Stochastic Analysis of Fog Computing and Machine Learning for Scalable Low-Latency Healthcare Monitoring
CLUSTER COMPUTING-THE JOURNAL OF NETWORKS SOFTWARE TOOLS AND APPLICATIONS(2024)
Key words
Fog computing,Machine learning,Healthcare monitoring,Queuing model,Performance analysis
AI Read Science
Must-Reading Tree
Example
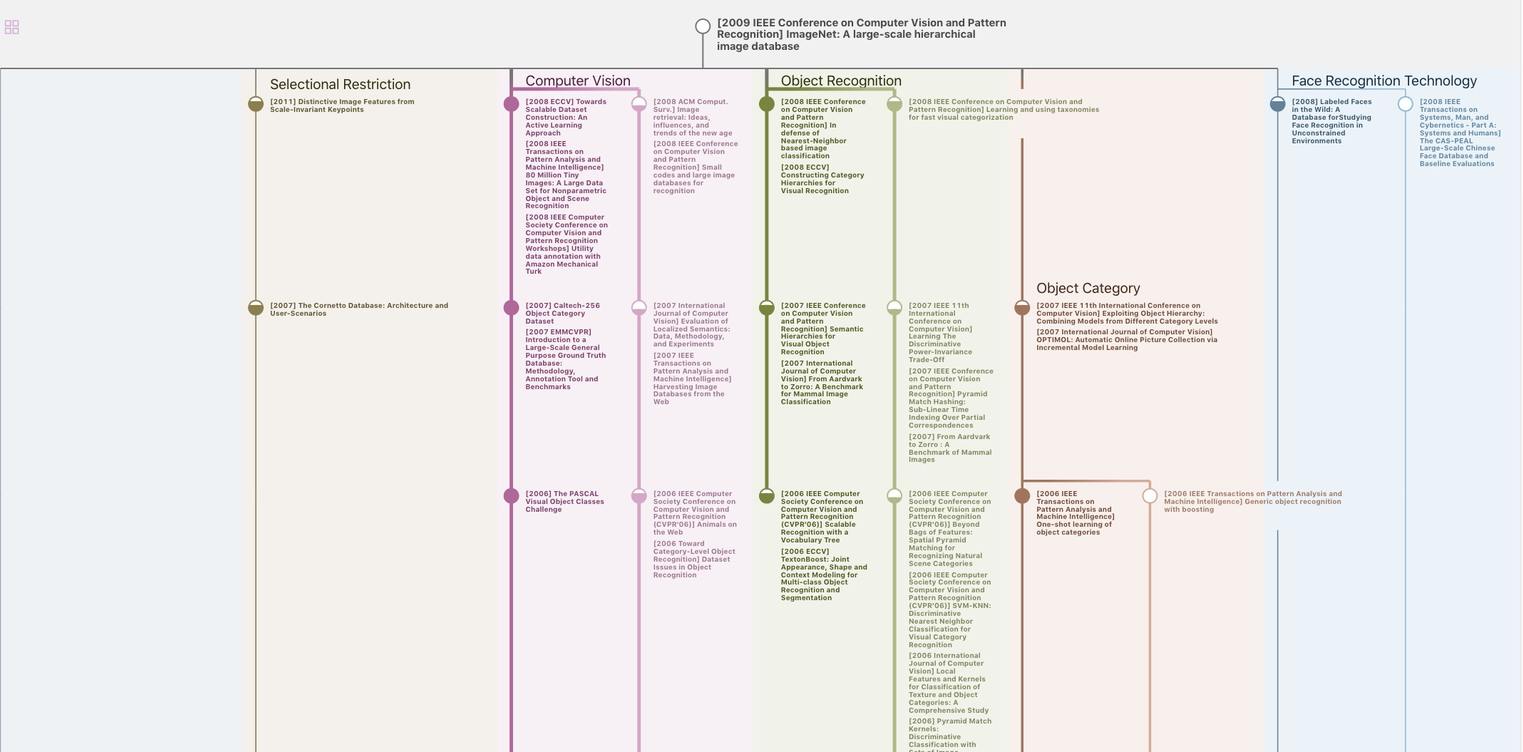
Generate MRT to find the research sequence of this paper
Chat Paper
Summary is being generated by the instructions you defined