Practical Kernel Tests of Conditional Independence
CoRR(2024)
Abstract
We describe a data-efficient, kernel-based approach to statistical testing of
conditional independence. A major challenge of conditional independence
testing, absent in tests of unconditional independence, is to obtain the
correct test level (the specified upper bound on the rate of false positives),
while still attaining competitive test power. Excess false positives arise due
to bias in the test statistic, which is obtained using nonparametric kernel
ridge regression. We propose three methods for bias control to correct the test
level, based on data splitting, auxiliary data, and (where possible) simpler
function classes. We show these combined strategies are effective both for
synthetic and real-world data.
MoreTranslated text
AI Read Science
Must-Reading Tree
Example
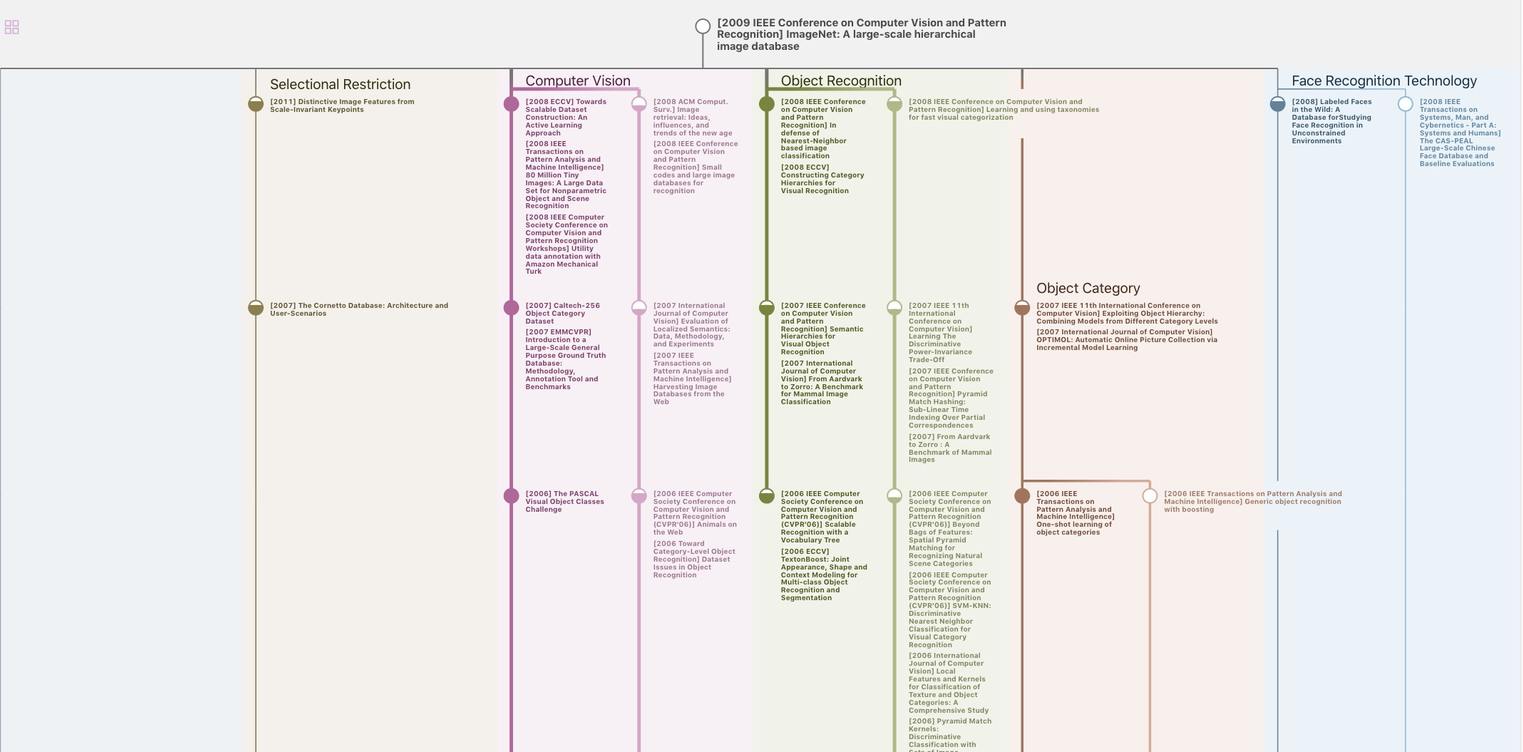
Generate MRT to find the research sequence of this paper
Chat Paper
Summary is being generated by the instructions you defined