ISSLIDE: A New InSAR Dataset for Slow SLIding Area DEtection with Machine Learning
IEEE geoscience and remote sensing letters(2024)
Abstract
Due to the high data demand of machine-learning algorithms, multiple datasets are emerging in remote sensing. However, these datasets are costly and time-consuming to annotate especially for change detection or natural phenomena monitoring. In particular, early warning systems for slow-moving disasters lack training datasets as they require both geomorphological and SAR interferometry expertise. In this letter: 1) we propose a novel interferometric SAR (InSAR) dataset for Slow SLIding area DEtection (ISSLIDE) with machine-learning algorithms. The latter consists of manually annotated patches of generated interferograms over slow-moving areas; and 2) we implement the segmentation of ISSLIDE interferograms with classical deep-learning approaches. FCN, DeepLabV3, and U-Net-like architectures are explored to serve as a baseline for future works. To the best of our knowledge, this is the first dataset adapted to machine learning and targeting slow sliding area detection.
MoreTranslated text
Key words
Annotations,Terrain factors,Coherence,Training,Rocks,Delays,Optical imaging,Dataset,deep learning,ground motions,InSAR dataset for Slow SLIding area DEtection (ISSLIDE),interferometric SAR (InSAR)
AI Read Science
Must-Reading Tree
Example
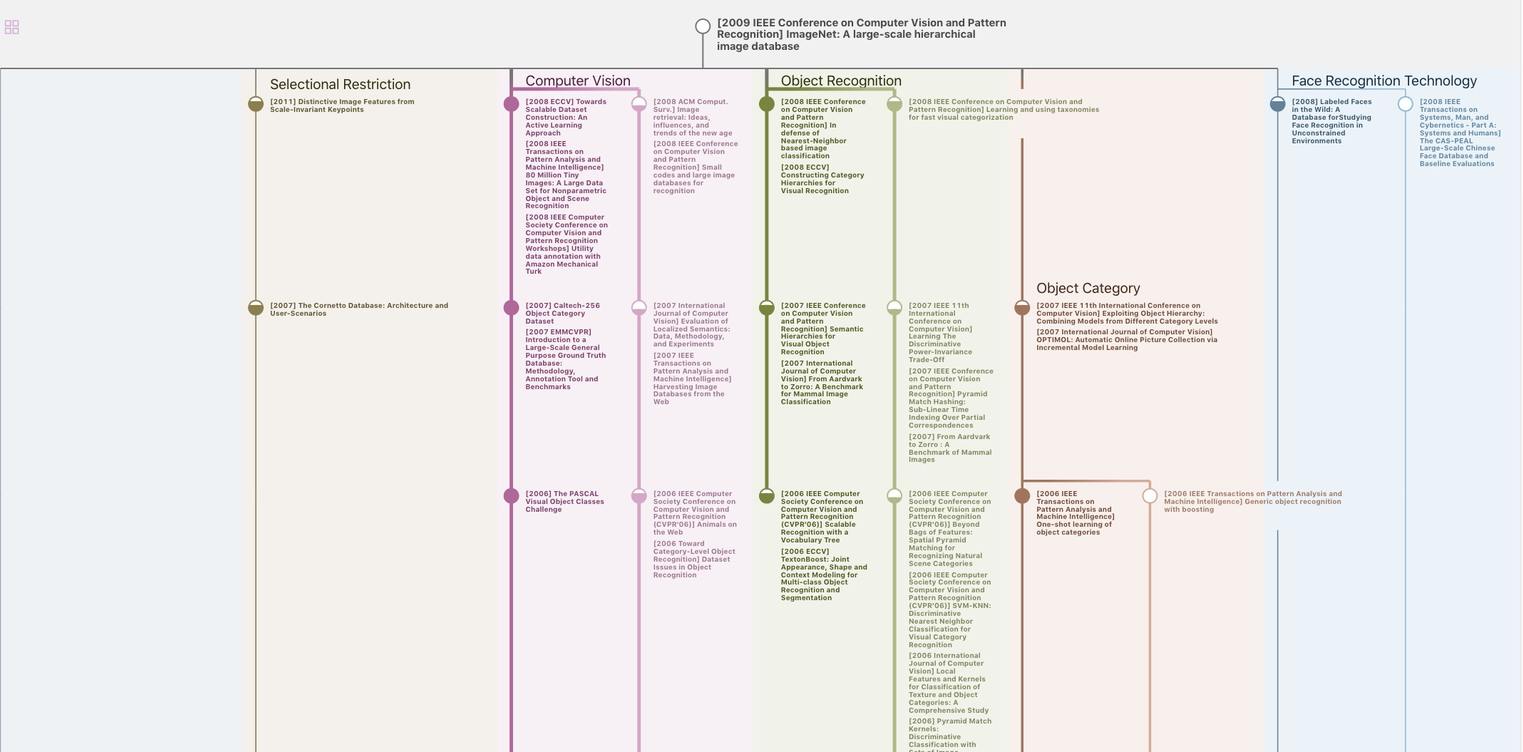
Generate MRT to find the research sequence of this paper
Chat Paper
Summary is being generated by the instructions you defined