X-rays radiomics-based machine learning classification of atypical cartilaginous tumour and high-grade chondrosarcoma of long bones
EBIOMEDICINE(2024)
摘要
Background Atypical cartilaginous tumour (ACT) and high-grade chondrosarcoma (CS) of long bones are respectively managed with active surveillance or curettage and wide resection. Our aim was to determine diagnostic performance of X-rays radiomics-based machine learning for classification of ACT and high-grade CS of long bones. Methods This retrospective, IRB-approved study included 150 patients with surgically treated and histology -proven lesions at two tertiary bone sarcoma centres. At centre 1, the dataset was split into training (n = 71 ACT, n = 24 high-grade CS) and internal test (n = 19 ACT, n = 6 high-grade CS) cohorts, respectively, based on the date of surgery. At centre 2, the dataset constituted the external test cohort (n = 12 ACT, n = 18 high-grade CS). Manual segmentation was performed on frontal view X-rays, using MRI or CT for preliminary identification of lesion margins. After image pre-processing, radiomic features were extracted. Dimensionality reduction included stability, coefficient of variation, and mutual information analyses. In the training cohort, after class balancing, a machine learning classifier (Support Vector Machine) was automatically tuned using nested 10 -fold crossvalidation. Then, it was tested on both the test cohorts and compared to two musculoskeletal radiologists' performance using McNemar's test. Findings Five radiomic features (3 morphology, 2 texture) passed dimensionality reduction. After tuning on the training cohort (AUC = 0.75), the classifier had 80%, 83%, 79% and 80%, 89%, 67% accuracy, sensitivity, and specificity in the internal (temporally independent) and external (geographically independent) test cohorts, respectively, with no difference compared to the radiologists (p >= 0.617). Interpretation X-rays radiomics-based machine learning accurately differentiates between ACT and high-grade CS of long bones. Funding AIRC Investigator Grant. Copyright (c) 2024 The Author(s). Published by Elsevier B.V. This is an open access article under the CC BY -NC -ND license (http://creativecommons.org/licenses/by-nc-nd/4.0/).
更多查看译文
关键词
Arti fi cial intelligence,Atypical cartilaginous tumour,Bone neoplasm,Chondrosarcoma,Radiomics
AI 理解论文
溯源树
样例
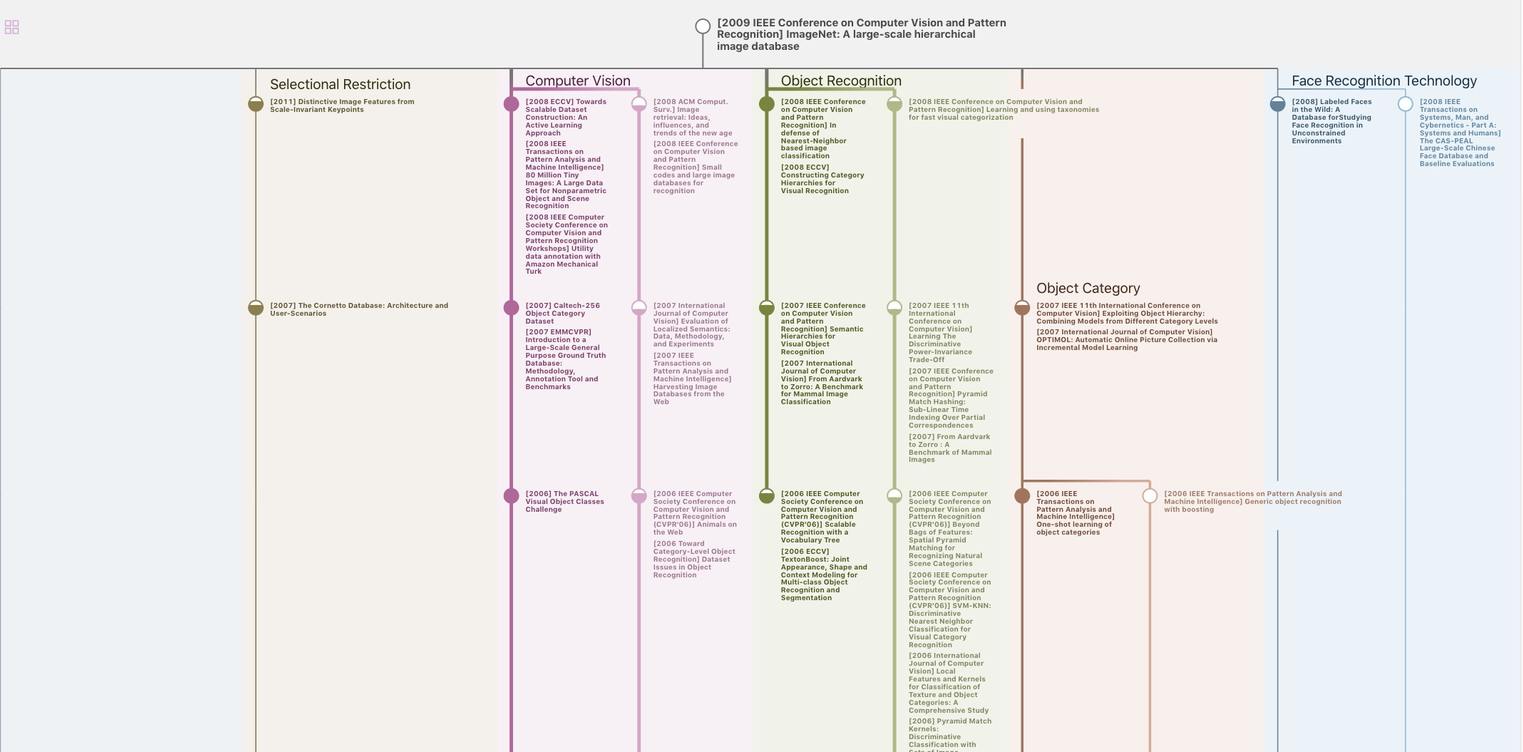
生成溯源树,研究论文发展脉络
Chat Paper
正在生成论文摘要