Is ImageNet Always the Best Option? An Overview on Transfer Learning Strategies for Document Layout Analysis
IMAGE ANALYSIS AND PROCESSING - ICIAP 2023 WORKSHOPS, PT II(2024)
摘要
Semantic segmentation models have shown impressive performance in the context of historical document layout analysis, but their effectiveness is reliant on having access to a large number of high-quality annotated images for training. A popular approach to address the lack of training data in other domains is to rely on transfer learning to transfer the knowledge learned from a large-scale, general-purpose dataset (e.g. ImageNet) to a domain-specific task. However, this approach has been shown to lead to unsatisfactory results when the target task is completely unrelated to the data employed for the pre-training process, which is the case when working on document layout analysis. For this reason, in the present paper, we provide an overview of domain-specific transfer learning for document layout segmentation. In particular, we show how relying on document-related images for the pre-training process leads to consistently improved performance and faster convergence compared to training from scratch or even relying on a large, general purpose, dataset such as ImageNet.
更多查看译文
关键词
Document Layout Analysis,Fine Tuning Approach,Page Segmentation
AI 理解论文
溯源树
样例
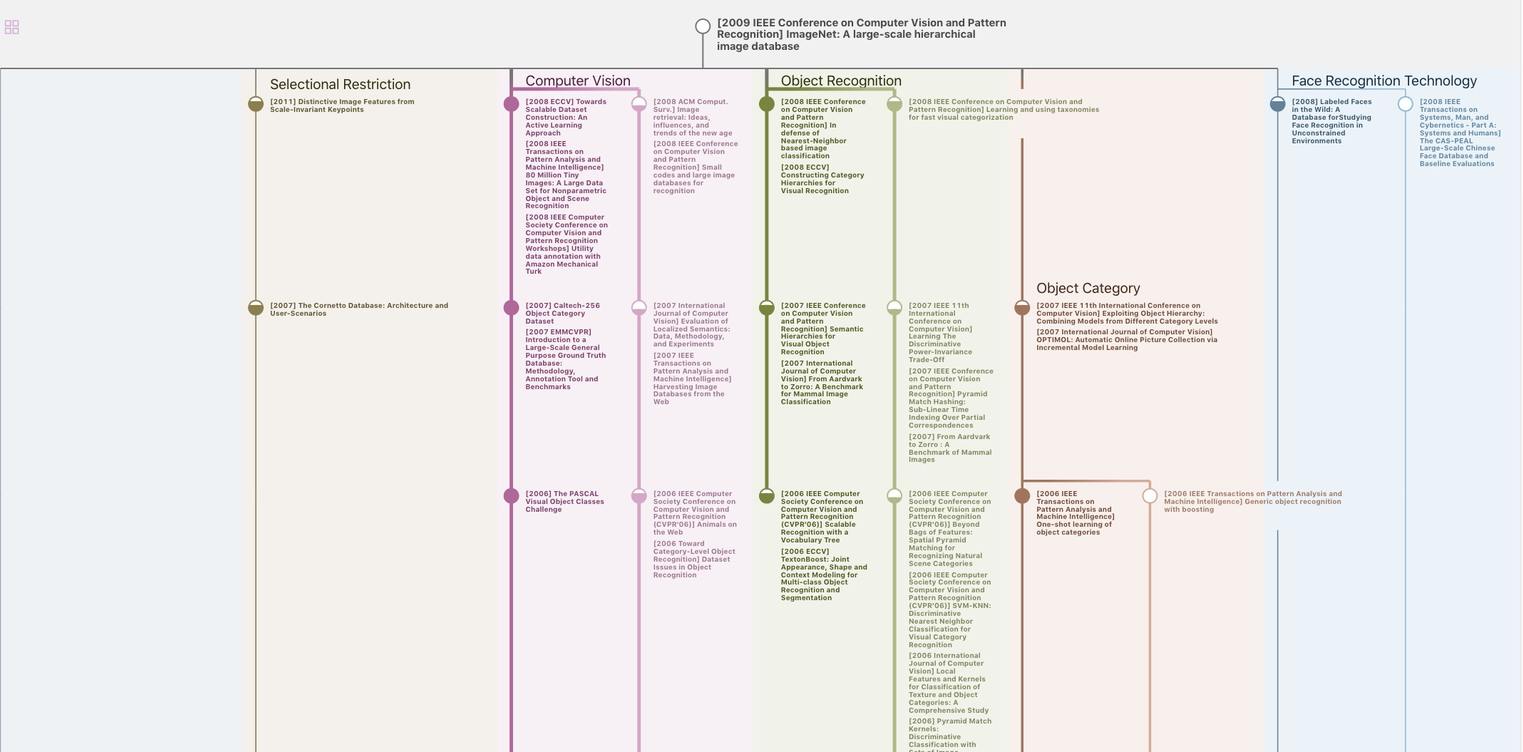
生成溯源树,研究论文发展脉络
Chat Paper
正在生成论文摘要