A hybrid-Lp- Norms-Oriented Robot Kinematic Calibration Model Optimized by a Novel Newton Interpolated Differential Evolution Algorithm
2023 23RD IEEE INTERNATIONAL CONFERENCE ON DATA MINING WORKSHOPS, ICDMW 2023(2023)
摘要
Kinematic calibration can obtain real kinematic parameters by the establishment and the solution of the kinematic error models, achieving high-precision control of an industrial robot. However, the data collection points frequently fail to cover the entire robot workspace in data collection due to human error, thereby yielding incomplete robot kinematic calibration (RKC) data. Moreover, existing RKC models all adopt a single distance-oriented Loss scheme, resulting in the ignoration of the RKC data's characteristics demonstrated by other metrics. Motivated by these discoveries, this work first fills in the RKC data as a preprocessing step for robot calibration. Then, we propose a hybrid-L-p-norms-oriented RKC (HLRKC) model to address the RKC data's characteristics issue. It adopts twofold ideas: a) aggregating multiple L-p norm effects to form a hybrid Loss; b) adaptively adjusting the weights of all L-p norms in the hybrid Loss. Subsequently, based on the HLRKC model, we present a new Newton Interpolated Differential Evolution (NIDE) algorithm to form a NIDE-HLRKC calibrator. Experimental results demonstrate that the HLRKC-NIDE calibrator substantially exceeds the state-of-the-art calibrators in calibration accuracy and convergence rate. Hence, it strongly supports its excellent potential in robot calibration issues.
更多查看译文
关键词
Industrial Robot,robot kinematic calibration (RKC),incomplete RKC data,L-p norm,kinematic parameters,Newton interpolated differential evolution (NIDE),calibrator.
AI 理解论文
溯源树
样例
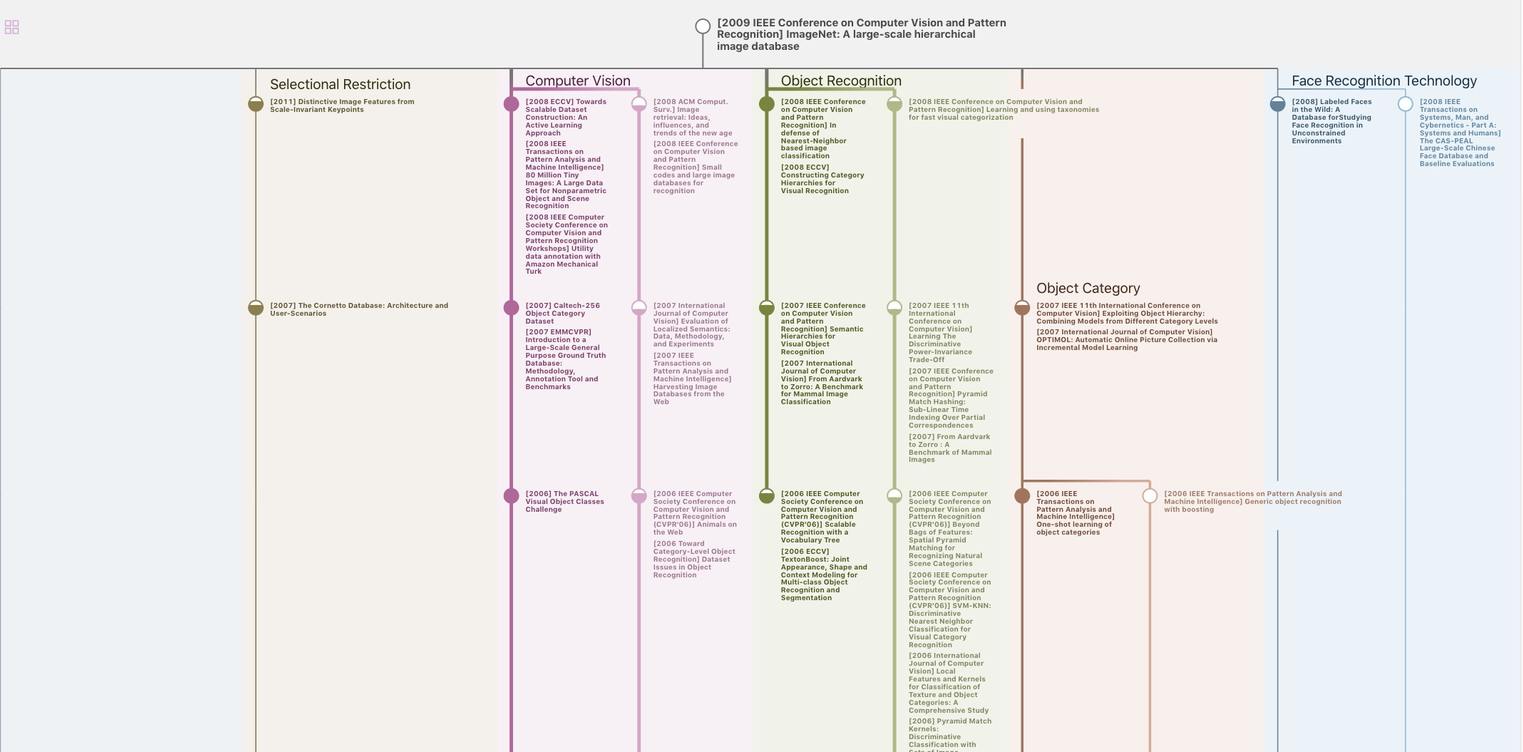
生成溯源树,研究论文发展脉络
Chat Paper
正在生成论文摘要