Asymptotic Gaussian Fluctuations of Eigenvectors in Spectral Clustering
CoRR(2024)
摘要
The performance of spectral clustering relies on the fluctuations of the
entries of the eigenvectors of a similarity matrix, which has been left
uncharacterized until now. In this letter, it is shown that the signal +
noise structure of a general spike random matrix model is transferred to the
eigenvectors of the corresponding Gram kernel matrix and the fluctuations of
their entries are Gaussian in the large-dimensional regime. This CLT-like
result was the last missing piece to precisely predict the classification
performance of spectral clustering. The proposed proof is very general and
relies solely on the rotational invariance of the noise. Numerical experiments
on synthetic and real data illustrate the universality of this phenomenon.
更多查看译文
AI 理解论文
溯源树
样例
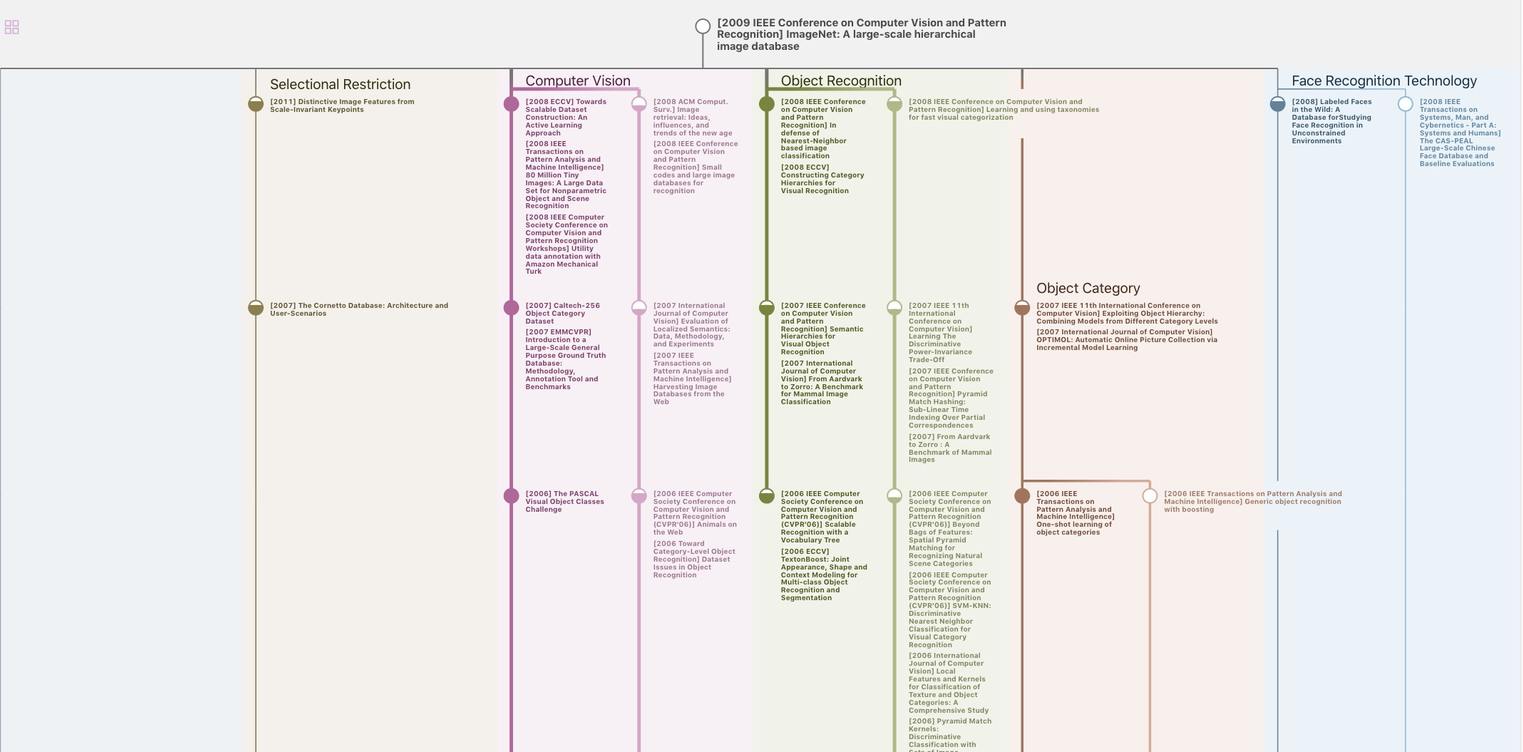
生成溯源树,研究论文发展脉络
Chat Paper
正在生成论文摘要