Study of dark photons using future electron–positron colliders based on machine learning
Journal of the Korean Physical Society(2024)
摘要
dark photon is a hypothetical particle that has a similar role to a photon in the Standard Model (SM) electromagnetic interaction but has been proposed as a force carrier with a potential link to dark matter. Detecting dark photon in particle collider experiments poses significant challenges owing to the interference from the SM events. This paper presents how effectively machine learning can reduce background events that pose a major problem in search for dark photons at future electron–positron accelerators/detectors: CEPC/CEPC, FCC-ee/IDEA and ILC/ILD. Based on the simplified model, we focus on the signal modes of e^+e^-→ A^'A^' and e^+e^-→A^'A^'γ , where A^' is a dark photon decaying into a muon pair. The dominant background originates from the SM. The background mode for the e^+e^-→ A^'A^' is e^+e^-→μ^+μ^-μ^+μ^- , and for the e^+e^-→A^'A^'γ , it is e^+e^-→μ^+μ^-μ^+μ^-γ . The signal events are generated using MadGraph5 with the simplified model, while the background events are generated with the SM. We simulated detector responses using Delphes. Machine learning classification was performed using a Boosted Decision Trees classifier built on the Toolkit for Multivariate Data Analysis. Our study demonstrates a significant improvement in the signal-to-background ratio by an order of 100–10000 by effectively reducing background events using machine learning. Finally, we show the detector efficiencies from well-separated signal events.
更多查看译文
关键词
Dark matter,Dark photon,Accelerator,Electron–positron collider,Machine learning
AI 理解论文
溯源树
样例
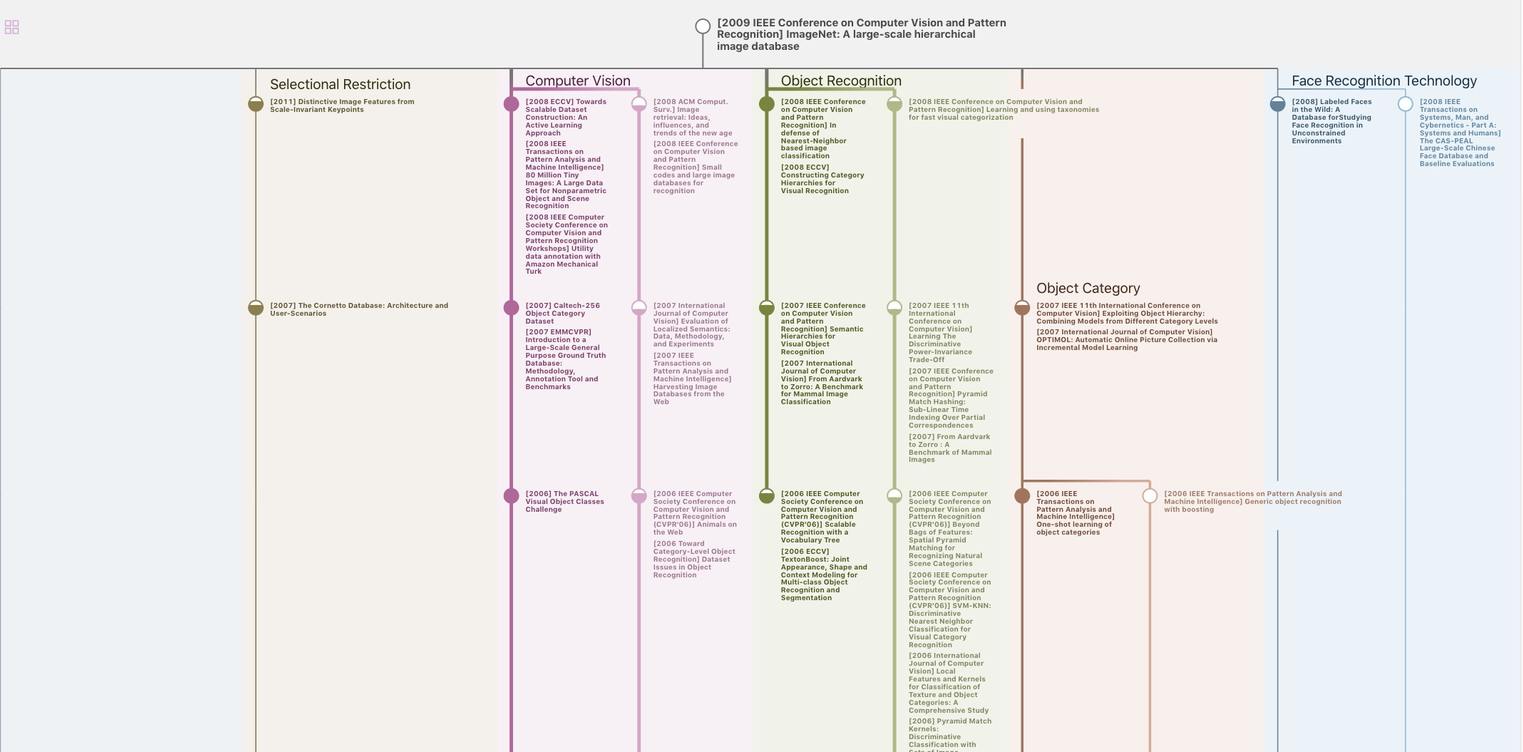
生成溯源树,研究论文发展脉络
Chat Paper
正在生成论文摘要