PolyNet: Learning Diverse Solution Strategies for Neural Combinatorial Optimization
CoRR(2024)
Abstract
Reinforcement learning-based methods for constructing solutions to
combinatorial optimization problems are rapidly approaching the performance of
human-designed algorithms. To further narrow the gap, learning-based approaches
must efficiently explore the solution space during the search process. Recent
approaches artificially increase exploration by enforcing diverse solution
generation through handcrafted rules, however, these rules can impair solution
quality and are difficult to design for more complex problems. In this paper,
we introduce PolyNet, an approach for improving exploration of the solution
space by learning complementary solution strategies. In contrast to other
works, PolyNet uses only a single-decoder and a training schema that does not
enforce diverse solution generation through handcrafted rules. We evaluate
PolyNet on four combinatorial optimization problems and observe that the
implicit diversity mechanism allows PolyNet to find better solutions than
approaches the explicitly enforce diverse solution generation.
MoreTranslated text
AI Read Science
Must-Reading Tree
Example
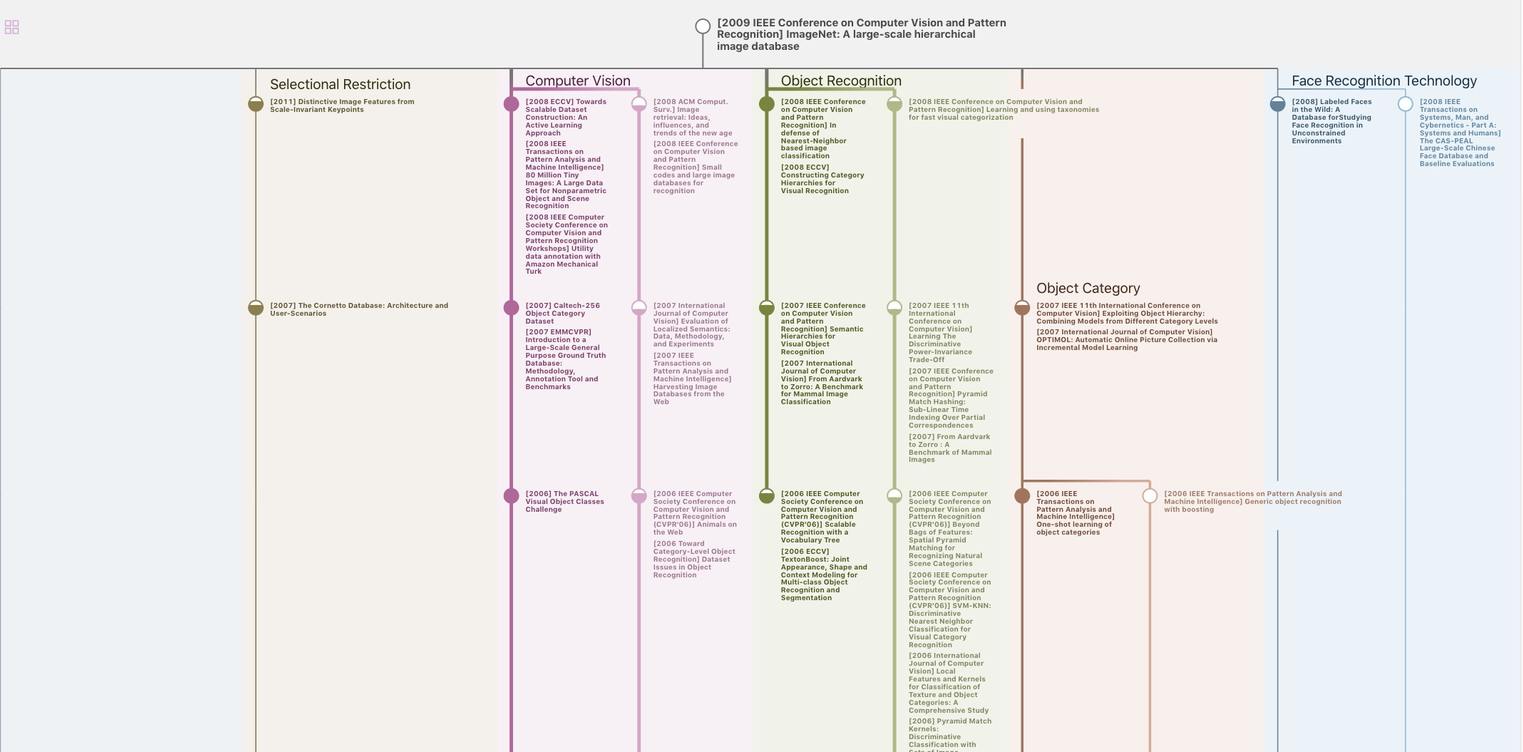
Generate MRT to find the research sequence of this paper
Chat Paper
Summary is being generated by the instructions you defined