Toward enhancing concrete crack segmentation accuracy under complex scenarios: a novel modified U-Net network
Multimedia Tools and Applications(2024)
Abstract
Convolutional neural networks (CNNs) have demonstrated promising accuracy in segmenting concrete cracks under controlled conditions. However, these existing methods usually are challenging in addressing multi-scale patterns or complex scenarios. To address this pervasive issue, a modified U-Net network was established in the current study. The proposed model, namely U-Net-ASPP-CBAM, integrates the convolutional block attention module after a convolution operator to selectively focus on the crack information while disregarding irrelevant background details during feature extraction. Furthermore, U-Net-ASPP-CBAM network replaces a pooling layer using the atrous spatial pyramid pooling module to explore and fuse features across multiple scales, enhancing information capture for the segmentation of small objects. The performance of the proposed model has been validated by a self-built dataset comprising crack images with diverse complex backgrounds. And the segmentation effectiveness is assessed through evaluation indices, including precision, recall, F1 score, pixel accuracy, and mean intersection over union. The results show the proposed U-Net-ASPP-CBAM model outperforms other segmentation models.
MoreTranslated text
Key words
Pixel-level crack segmentation,Deep learning,Improved U-Net model,Attention module,Atrous spatial pyramid pooling module
AI Read Science
Must-Reading Tree
Example
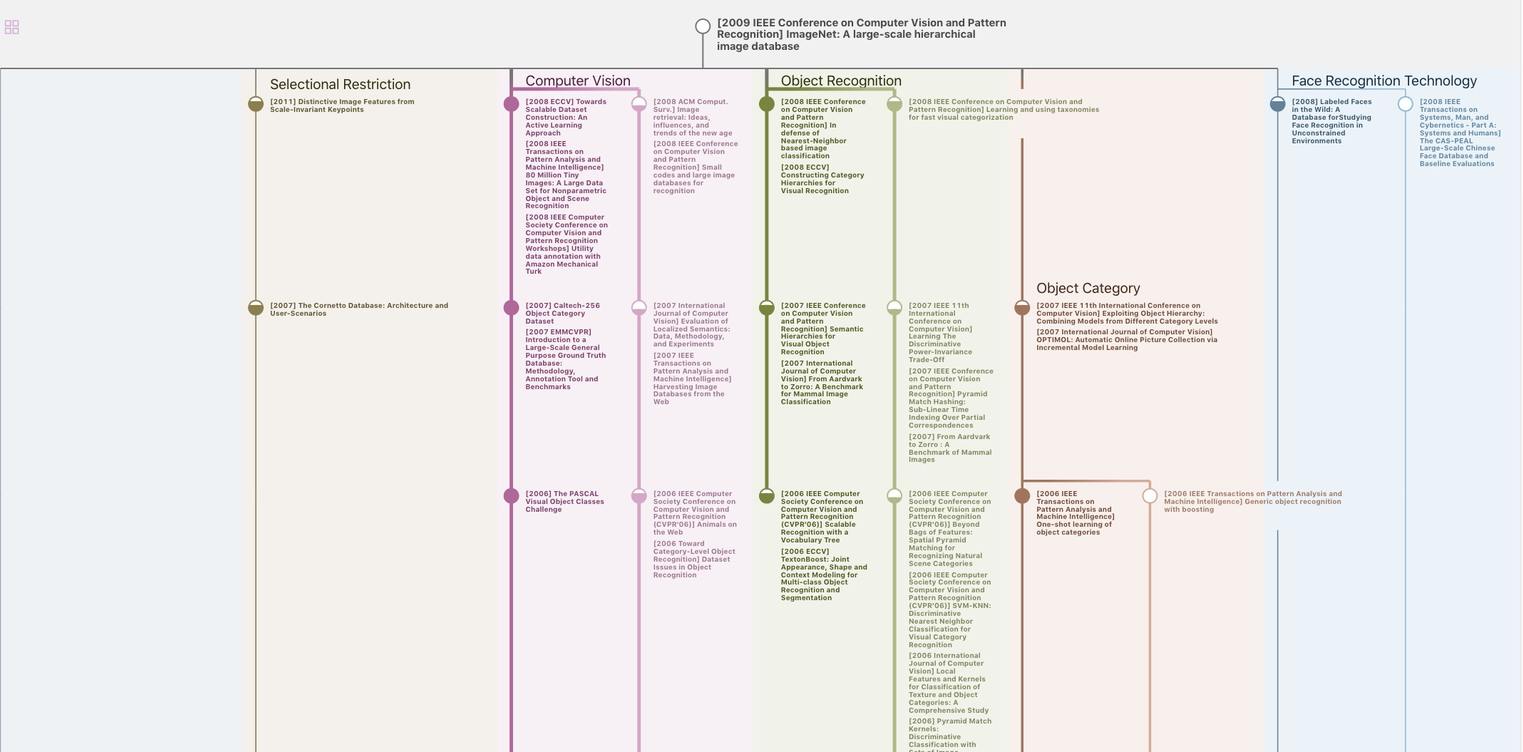
Generate MRT to find the research sequence of this paper
Chat Paper
Summary is being generated by the instructions you defined