Statistically Guided Near-End Speech Intelligibility Improvement Through Voice Transformation and Transfer Learning
IEEE-ACM TRANSACTIONS ON AUDIO SPEECH AND LANGUAGE PROCESSING(2024)
摘要
In recent developments, speech intelligibility has been improved through an optimal trapezoidal transformation function, which performed normal to Lombard speech conversion via formant shifting. Despite performing well, the optimization took very long to converge and led to artifacts in the modified signal due to aggressive formant shifts in unvoiced frames. Therefore, transfer learning was used to rapidly modify the optimized parameters for a target language to bypass re-optimization for a new language. However, such transfer across noises was left unaddressed. This work proposes a Gaussian transformation function to perform statistically guided normal to Lombard speech conversion. Optimizing fewer parameters ensures faster convergence than before. The new transformation function generates fewer artifacts during voice modification while performing at par with the earlier function. This work enhances transfer learning performance by mitigating the directional nature in case of language mismatch. We also propose the transfer learning across noises using the comparative estimations of noise magnitude spectra, which was not feasible earlier. The simultaneous transfer of parameters across languages and noises is now feasible via the proposed Gaussian transformation function. We also explore the statistical difference between formant shifts produced by the Gaussian transformation function and its predecessor and their effect on intelligibility improvement. All experiments were conducted on exhaustive combinations of three languages, four noise types, and three SNR levels.
更多查看译文
关键词
Speech processing,Transfer learning,Optimization,Signal to noise ratio,Noise measurement,Speech enhancement,Speech synthesis,CLPSO,formant shifting,Gaussian transformation,near-end intelligibility,STOI,transfer learning
AI 理解论文
溯源树
样例
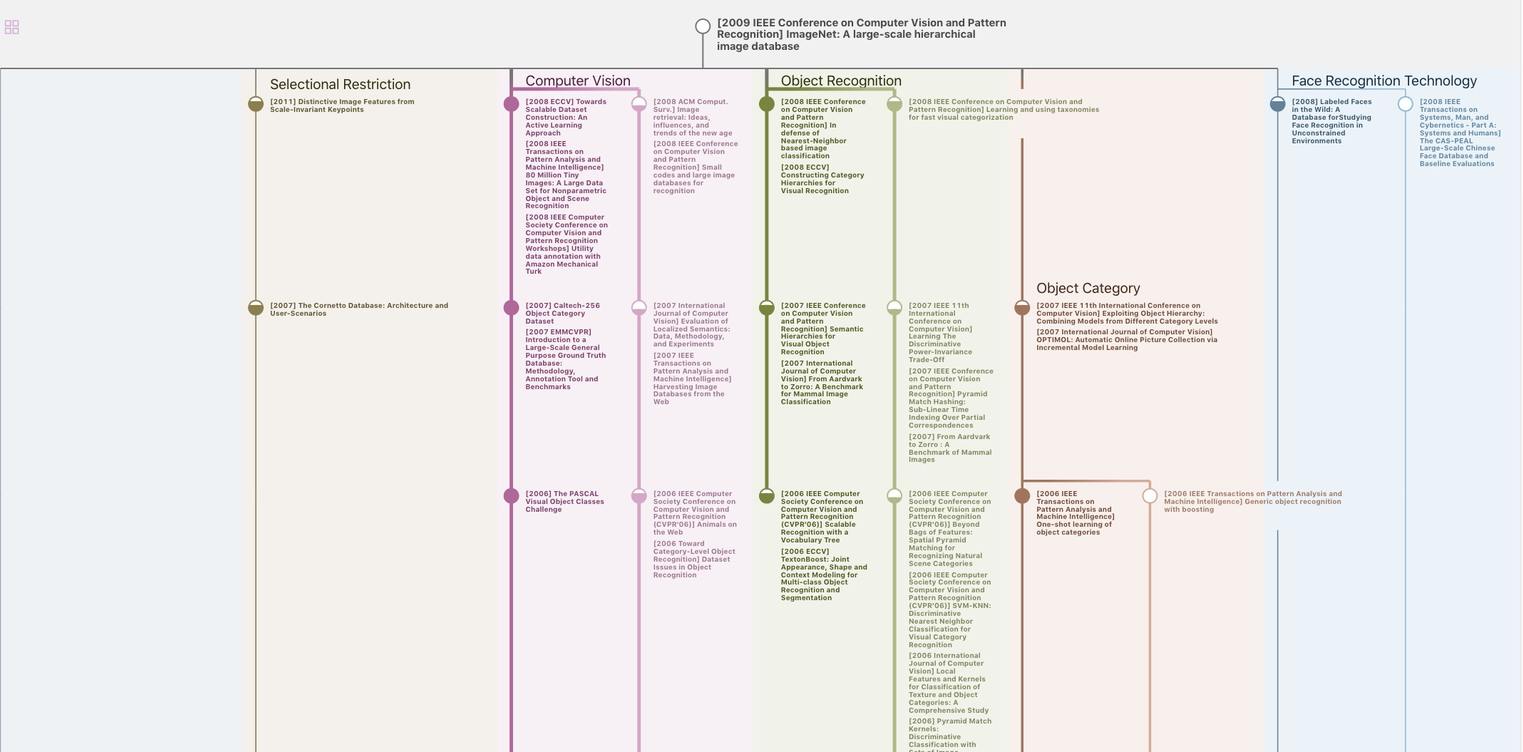
生成溯源树,研究论文发展脉络
Chat Paper
正在生成论文摘要