Physically Meaningful Surrogate Data for COPD
IEEE OPEN JOURNAL OF ENGINEERING IN MEDICINE AND BIOLOGY(2024)
摘要
The rapidly increasing prevalence of debilitating breathing disorders, such as chronic obstructive pulmonary disease (COPD), calls for a meaningful integration of artificial intelligence (AI) into respiratory healthcare. Deep learning techniques are "data hungry" whilst patient-based data is invariably expensive and time consuming to record. To this end, we introduce a novel COPD-simulator, a physical apparatus with an easy to replicate design which enables rapid and effective generation of a wide range of COPD-like data from healthy subjects, for enhanced training of deep learning frameworks. To ensure the faithfulness of our domain-aware COPD surrogates, the generated waveforms are examined through both flow waveforms and photoplethysmography (PPG) waveforms (as a proxy for intrathoracic pressure) in terms of duty cycle, sample entropy, FEV1/FVC ratios and flow-volume loops. The proposed simulator operates on healthy subjects and is able to generate FEV1/FVC obstruction ratios ranging from greater than 0.8 to less than 0.2, mirroring values that can observed in the full spectrum of real-world COPD. As a final stage of verification, a simple convolutional neural network is trained on surrogate data alone, and is used to accurately detect COPD in real-world patients. When training solely on surrogate data, and testing on real-world data, a comparison of true positive rate against false positive rate yields an area under the curve of 0.75, compared with 0.63 when training solely on real-world data.
更多查看译文
关键词
Electron tubes,Lung,Chronic obstructive pulmonary disease,Valves,Plugs,Resistance,Training,COPD,deep learning,photoplethysmography,surrogate data,wearable health
AI 理解论文
溯源树
样例
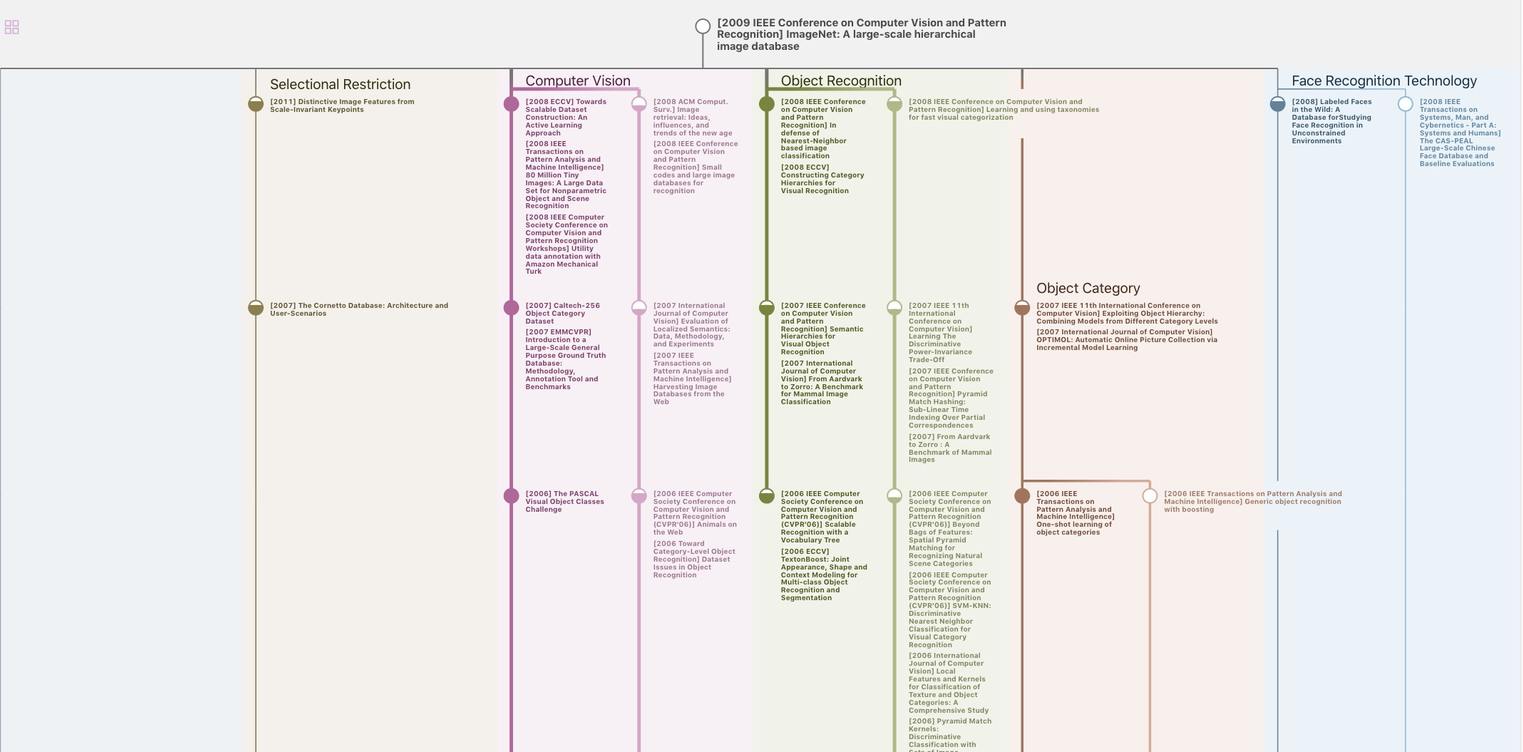
生成溯源树,研究论文发展脉络
Chat Paper
正在生成论文摘要