Breast Cancer Histopathology Images Classification Through Multi-View Augmented Contrastive Learning and Pre-Learning Knowledge Distillation
IEEE ACCESS(2024)
Abstract
Breast cancer is one of the most common malignant tumors in women, and accurate classification of benign and malignant breast cancer histopathology images is critical for diagnosis and treatment. Knowledge distillation techniques have recently received considerable attention for enabling cost-effective image classification of breast cancer histopathology images. However, there are still challenges, including the inefficient extraction of global and local features from breast cancer histopathology images by the teacher network, and the over-prioritization of feature fusion within the same encoder layer, which tends to neglect valuable information from adjacent encoder layers during knowledge extraction. To address these challenges, we propose a method that combines multi-view augmented contrastive learning and pre-learning knowledge distillation. Multi-view augmented contrastive learning improves the ability of the teacher network to extract global and local features. Pre-learning knowledge distillation merges features from adjacent encoder layers of the teacher network, reducing feature loss between these layers. Empirical experiments demonstrate the effectiveness of our proposed method in classifying histopathology images of benign and malignant breast cancer, achieving excellent results on the BreakHis dataset. These results confirm our method as an effective tool for the classification of benign and malignant breast cancer histopathology images.
MoreTranslated text
Key words
Breast cancer,Histopathology,Feature extraction,Self-supervised learning,Computational modeling,Knowledge transfer,Knowledge representation,Knowledge discovery,Contrast resolution,Image classification,Biomedical imaging,Knowledge distillation,contrastive learning,breast cancer histopathology image,global feature,local feature
AI Read Science
Must-Reading Tree
Example
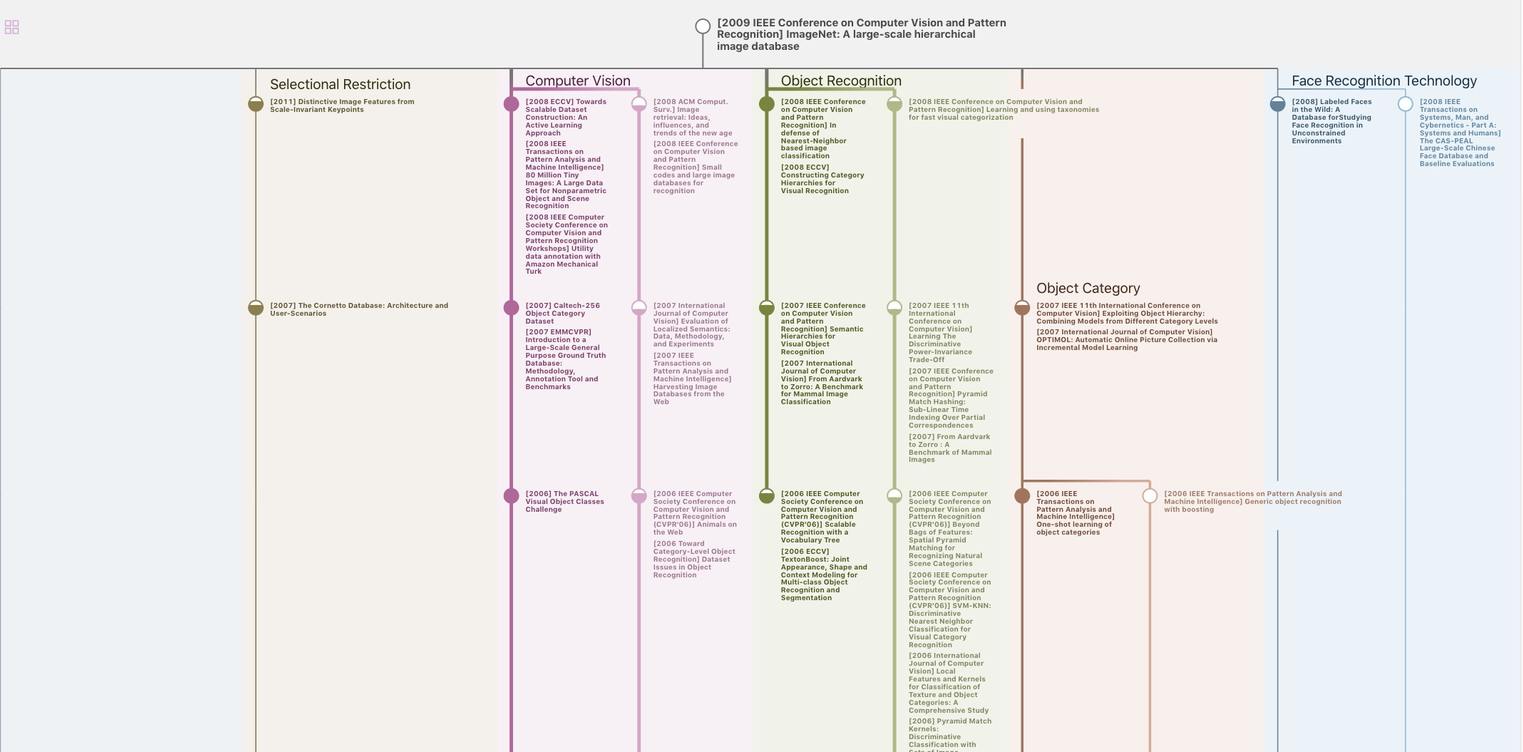
Generate MRT to find the research sequence of this paper
Chat Paper
Summary is being generated by the instructions you defined