Recurrent neural networks as neuro-computational models of human speech recognition
biorxiv(2024)
摘要
Human speech recognition transforms a continuous acoustic signal into categorical linguistic units, by aggregating information that is distributed in time. It has been suggested that this kind of information processing may be understood through the computations of a Recurrent Neural Network (RNN) that receives input frame by frame, linearly in time, but builds an incremental representation of this input through a continually evolving internal state. While RNNs can simulate several key behavioral observations about human speech and language processing, it is unknown whether RNNs also develop computational dynamics that resemble human neural speech processing . Here we show that the internal dynamics of long short-term memory (LSTM) RNNs, trained to recognize speech from auditory spectrograms, predict human neural population responses to the same stimuli, beyond predictions from auditory features. Variations in the RNN architecture motivated by cognitive principles further improve this predictive power. Moreover, different components of hierarchical RNNs predict separable components of brain responses to speech in an anatomically structured manner, suggesting that RNNs reproduce a hierarchy of speech recognition in the brain. Our results suggest that RNNs provide plausible computational models of the cortical processes supporting human speech recognition.
### Competing Interest Statement
The authors have declared no competing interest.
更多查看译文
AI 理解论文
溯源树
样例
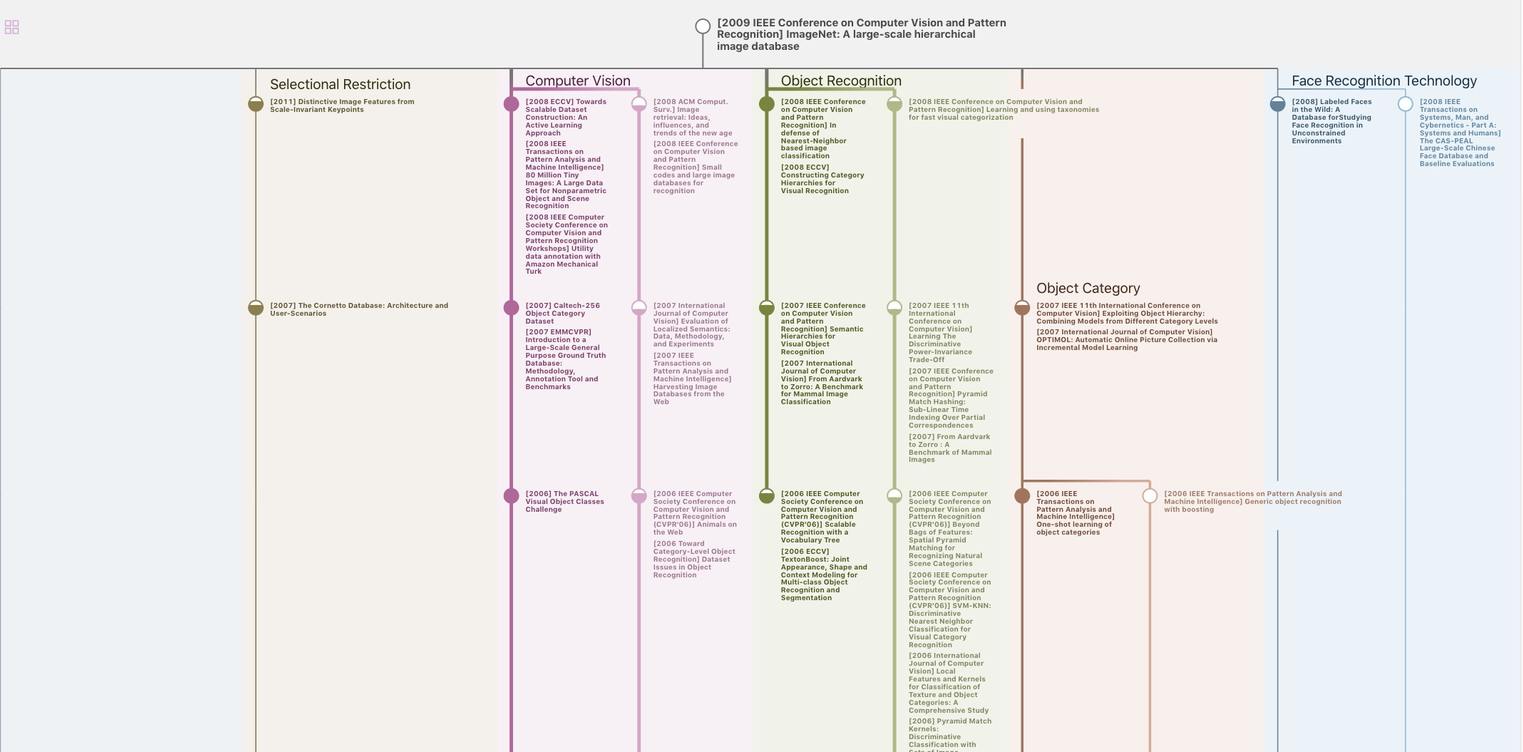
生成溯源树,研究论文发展脉络
Chat Paper
正在生成论文摘要