Multi-span transition networks: a new unified framework for analyzing time series
Nonlinear Dynamics(2024)
Abstract
The paper seeks to overcome the limitations inherent in traditional transition network methods, which primarily concentrate on transition frequencies between adjacent symbols, neglecting broader transition relationships. We present a novel approach called “multi-span transition network.” This method excels at capturing dynamic information within time series by incorporating transitions across higher time-scale patterns. We also propose a conditional entropy measure to assess the complexity of time-series data derived from the multi-span transition network. With expanding dimensionality, the multi-span transition network adeptly discriminates between various types of time series and unveils concealed information. The conditional entropy of the multi-span transition network exhibits a robust correlation with the maximum Lyapunov exponent of the system. The conditional entropy of a multi-span network can distinguish the time series of different states and determine chaos degradation. Employing the multi-span transition network for the classification of epileptic EEG data resulted in a substantial enhancement in accuracy compared to conventional transition network methods. The method is a more general form of the traditional transition network and is more generalizable.
MoreTranslated text
Key words
Ordinal pattern method,Multi-span transition networks,Complexity of time series,Chaotic transitions
AI Read Science
Must-Reading Tree
Example
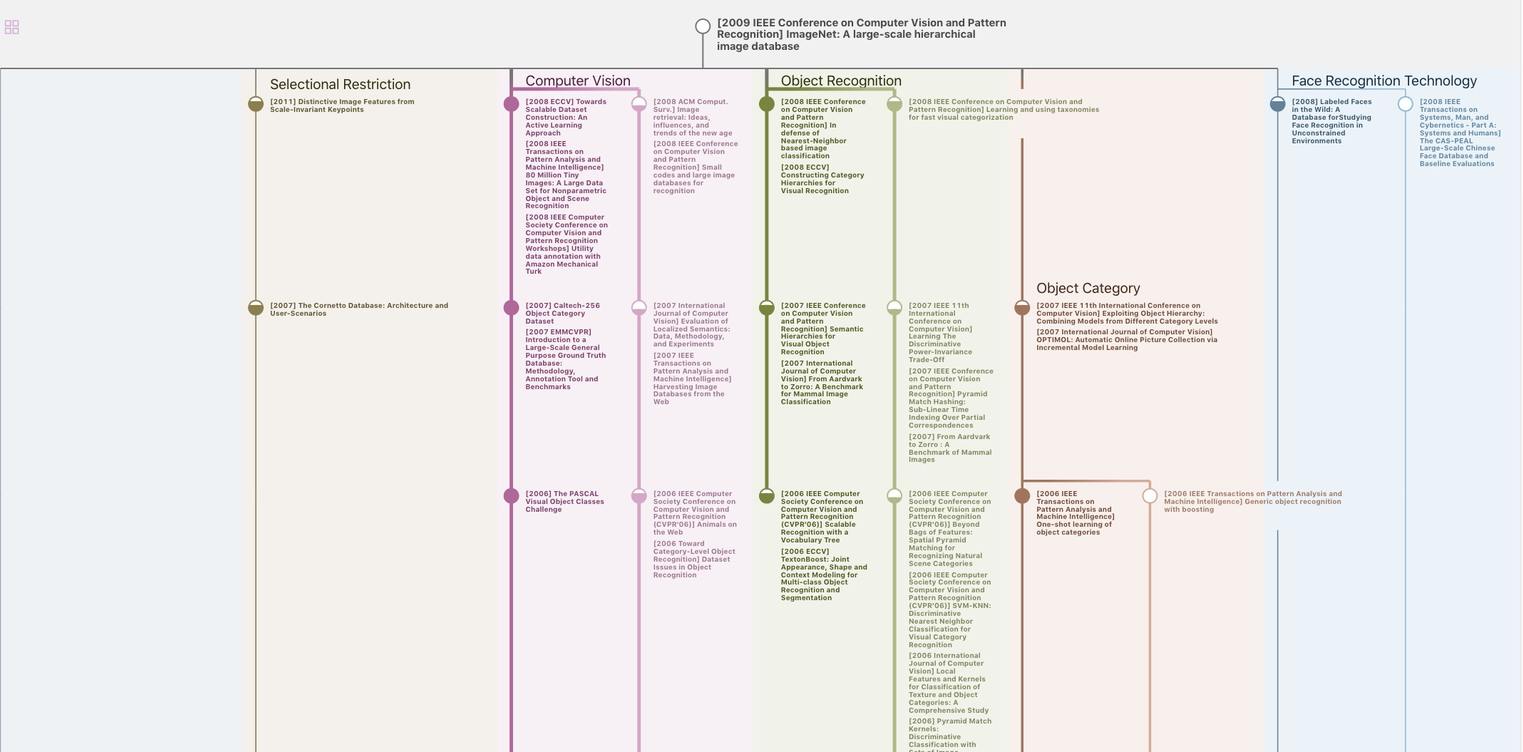
Generate MRT to find the research sequence of this paper
Chat Paper
Summary is being generated by the instructions you defined