Efficient Parameter Mining and Freezing for Continual Object Detection
CoRR(2024)
摘要
Continual Object Detection is essential for enabling intelligent agents to
interact proactively with humans in real-world settings. While
parameter-isolation strategies have been extensively explored in the context of
continual learning for classification, they have yet to be fully harnessed for
incremental object detection scenarios. Drawing inspiration from prior research
that focused on mining individual neuron responses and integrating insights
from recent developments in neural pruning, we proposed efficient ways to
identify which layers are the most important for a network to maintain the
performance of a detector across sequential updates. The presented findings
highlight the substantial advantages of layer-level parameter isolation in
facilitating incremental learning within object detection models, offering
promising avenues for future research and application in real-world scenarios.
更多查看译文
AI 理解论文
溯源树
样例
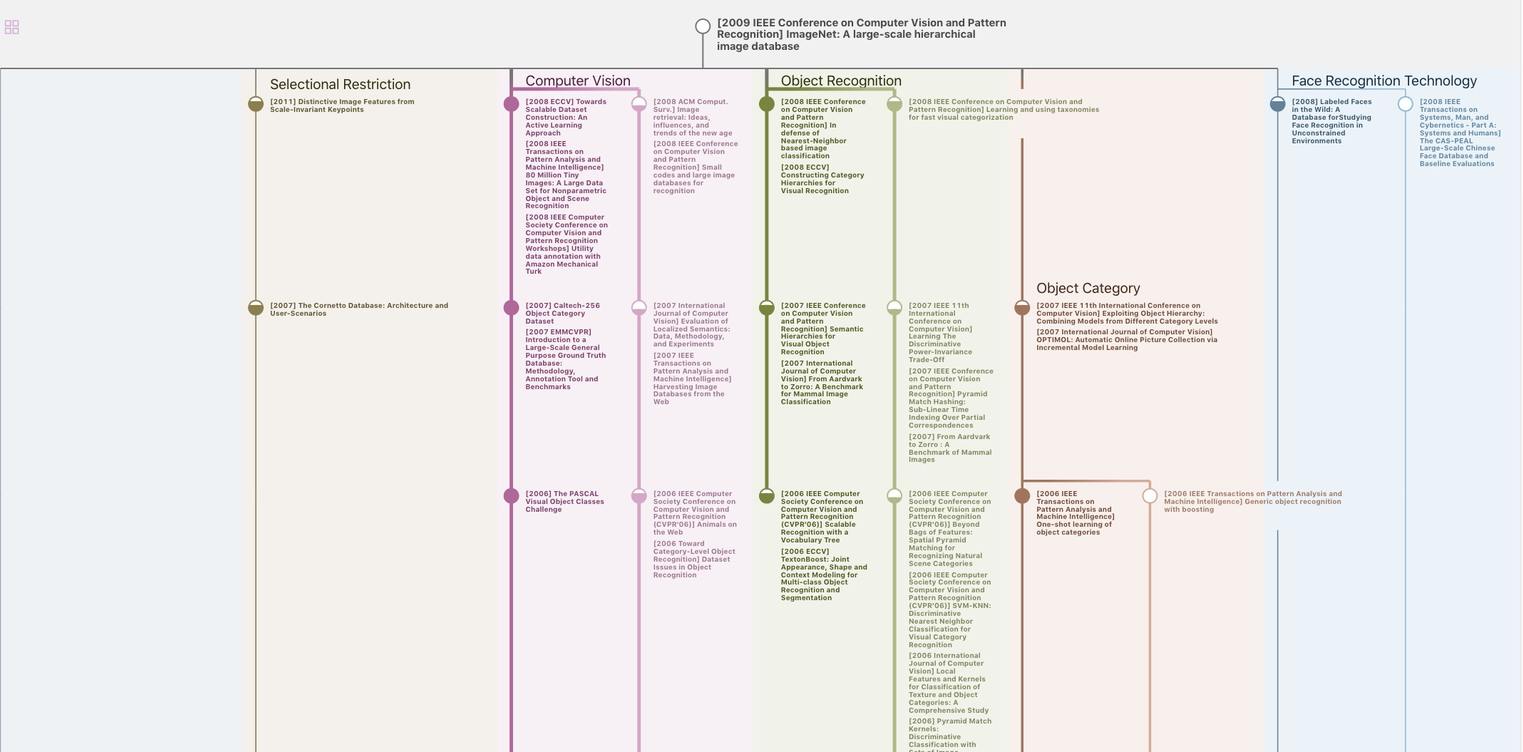
生成溯源树,研究论文发展脉络
Chat Paper
正在生成论文摘要