DynGMA: a robust approach for learning stochastic differential equations from data
Journal of Computational Physics(2024)
摘要
Learning unknown stochastic differential equations (SDEs) from observed data is a significant and challenging task with applications in various fields. Current approaches often use neural networks to represent drift and diffusion functions, and construct likelihood-based loss by approximating the transition density to train these networks. However, these methods often rely on one-step stochastic numerical schemes, necessitating data with sufficiently high time resolution. In this paper, we introduce novel approximations to the transition density of the parameterized SDE: a Gaussian density approximation inspired by the random perturbation theory of dynamical systems, and its extension, the dynamical Gaussian mixture approximation (DynGMA). Benefiting from the robust density approximation, our method exhibits superior accuracy compared to baseline methods in learning the fully unknown drift and diffusion functions and computing the invariant distribution from trajectory data. And it is capable of handling trajectory data with low time resolution and variable, even uncontrollable, time step sizes, such as data generated from Gillespie's stochastic simulations. We then conduct several experiments across various scenarios to verify the advantages and robustness of the proposed method.
更多查看译文
关键词
Neural networks,Learning dynamics,Maximum likelihood estimation,Invariant distribution
AI 理解论文
溯源树
样例
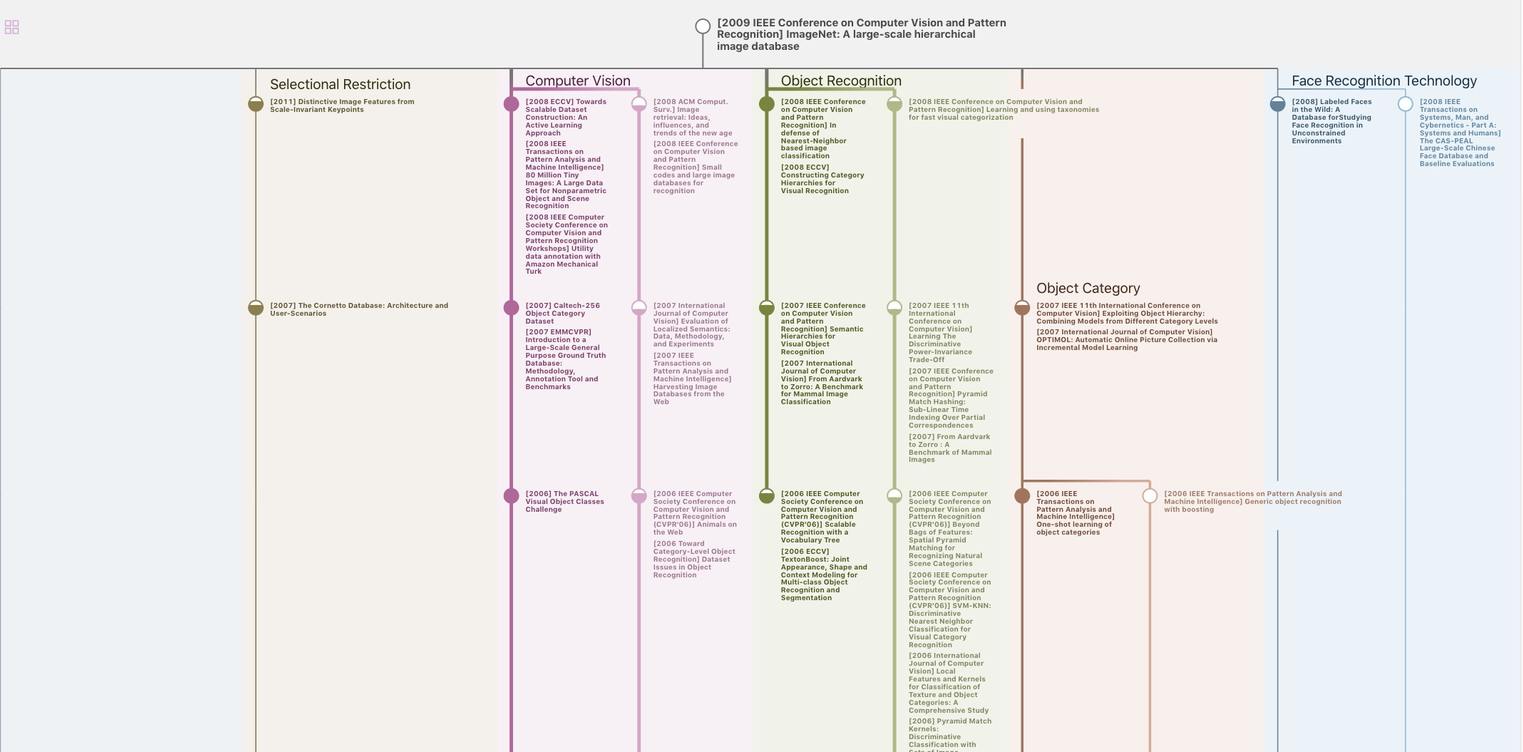
生成溯源树,研究论文发展脉络
Chat Paper
正在生成论文摘要