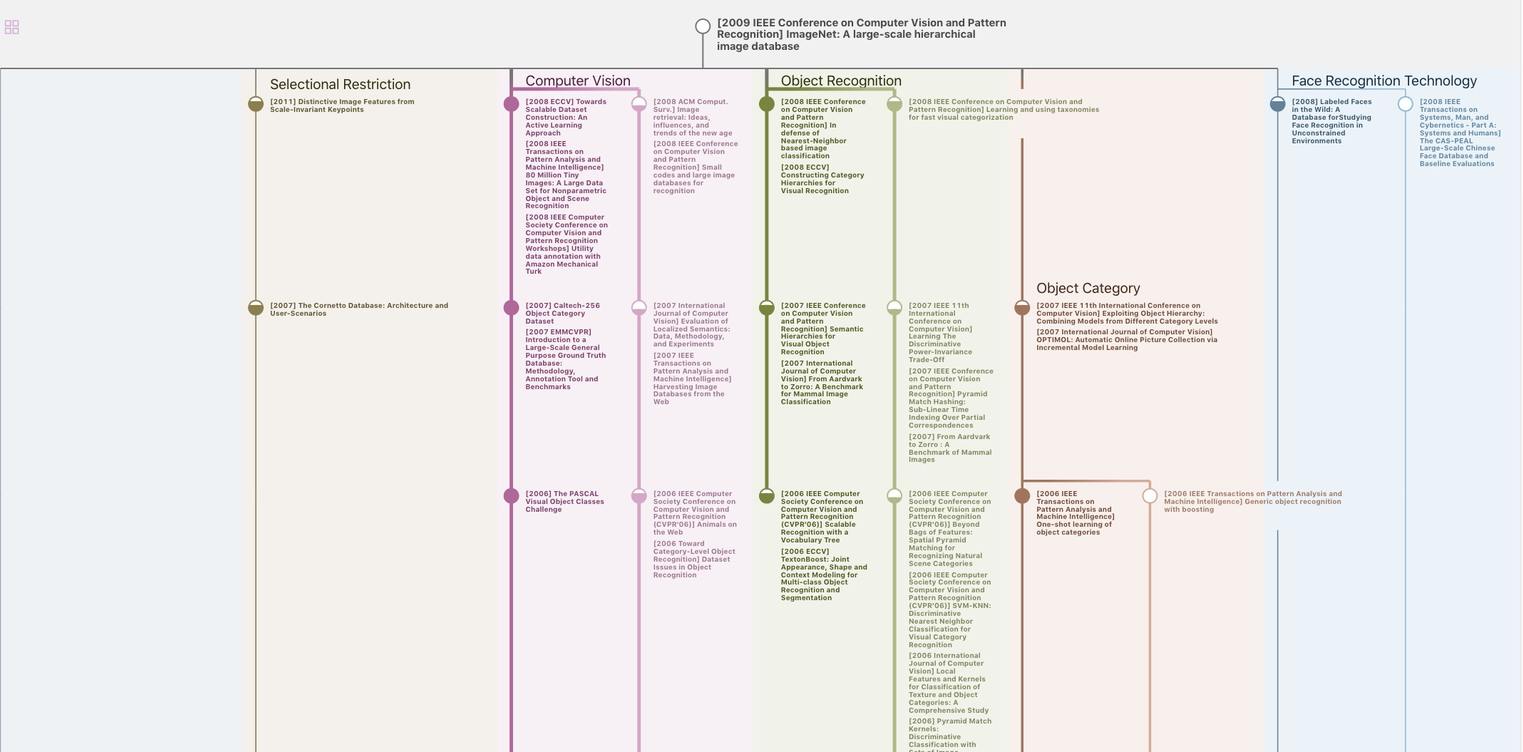
Generate MRT to find the research sequence of this paper
Chat Paper
Summary is being generated by the instructions you defined