CTMANet: A CNN-Transformer Hybrid Semantic Segmentation Network for Fine-Grained Airport Extraction in Complex SAR Scenes
IEEE JOURNAL OF SELECTED TOPICS IN APPLIED EARTH OBSERVATIONS AND REMOTE SENSING(2024)
摘要
Airports represent essential infrastructure, offering substantial research and application potential. However, extracting airports from complex synthetic aperture radar (SAR) scenes is challenging due to the cluttered background and fine structure of airports. This necessitates the integration of global and local information for fine-grained extraction. To tackle this issue, this article introduces a novel framework for fine-grained extraction of airports from large-scale SAR images. First, a convolutional neural networks (CNN) transformer hybrid semantic segmentation network with multiscale contextual fusion is proposed, named CNN-transformer network (CTMANet). In this network, the encoder combines CNNs and transformers to capture local and global information, while the multiscale context aggregation block fuses multiscale contextual information. Skip connections between the encoder and decoder are established to minimize the loss of detailed information and fuse low-level features with high-level semantic features. Moreover, a category balance block is designed to address class imbalance. Experimental results on the GF-3 dataset demonstrate that CTMANet outperforms state-of-the-art methods, proving its superior suitability for fine-grained airport extraction in large-scale scenarios.
更多查看译文
关键词
Airports,Feature extraction,Transformers,Radar polarimetry,Data mining,Synthetic aperture radar,Semantics,Convolutional neural networks (CNN) transformer hybrid,fine-grained airport extraction,multiscale context fusion,semantic segmentation
AI 理解论文
溯源树
样例
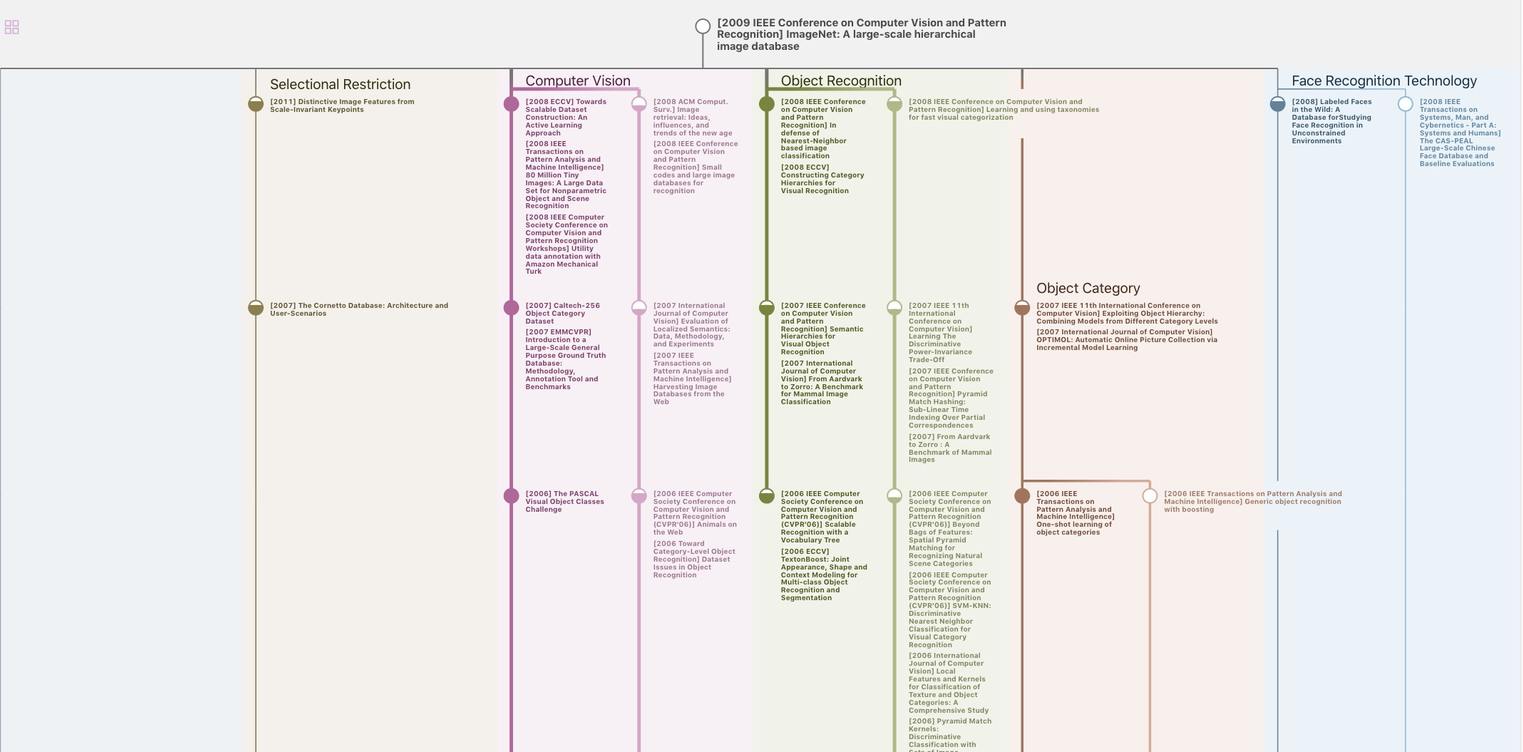
生成溯源树,研究论文发展脉络
Chat Paper
正在生成论文摘要