Multi-feature-fused generative neural network with Gaussian mixture for multi-step probabilistic wind speed prediction
APPLIED ENERGY(2024)
摘要
Wind speed prediction is a crucial element of effective wind energy utilization, necessitating the use of probabilistic wind speed prediction to facilitate practical decision-making. However, most current deep learning generative models neglect to incorporate frequency information. To address this issue, this study proposes a generative model neural network that utilizes multi-feature fusion and Gaussian mixture techniques. The proposed model leverages wavelet transformation, which is represented as a weight matrix, in combination with several convolution operations to extensively explore the fundamental features of wind speed time series. Prior to multi-step prediction, target values are modeled as a multi-dimensional Gaussian mixture distribution, with the extracted characteristics mapped onto crucial distribution parameters. Monte Carlo sampling is then used to complete the wind speed probability prediction. Furthermore, the study develops a loss function and a two-stage training strategy for this network. The performance of the proposed model is compared to that of ten state-of-theart models using actual wind speed time series and six typical error indices. The results demonstrate that the proposed model provides both more accurate deterministic predictions and more meaningful probabilistic forecasts than other examined models for various prediction.
更多查看译文
关键词
Wind speed probabilistic prediction,Generative model,Wavelet transformation,Convolution operation,Gaussian mixture
AI 理解论文
溯源树
样例
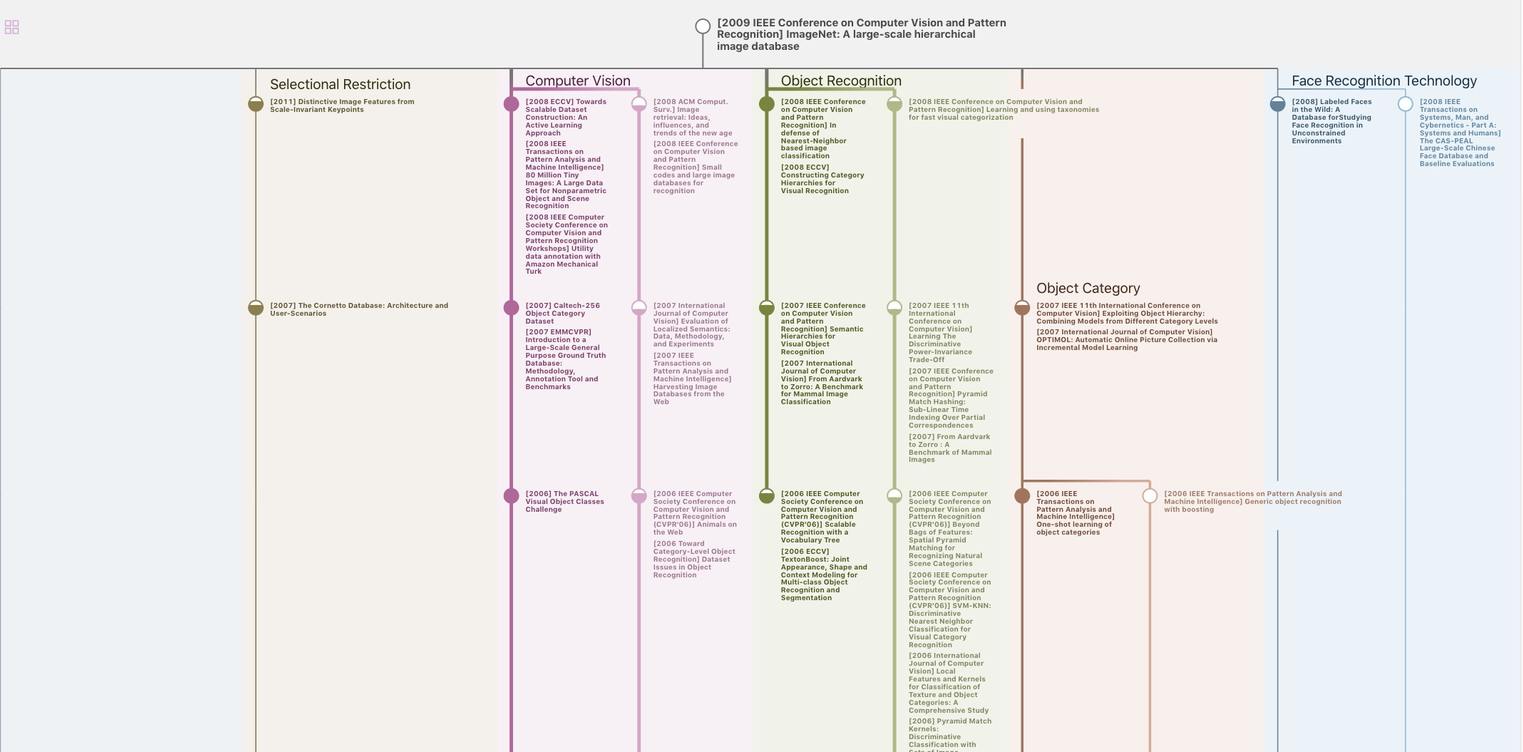
生成溯源树,研究论文发展脉络
Chat Paper
正在生成论文摘要