QRChEM: A Deep Learning Framework for Materials Property Prediction and Design Using QR Codes
ACS ENGINEERING AU(2023)
摘要
Machine learning (ML) surrogate models are used for the rapid prediction of materials properties and are promising tools for accelerating new materials design and development. The performance and accuracy of these surrogate models appear to be intricately connected to the molecular representation that is employed. Developing efficient numerical representations of molecules is vital for the success of surrogate models in predicting materials' properties. Here, we propose a new machine-readable molecular representation, namely a molecular quick response (QR) code, for the deep learning of materials structure-property correlations. We built a convolutional deep neural network (CNN) model based on molecular QR codes, which is abbreviated as QRChEM. QRChEM was trained and validated using similar to 21 000 data for four representative properties of small molecules, namely specific heat, enthalpy, zero-point vibrational energy, and HOMO-LUMO band gap. We show that QRChEM outperforms the commonly used Morgan fingerprint-based and one-hot encoding (OHE)-based deep learning frameworks. We further performed UMAP (uniform manifold approximation and projection) on the molecular QR codes to demonstrate the differentiability of the molecular topologies, which is vital for high-fidelity surrogate model development.
更多查看译文
关键词
Cheminformatics,Materials Design,QR Code,Deep Learning,Morgan Fingerprint,One-HotEncoding,Convolutional Neural Network
AI 理解论文
溯源树
样例
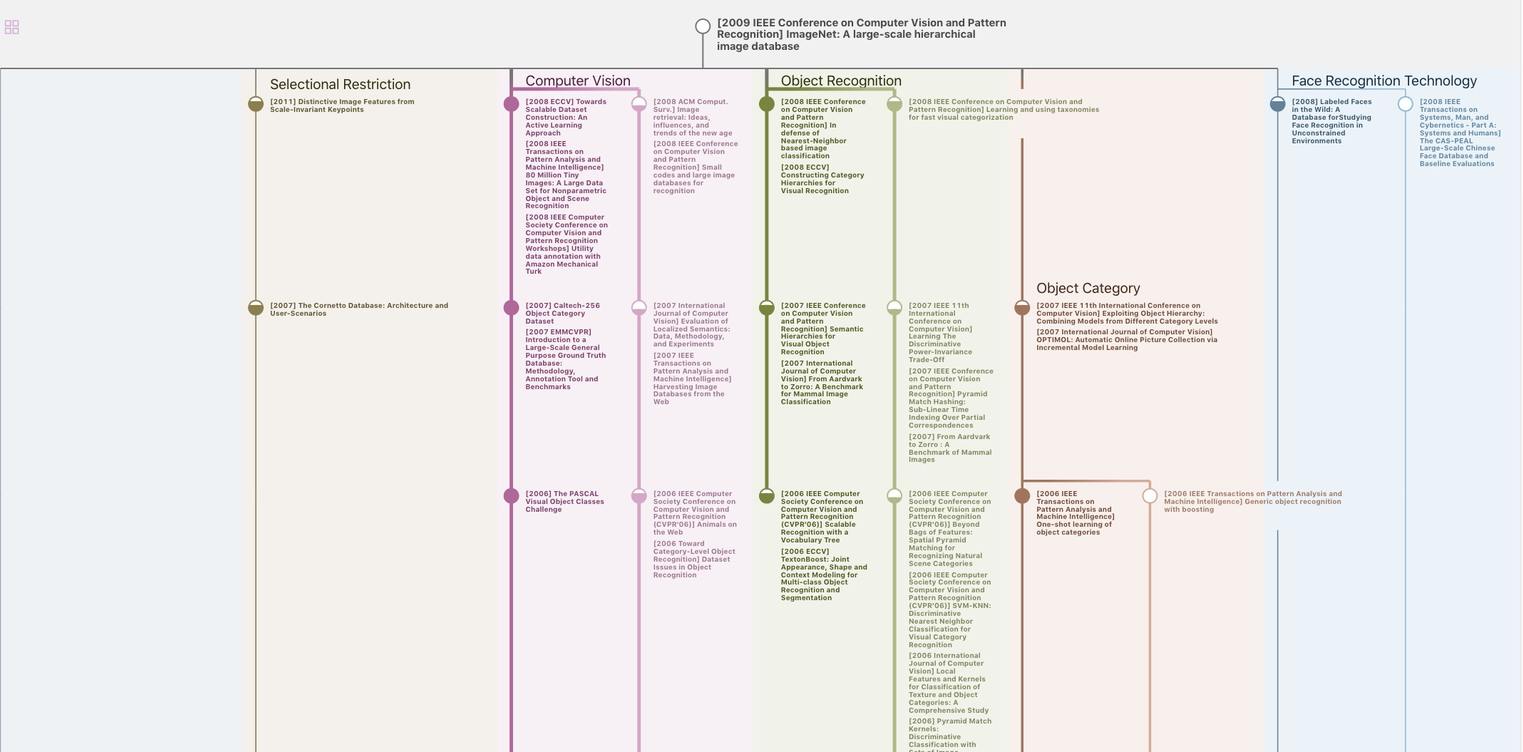
生成溯源树,研究论文发展脉络
Chat Paper
正在生成论文摘要