Breaking the Barrier: Utilizing Large Language Models for Industrial Recommendation Systems through an Inferential Knowledge Graph
CoRR(2024)
摘要
Recommendation systems are widely used in e-commerce websites and online
platforms to address information overload. However, existing systems primarily
rely on historical data and user feedback, making it difficult to capture user
intent transitions. Recently, Knowledge Base (KB)-based models are proposed to
incorporate expert knowledge, but it struggle to adapt to new items and the
evolving e-commerce environment. To address these challenges, we propose a
novel Large Language Model based Complementary Knowledge Enhanced
Recommendation System (LLM-KERec). It introduces an entity extractor that
extracts unified concept terms from item and user information. To provide
cost-effective and reliable prior knowledge, entity pairs are generated based
on entity popularity and specific strategies. The large language model
determines complementary relationships in each entity pair, constructing a
complementary knowledge graph. Furthermore, a new complementary recall module
and an Entity-Entity-Item (E-E-I) weight decision model refine the scoring of
the ranking model using real complementary exposure-click samples. Extensive
experiments conducted on three industry datasets demonstrate the significant
performance improvement of our model compared to existing approaches.
Additionally, detailed analysis shows that LLM-KERec enhances users' enthusiasm
for consumption by recommending complementary items. In summary, LLM-KERec
addresses the limitations of traditional recommendation systems by
incorporating complementary knowledge and utilizing a large language model to
capture user intent transitions, adapt to new items, and enhance recommendation
efficiency in the evolving e-commerce landscape.
更多查看译文
AI 理解论文
溯源树
样例
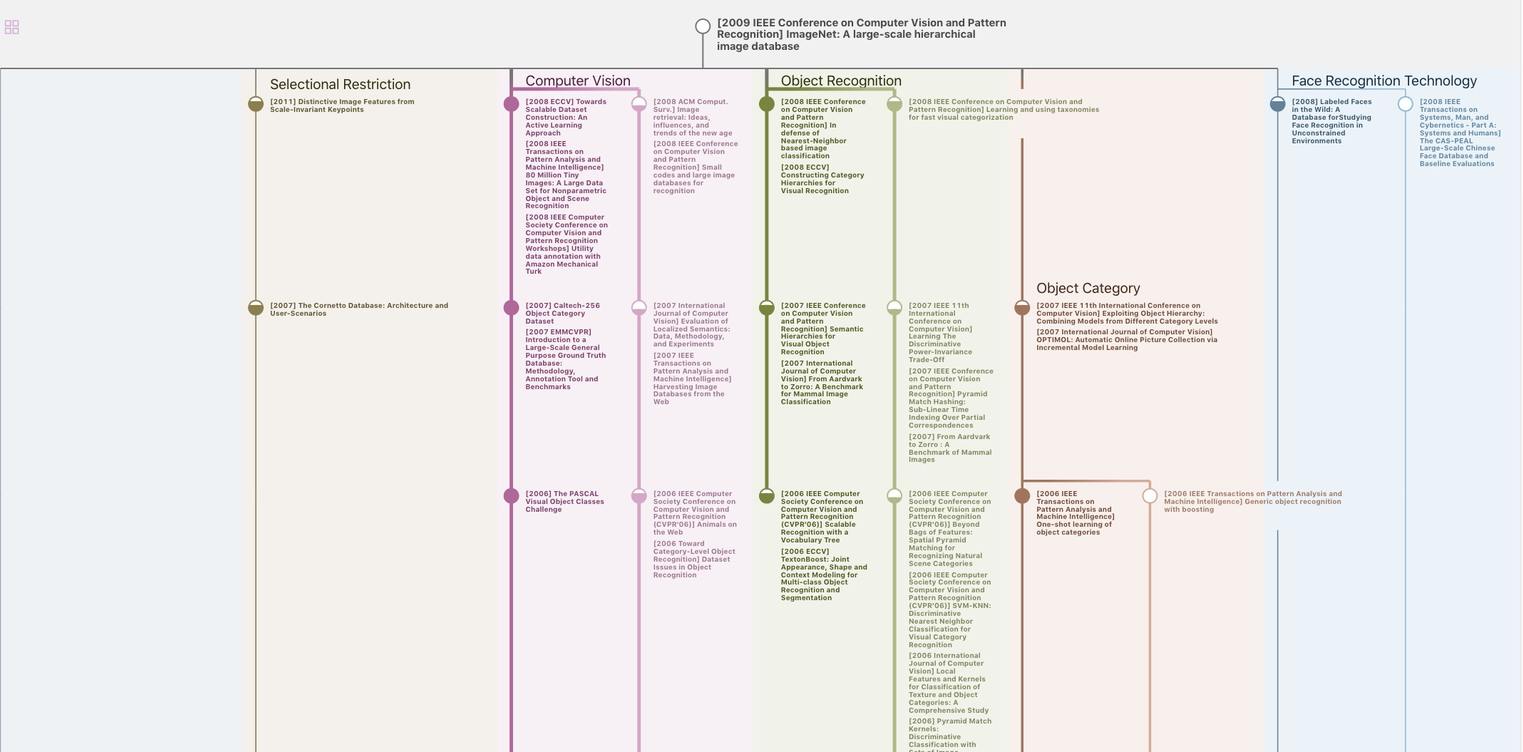
生成溯源树,研究论文发展脉络
Chat Paper
正在生成论文摘要