Multi-scale Attention Conditional GAN for Underwater Image Enhancement
ADVANCES IN COMPUTER GRAPHICS, CGI 2023, PT I(2024)
摘要
Underwater image enhancement (UIE) has achieved impressive achievements in various marine tasks, such as aquaculture and biological monitoring. However, complex underwater scenarios impede current UIE method application development. Some UIEmethods utilize convolutional neural network (CNN) based models to improve the quality of degradation images, but these methods fail to capture multi-scale high-level features, leading to sub-optimal results. To address these issues, we propose a multi-scale attention conditional generative adversarial network (GAN), dubbed Mac-GAN, to recover the degraded underwater images by utilizing an encoder-decoder structure. Concretely, a novel multi-scale conditional GAN architecture is utilized to aggregate the multi-scale features and reconstruct the high-quality underwater images with high perceptual information. Different from the reference model, a novel attention module (AMU) is designed to integrate associated features among the channels for the UIE tasks and embedded after the down sampling layer, effectively suppressing non-significant features to improve the extraction effect of multi-scale features. Meanwhile, perceptual loss and total variation loss are utilized to enhance smoothness and suppress artifacts. Extensive experiments demonstrate that our proposed model achieves remarkable results in terms of qualitative and quantitative metrics, such as 0.7dB improvement in PSNR metrics and 0.8dB improvement in UIQM metrics. Moreover, Mac-GAN can generate a pleasing visual result without obvious over-enhancement and over-saturation over the comparison of UIE methods. A detailed set of ablation experiments analyzes core components' contribution to the proposed approach.
更多查看译文
关键词
Conditional Generative Adversarial Network,Underwater Image Enhancement,Attention Module
AI 理解论文
溯源树
样例
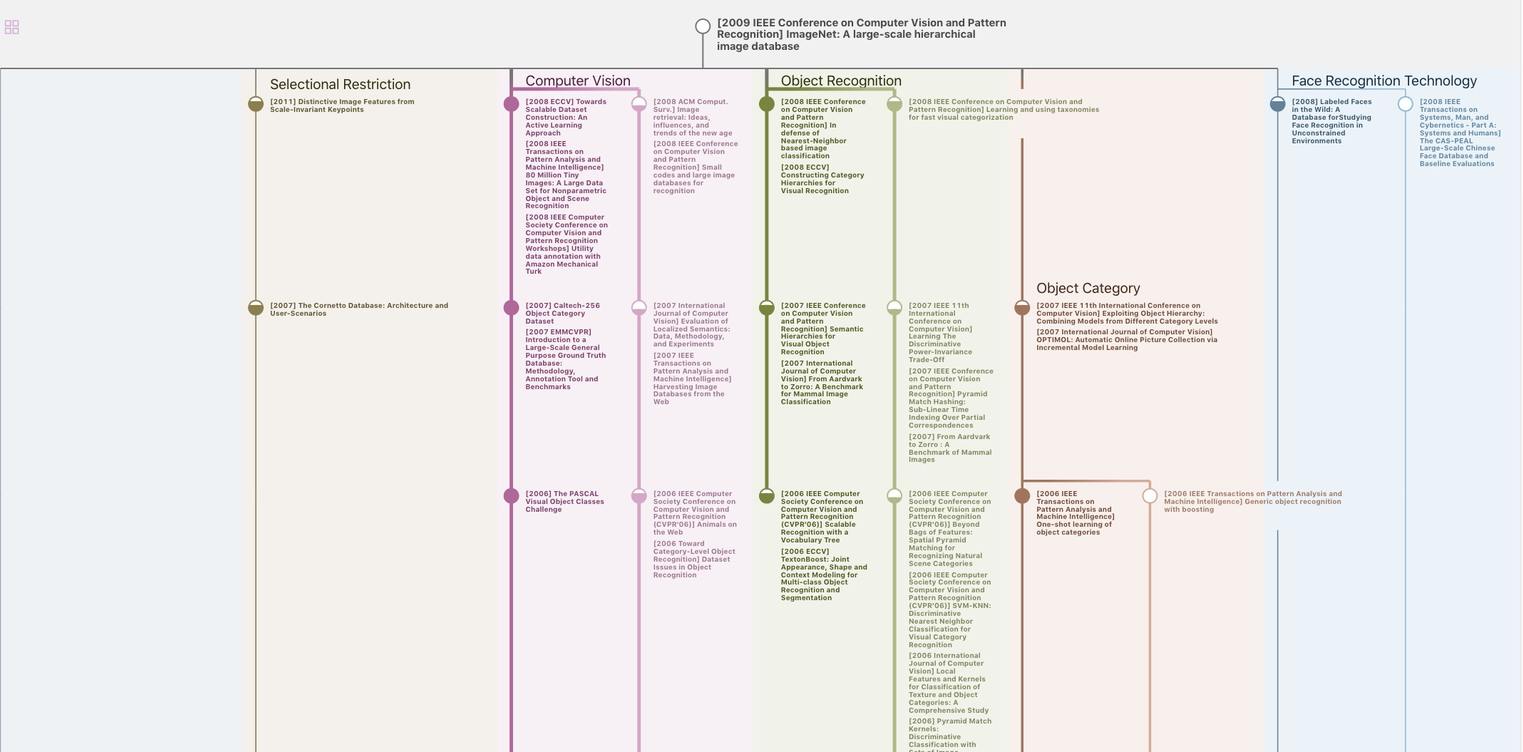
生成溯源树,研究论文发展脉络
Chat Paper
正在生成论文摘要