Hand Motor Imagery Identification Using Machine Learning Approaches in a Protocol Based on Visual Stimuli and Passive Movement
2023 IEEE Colombian Caribbean Conference (C3)(2023)
摘要
In recent years, advancements in rehabilitation techniques have been made to leverage biosignals and passive movement as integral components of physical interventions to restore upper limb mobility in people with disabilities. This study introduces a novel Motor Imagery (MI) protocol based on Action Observation (AO) using static visual cues and a robotic glove used in the right hand. To address this, Machine Learning (ML) techniques were assessed using features based on Power Spectral Density (PSD) in the mu (μ, 8–13 Hz) and beta (β, 13–30 Hz) frequency bands. Four distinct classifiers were explored: Kernel Naive Bayes (NB), Quadratic Support Vector Machine (QSVM), Fine k-nearest neighbors (KNN), and Logistic Regression (LR). These classifiers were employed to discriminate between open- and closed-hand MI tasks. Based on the evaluated metrics, it was concluded that the implemented methodology is feasible for classifying MI tasks from the same limb, where the QSVM classifier showed the most promising results, with a mean accuracy of approximately 75%. Future work will focus on implementing the system to control the robotic glove in the context of post-stroke rehabilitation, underscoring its potential clinical utility.
更多查看译文
关键词
EEG,Robotic Glove,Hand Motor Imagery,Upper-limb rehabilitation,Action Observation
AI 理解论文
溯源树
样例
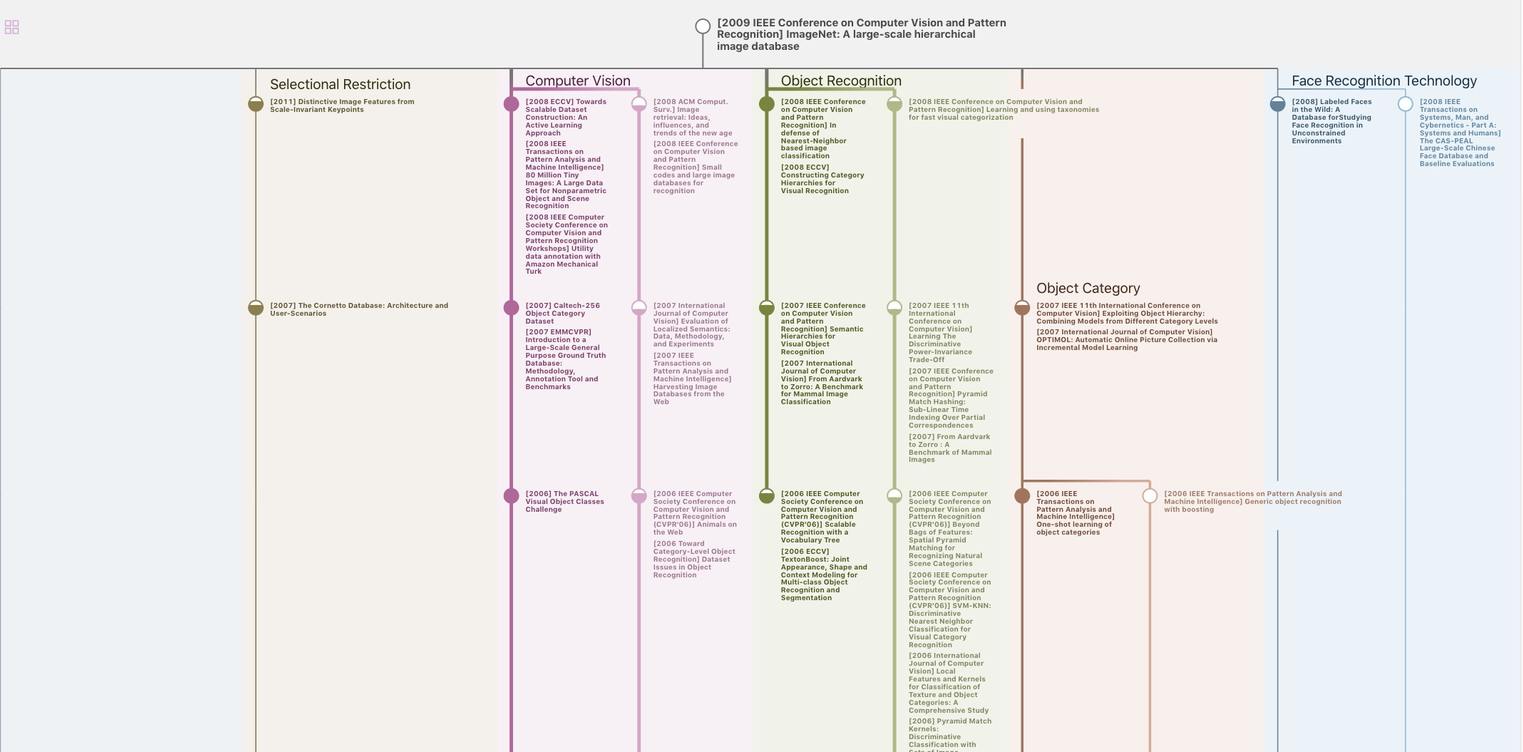
生成溯源树,研究论文发展脉络
Chat Paper
正在生成论文摘要