Local convergence analysis of an inexact trust-region method for nonsmooth optimization
Optimization Letters(2024)
摘要
In Baraldi (Math Program 20:1–40, 2022), we introduced an inexact trust-region algorithm for minimizing the sum of a smooth nonconvex function and a nonsmooth convex function in Hilbert space—a class of problems that is ubiquitous in data science, learning, optimal control, and inverse problems. This algorithm has demonstrated excellent performance and scalability with problem size. In this paper, we enrich the convergence analysis for this algorithm, proving strong convergence of the iterates with guaranteed rates. In particular, we demonstrate that the trust-region algorithm recovers superlinear, even quadratic, convergence rates when using a second-order Taylor approximation of the smooth objective function term.
更多查看译文
关键词
Nonsmooth optimization,Nonconvex optimization,Trust region,Newton’s method,Superlinear convergence,Quadratic convergence
AI 理解论文
溯源树
样例
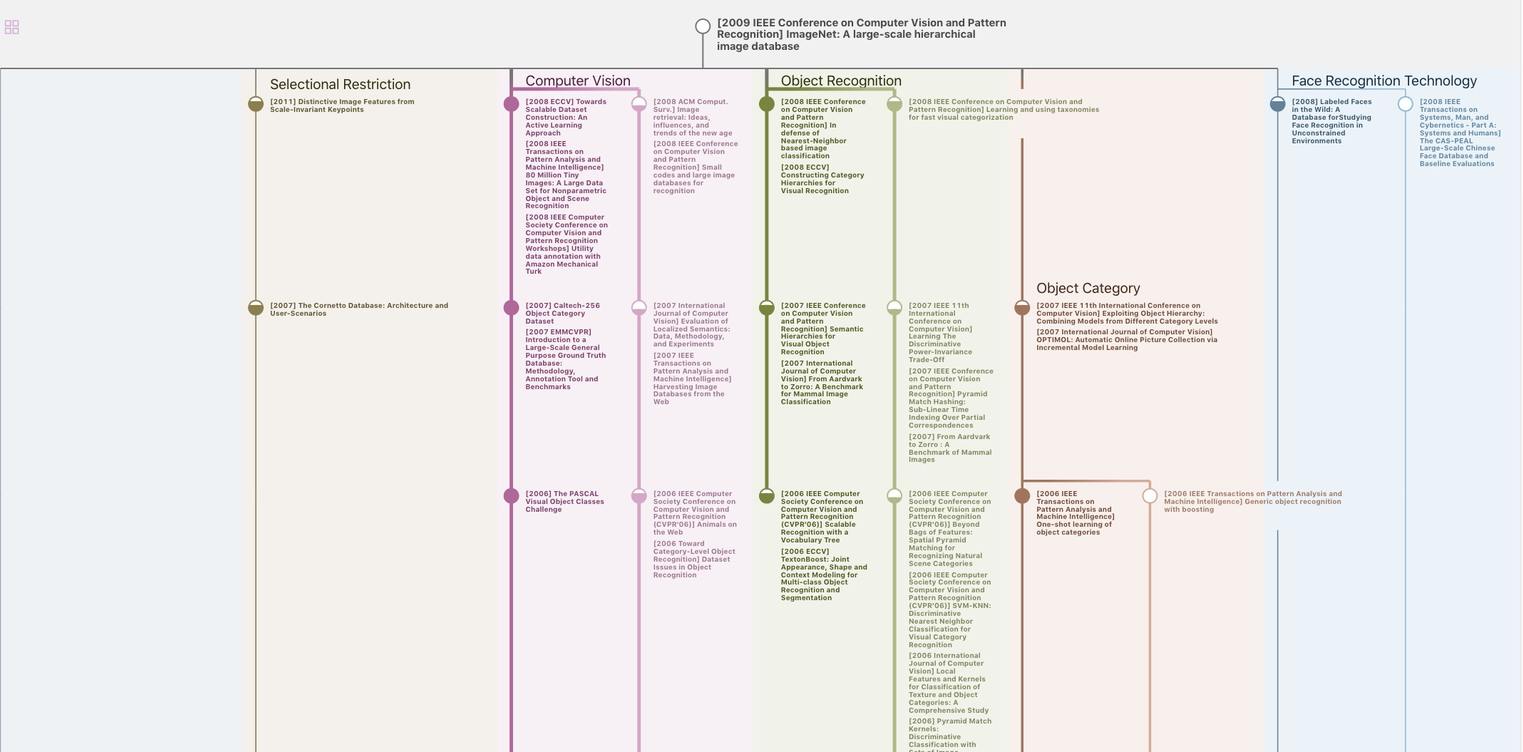
生成溯源树,研究论文发展脉络
Chat Paper
正在生成论文摘要