Ensemble automated approaches for producing high quality herbarium digital records
biorxiv(2024)
摘要
One of the slowest steps in digitizing natural history collections is converting labels associated with specimens into a digital data record usable for collections management and research. Recent work has shown a path for semi-automated approaches that can find labels, OCR them and convert the raw OCR text into digital data records. Here we address how raw OCR can be converted into a digital data record via extraction into standardized Darwin Core fields. We first showcase development of a rule-based approach and compare outcomes with a large language model-based approach, in particular ChatGPT4. We next quantified error rates in a set of OCRed labels, determining omission and commission errors for both approaches and documenting other issues. For example, we find that ChatGPT4 will often create field names that are not Darwin Core compliant. Our results suggest that these approaches each have different limitations. Therefore, we developed an ensemble approach that utilizes outputs from both in order to reduce problems from each individual method. An ensemble method reduces issues with field name heterogeneity and strongly reduces information extraction errors. This suggests that such an ensemble method is likely to have particular value for creating digital data records, even for complicated label content, given that longer labels, though more error prone, are still successfully extracted. While human validation is still much needed to ensure the best possible quality, we showcase working solutions to speed digitization of herbarium specimen labels that are likely usable more broadly for all natural history collection types.
### Competing Interest Statement
The authors have declared no competing interest.
更多查看译文
AI 理解论文
溯源树
样例
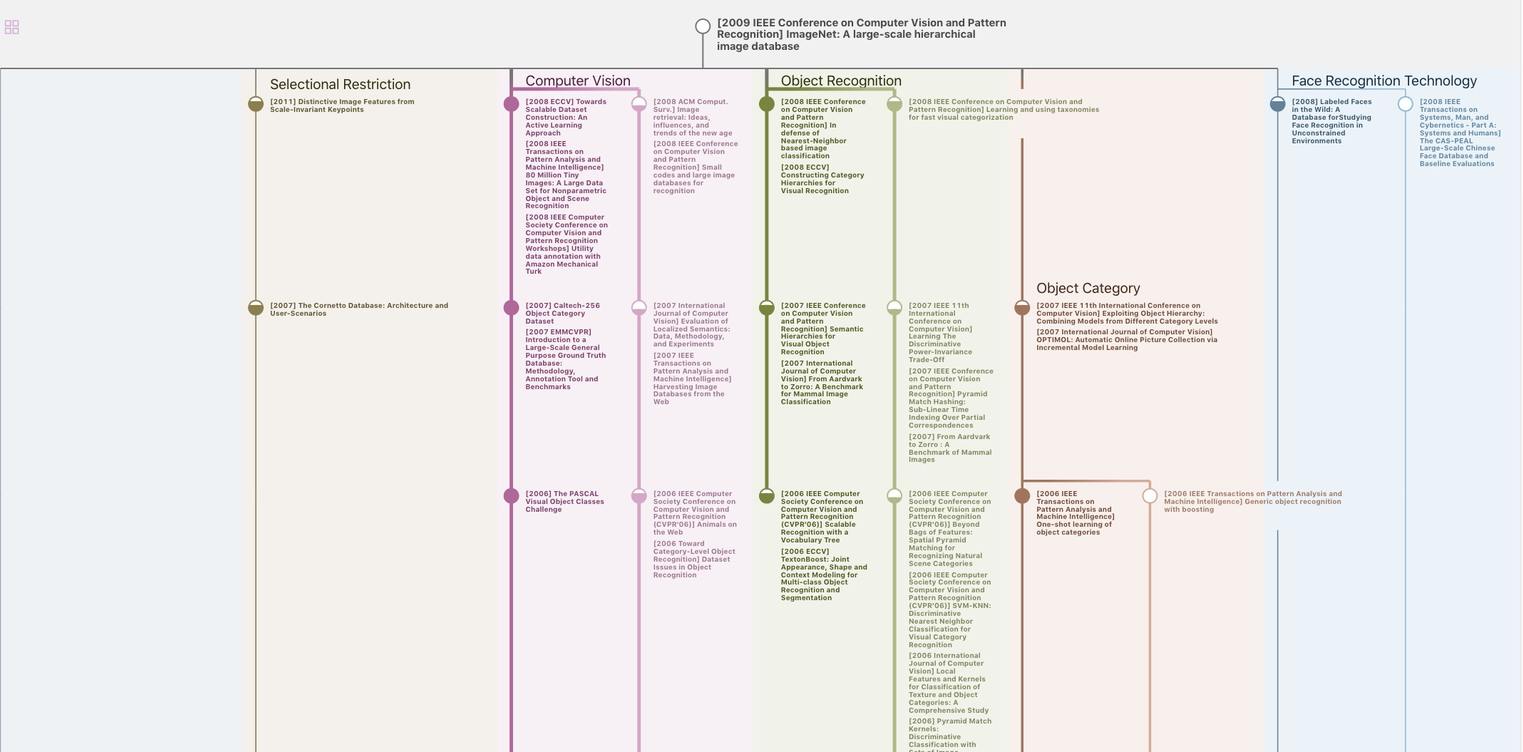
生成溯源树,研究论文发展脉络
Chat Paper
正在生成论文摘要