DLAN: Modeling user long- and short-term preferences based on double-layer attention network for next point-of-interest recommendation
Journal of Intelligent & Fuzzy Systems: Applications in Engineering and Technology(2024)
摘要
The next Point-of-Interest (POI) recommendation, in recent years, has attracted an extensive amount of attention from the academic community. RNN-based methods cannot establish effective long-term dependencies among the input sequences when capturing the user’s motion patterns, resulting in inadequate exploitation of user preferences. Besides, the majority of prior studies often neglect high-order neighborhood information in users’ check-in trajectory and their social relationships, yielding suboptimal recommendation efficacy. To address these issues, this paper proposes a novel Double-Layer Attention Network model, named DLAN. Firstly, DLAN incorporates a multi-head attention module that can combine first-order and high-order neighborhood information in user check-in trajectories, thereby effectively and parallelly capturing both long- and short-term preferences of users and overcoming the problem that RNN-based methods cannot establish long-term dependencies between sequences. Secondly, this paper designs a user similarity weighting layer to measure the influence of other users on the target users leverage the social relationships among them. Finally, comprehensive experiments are conducted on user check-in data from two cities, New York (NYC) and Tokyo (TKY), and the results demonstrate that DLAN achieves a performance in Accuracy and Mean Reverse Rank enhancement by 8.07% -36.67% compared to the state-of-the-art method. Moreover, to investigate the effect of dimensionality and the number of heads of the multi-head attention mechanism on the performance of the DLAN model, we have done sufficient sensitivity experiments.
更多查看译文
关键词
Point-of-interest recommendation,user preferences,attention network,social information
AI 理解论文
溯源树
样例
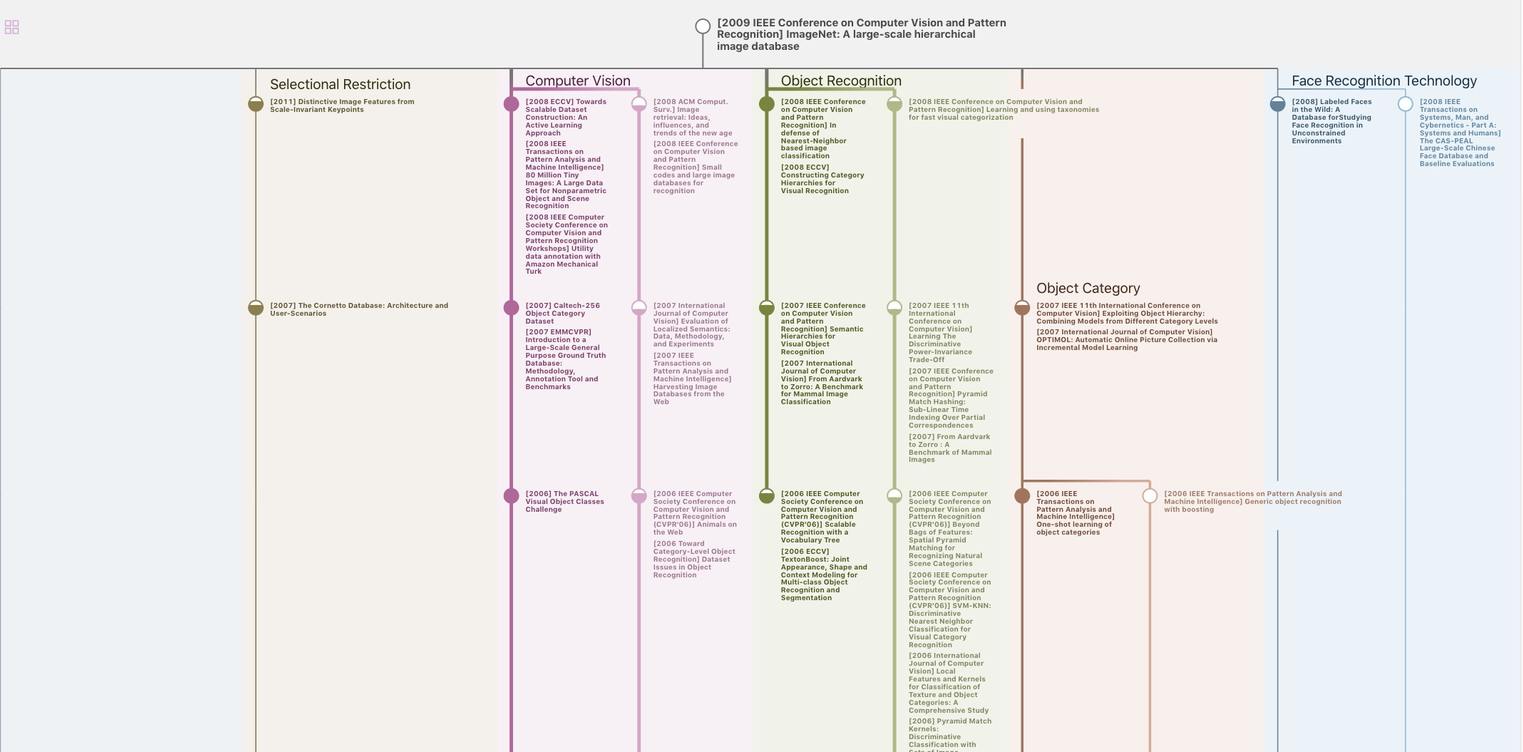
生成溯源树,研究论文发展脉络
Chat Paper
正在生成论文摘要